AI-powered Root Cause Analysis (RCA) is transforming the way modern systems identify and resolve underlying issues. Nikhil BharadwajRamashastri, a pioneer in this field, explores the technology that is enhancing operational efficiency, reducing costs, and increasing system reliability across industries. Through the integration of machine learning, anomaly detection, and natural language processing (NLP), AI is driving a new era of problem-solving that surpasses traditional manual methods in both speed and accuracy.
Traditional vs. AI-Powered Root Cause Analysis
Traditional RCA is labor-intensive, prone to errors, and inefficient for complex systems, requiring expert teams to analyze logs and data manually. AI-powered RCA, in contrast, leverages machine learning algorithms to process large datasets quickly and accurately, identifying root causes in minutes instead of hours.
AI-powered RCA can eliminate many of the shortcomings of manual analysis. Human experts may overlook subtle patterns, but AI algorithms can detect anomalies and correlations across multiple data sources. As AI continuously learns from past incidents, it improves its accuracy over time, becoming increasingly effective at diagnosing issues across diverse systems.
Core Technologies in AI-Powered RCA
AI-powered RCA relies on machine learning models and anomaly detection techniques to identify deviations from normal behavior in complex systems. Tools like autoencoders and isolation forests detect anomalies in real time by analyzing relevant data. Additionally, natural language processing (NLP) is key for parsing unstructured data, such as system logs and user reports, to extract insights and uncover correlations. Together, these AI-driven approaches help organizations identify and resolve issues before they escalate, improving system reliability and performance.
Machine Learning and Predictive Maintenance
Machine learning plays a key role in predictive maintenance by analyzing sensor data to detect early signs of equipment failure. This proactive approach helps organizations schedule maintenance before issues occur, reducing unplanned downtime and extending equipment lifespan. Predictive maintenance, enhanced by anomaly detection, is especially valuable in manufacturing, where equipment failures can cause costly delays. AI systems monitor machines in real time, identifying patterns that signal potential breakdowns, enabling timely intervention, and reducing repair costs and the risk of major failures.
Natural Language Processing for Unstructured Data
NLP is crucial for analyzing unstructured data like error logs and support tickets, allowing AI systems to extract key information and identify patterns missed by traditional methods. Machine learning models, such as BERT, categorize problem reports and prioritize issues based on severity. Named Entity Recognition (NER) further enhances AI-powered RCA by automatically identifying components like error codes, and speeding up diagnosis. Combined with AI’s capacity to process large datasets, NLP is a powerful tool for managing complex technical environments efficiently.
Scalability of AI-Powered RCA
A key advantage of AI-powered RCA is its scalability. While traditional methods struggle with increasing system complexity, AI easily adapts to larger data volumes. AI algorithms can process massive information generated by large-scale operations, offering real-time insights into system behavior as organizations expand. Cloud-based AI further boosts scalability by dynamically allocating resources based on demand, ensuring consistent performance without requiring substantial infrastructure investments. This allows AI-powered RCA to remain effective, regardless of system size or complexity.
The Impact of AI-Powered RCA on Operational Efficiency
Implementing AI-powered RCA offers organizations substantial improvements in operational efficiency. AI can reduce the time required to resolve incidents by 50% or more, translating into significant cost savings, particularly in industries where downtime can be financially devastating. AI’s ability to quickly identify root causes also allows teams to focus on fixing the most critical issues and optimizing resource allocation.
The accuracy of AI-powered RCA is another critical benefit. AI can account for a wider range of variables and data points than human analysts, leading to more reliable diagnoses. For organizations that rely on precision and minimal downtime, the accuracy and speed of AI-powered RCA are invaluable.
In conclusion, Nikhil BharadwajRamashastri’s exploration of AI-powered Root Cause Analysis highlights its transformative potential in modern system optimization. By harnessing the power of machine learning, anomaly detection, and natural language processing, AI-driven RCA not only improves operational efficiency but also helps organizations anticipate problems before they arise. As businesses face increasingly complex technological challenges, the integration of AI-powered RCA will play a crucial role in maintaining system reliability and competitiveness in the digital age.
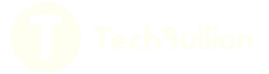