Data science is taking center stage. With the explosion of smart vehicles and connected car technologies, a vast amount of data is generated daily from millions of vehicles around the world. This data, if properly harnessed, has the potential to revolutionize the automotive sector by improving vehicle design, safety, efficiency, and even the driving experience. Automotive data science focuses on extracting valuable insights from this data, allowing car manufacturers, fleet operators, and even individual drivers to make informed decisions.
What is Automotive Data Science?
Automotive data science is the process of collecting, analyzing, and deriving meaningful insights from vehicle data. Vehicles today are equipped with numerous sensors, advanced telematics systems, and Internet of Things (IoT) devices that record a wide range of data points, from engine performance and fuel consumption to driver behavior and traffic patterns. This massive volume of data is then analyzed using data science techniques such as machine learning, statistical analysis, and predictive modeling.
By leveraging automotive data science, manufacturers and service providers can gain deeper insights into vehicle performance, detect potential issues before they become critical, and enhance the overall driving experience. Moreover, it plays a crucial role in the development of autonomous vehicles, electric cars, and predictive maintenance systems.
Key Components of Automotive Data Science
Automotive data science encompasses several crucial components that work together to extract actionable insights. These include:
Collection:
Data is gathered from various sources such as sensors, cameras, GPS systems, and onboard diagnostics (OBD) units.
Storage:
Massive amounts of vehicle data require scalable storage solutions such as cloud computing platforms.
Data Processing:
This involves cleaning, structuring, and organizing raw data for analysis.
Data Analysis:
Using data science tools like machine learning algorithms, big data analytics, and artificial intelligence (AI), meaningful insights are derived.
Data Visualization:
Presenting insights in a human-readable format such as charts, graphs, and dashboards helps users understand the results.
Key Applications of Automotive Data Science
Automotive data science has numerous real-world applications. Below are some of the most prominent ones:
Predictive Maintenance
Predictive maintenance is one of the most significant applications of automotive data science. By analyzing vehicle sensor data, engineers can detect patterns that may indicate an impending failure or mechanical issue. For example, anomalies in engine temperature, brake wear, or fuel efficiency can be early indicators of problems. By using predictive models, car manufacturers can notify drivers or fleet managers about necessary maintenance, preventing breakdowns and reducing costs.
Autonomous Driving
Data science plays a pivotal role in the development of autonomous vehicles. Self-driving cars rely heavily on data from sensors such as LiDAR, radar, and cameras to make real-time decisions. Automotive data science helps autonomous vehicles interpret their surroundings, detect obstacles, and make safe driving decisions. Machine learning algorithms analyze this data and improve the vehicle’s ability to navigate complex driving environments, such as urban streets or highways.
Moreover, automotive data science is critical in ensuring the safety of autonomous vehicles. By analyzing the data collected during test drives, developers can identify areas where autonomous systems need improvement.
Optimizing Fuel Efficiency
Another important application of automotive data science is improving vehicle fuel efficiency. By analyzing data such as driving speed, engine performance, and road conditions, machine learning algorithms can provide drivers with real-time feedback on how to optimize fuel consumption. This helps reduce overall fuel costs and supports environmental sustainability.
In fleet management, analyzing vehicle usage patterns and driver behavior can lead to insights that help optimize fuel consumption across large fleets of vehicles, saving companies millions of dollars annually.
Connected Vehicles and Telematics
Connected vehicles generate massive amounts of data that are used to improve driving experiences and services. Automotive data science enables the analysis of this data to enhance vehicle connectivity, enabling features such as real-time navigation, traffic updates, and even remote diagnostics. Telematics systems, which track a vehicle’s location, speed, and driving habits, also rely heavily on data science to provide insights into driver behavior and vehicle performance.
Telematics data can also be used by insurance companies to offer usage-based insurance (UBI). By analyzing driving behavior data, insurers can create personalized policies that reward safe driving habits with lower premiums.
Driver Behavior Analysis
Understanding how drivers behave behind the wheel is another key area where automotive data science is making a significant impact. By analyzing data from vehicle sensors, telematics systems, and even in-car cameras, data scientists can identify patterns in driver behavior. This can include detecting aggressive driving, fatigue, or distracted driving. With these insights, automakers can design better safety features, while fleet managers can offer training to improve driver performance and reduce accidents.
Enhancing In-Vehicle Experiences
Modern vehicles are not just about getting from point A to point B; they’re about offering a complete experience. Automotive data science is helping manufacturers understand consumer preferences and behaviors to enhance in-vehicle experiences. From personalized entertainment systems to voice-activated assistants, automakers are using data science to create more intuitive and enjoyable driving environments.
The Role of Machine Learning in Automotive Data Science
Machine learning is at the heart of automotive data science. It allows systems to learn from data without explicit programming and improve over time. In the automotive industry, machine learning is used to analyze massive datasets, identify patterns, and make predictions.
For instance, in predictive maintenance, machine learning algorithms can analyze historical data on vehicle performance and predict when a part is likely to fail. Similarly, in autonomous driving, machine learning is used to process sensor data and help the vehicle make real-time decisions.
Deep learning, a subset of machine learning, is also used to power image recognition systems in autonomous vehicles. By training deep learning models on vast amounts of image data, cars can “see” and recognize objects such as pedestrians, road signs, and other vehicles.
Challenges in Automotive Data Science
While the potential of automotive data science is immense, there are several challenges that the industry must overcome.
Data Security and Privacy
One of the biggest challenges in automotive data science is ensuring data security and privacy. Vehicles collect a vast amount of sensitive data, including location, speed, and driving behavior. This data must be protected from cyberattacks and unauthorized access. Data privacy regulations, such as the General Data Protection Regulation (GDPR), also place stringent requirements on how this data is handled.
Data Integration
Automotive data comes from a variety of sources, such as different sensors, telematics systems, and third-party applications. Integrating this data into a single, cohesive dataset can be challenging. Data scientists need to ensure that the data is accurate, consistent, and clean before it can be used for analysis.
Scalability
As the number of connected vehicles grows, so does the volume of data generated. This presents challenges in terms of data storage, processing, and analysis. Automotive companies must invest in scalable cloud-based platforms that can handle the ever-increasing data load.
Complexity of Algorithms
Machine learning and AI algorithms used in automotive data science are complex and require significant computational resources. Developing these algorithms and ensuring their accuracy requires expertise and significant time investment.
The Future of Automotive Data Science
The future of automotive data science looks incredibly promising. As vehicles become more connected, autonomous, and electric, the amount of data generated will continue to grow exponentially. With advancements in machine learning, AI, and cloud computing, we can expect even more sophisticated applications of data science in the automotive industry.
Autonomous Vehicles:
Fully autonomous vehicles will rely heavily on data science to operate safely and efficiently.
Electric Vehicles (EVs):
Data science will play a crucial role in optimizing battery performance and energy efficiency in electric vehicles.
Smart Cities:
As cities become smarter, automotive data will be integrated with urban infrastructure to improve traffic management and reduce congestion.
Conclusion
Automotive data science is revolutionizing the way we think about transportation. By extracting meaningful insights from vehicle data, we can improve vehicle performance, enhance safety, and create more enjoyable driving experiences. The future is bright for this exciting field, and its potential is limitless.
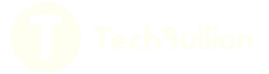