In today’s increasingly complex business environment, insurance companies are under tremendous pressure to deliver personalized, data-driven services. This is why a growing interest has been observed in integrating artificial intelligence into consulting workflows. Unfortunately, however, many AI applications used in this sector are incapable of matching the ethical considerations and nuanced reasoning that human experts bring to advisory roles.
Balaji Adusupalli, an expert in artificial intelligence, machine learning, and Gen AI-powered integrations, has proposed an approach combining artificial intelligence with human insight through a framework called Multi-Agent Advisory Networks (MAANs). His research titled “Multi-Agent Advisory Networks: Redefining Insurance Consulting with Collaborative Agentic AI Systems” focuses on building scalable, interpretable AI systems that support human consultants instead of replacing them. This study provides a collaborative path forward for decision-making in modern insurance advisory services.
Insurance Advisory in the Digital Age
The deep expertise of human consultants has always been integral to the insurance industry’s ability to recommend coverage, interpret risk, and navigate compliance frameworks. However, the limitations of purely human-driven advisory systems have become more prominent as organizations contend with highly specific regulatory environments, rising customer expectations, and vast volumes of real-time data. Traditional models are ill-equipped to deliver faster, more accurate and personalized consulting services at scale.
The problem is compounded by the growing diversity of client needs and complexity of insurance products. Human advisors often find it difficult to keep pace with continuous regulatory changes or synthesize data manually from claims, behavior, actuarial models, and external risk sources. Moreover, advisory decisions often involve a high degree of variability across clients and uncertainty, which makes it extremely challenging to achieve speed and consistency.
According to Adusupalli, in this emerging landscape, AI should be a partner to human consultants, not a competitor. However, AI adoption has been retarded significantly by concerns over its rigidity, opacity, and lack of contextual reasoning. Through his research, Adusupalli proposes a collaborative framework that preserves the nuanced decision-making capabilities of human experts while leveraging the computational strength of AI. Acknowledging that machines excel at recognizing patterns and processing large datasets, this hybrid approach also considers human advisors to be indispensable in interpreting insights, managing relationships, and navigating ethical concerns.
Importance of Multi-Agent Advisory Networks (MAAN)
The concept of Multi-Agent Advisory Networks (MAAN) forms the core of Adusupalli’s research. These systems use modular and specialized AI agents to imitate the collaborative nature of human consulting teams. Instead of relying on monolithic AI models, these systems distribute functionality across a network of agents. Each of these agents is assigned a unique role within the advisory process. Operating semi-independently, these agents share insights and refine recommendations through a process of continual learning and supervised collaboration.
Based on splitting neural networks, this architecture divides complex tasks across agents to reduce computational and memory overhead, which ensures performance even in low-resource environments. MAAN leverages supervised learning for easier implementation, greater stability, and faster convergence in real-world enterprise settings.
It is possible to specialize each agent within MAAN in a narrow domain, such as regulatory alignment, underwriting decision support, claims forecasting, or risk modeling. These agents deliver insights that are both comprehensive and contextually grounded by simulating the dynamics of a high-performing advisory team. Also, owing to independently interpretable logic and outputs of each agent, MAAN offers transparent decision-making pathways that support compliance, auditability, and user trust.
Bridging Fragmentation in Insurance Consulting
Critical advisory processes in many insurance organizations are siloed across functional units such as underwriters, claims adjusters, actuarial teams, brokers and legal advisors. As a result, these units often have limited visibility into each other’s decision logic. This fragmentation can cause inconsistencies, delays, and inefficiencies in client communications and policy recommendations.
MAAN addresses this problem strategically by establishing a coordinated agent ecosystem spanning across departmental boundaries. Ingesting data and context from a specific domain, each agent contributes its insights to a shared pool of knowledge. This shared intelligence ensures that all advisory outputs reflect a harmonized understanding of the organization’s priorities and the client’s situation.
MAAN also provides a common interface that can be used by human consultants to interact with the agents, review their findings, and apply professional judgment. This integration helps foster more informed and agile decision-making across the enterprise by facilitating collaboration between human advisors and digital assistants.
Conclusion
The research by Balaji Adusupalli provides a practical and compelling blueprint for the integration of artificial intelligence into complex advisory services. By designing AI systems that are transparent, collaborative, and adaptive, his work promotes responsible, human-centered innovation.
“The goal is not to replace consultants but to enhance the value they provide—through scalable intelligence, collaborative reasoning, and context-aware decision support,” Adusupalli concludes. “By combining agentic learning with domain-specific expertise, we can build systems that not only deliver recommendations but also adapt to the unique challenges of each client interaction.”
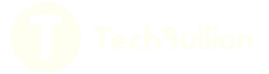