In this modern era, AI-Powered quality control in manufacturing is experiencing a transformative shift through AI integration, reveals new research published in the International Journal of Scientific Research in Computer Science. The comprehensive analysis by Divyansh Jain examines how AI technologies are revolutionizing inspection processes and boosting operational efficiency across industries, while reducing reliance on manual inspection methods.
The Dawn of Intelligent Inspection
Advanced machine learning algorithms are transforming traditional quality control by introducing capabilities beyond human inspection limits. These intelligent systems perform continuous monitoring with exceptional precision, identifying subtle defects that would typically go undetected in fast-paced production environments. The technology enables round-the-clock inspection without fatigue, maintaining consistent quality standards across manufacturing operations.
Breaking the Speed Barrier
AI-driven inspection systems demonstrate remarkable consistency in quality control, even at increased production speeds. The technology provides comprehensive monitoring across entire production cycles while minimizing manual inspection requirements. With detection accuracy improvements reaching 85-95% compared to traditional methods, these systems are setting new standards for manufacturing quality control efficiency.
Smart Sensors Lead the Way
Advanced sensor networks mark a critical evolution in quality control systems, working seamlessly with edge computing to enable real-time monitoring and instant decision-making. This powerful combination gives manufacturers deep insights into their production processes while maintaining exceptional reliability standards. The integration of smart sensors with processing capabilities creates a responsive system that can adapt to manufacturing needs in real-time.
Beyond Visual Inspection
AI’s role in quality control goes beyond basic visual inspection, embracing multiple inspection methods and advanced sensing technologies for comprehensive quality assessment. Modern systems combine visual analysis with diverse sensing tools to create a multi-modal approach, enabling reliable detection of both surface and internal defects. This integrated strategy ensures thorough quality monitoring throughout the entire manufacturing process.
The Human Factor
While automation drives quality control innovation, the human element remains essential. The shift to AI-driven systems demands comprehensive workforce adaptation and thorough training initiatives. Successful implementations emphasize the need for clear operational procedures and robust validation protocols. This balanced approach between technological advancement and human expertise ensures optimal system performance and reliable quality control outcomes.
Economic Impact Drives Adoption
The implementation of automated inspection systems is delivering significant returns through reduced labor costs and enhanced production efficiency. These AI-driven solutions prove especially valuable in high-volume manufacturing, where quality control represents a major operational expense. By detecting issues early in production, these systems substantially reduce material waste and rework needs, leading to measurable cost savings and improved resource utilization.
Future Horizons
The evolution of quality control technology is rapidly accelerating through edge computing and advanced sensor integration. These innovations enable sophisticated quality management approaches that adapt to changing production needs while maintaining consistent standards across various operational scales. The combination of real-time processing and smart sensors creates flexible systems that can evolve with manufacturing demands without compromising quality standards.
Technical Challenges and Solutions
While implementing AI-driven quality control systems presents challenges in infrastructure setup, data management, and system integration, new solutions are emerging to address these barriers. Standardized deployment frameworks and practical guidelines are making the technology increasingly accessible across manufacturing sectors. These developments are helping organizations overcome initial hurdles and successfully integrate AI into their quality control processes.
A New Quality Paradigm
The integration of AI in quality control marks a fundamental shift beyond mere technological advancement, transforming how industries approach quality assurance. By combining real-time monitoring with predictive analytics, manufacturers can now implement proactive quality management strategies that were previously unattainable. This new approach enables early detection of potential issues and automated adjustments, setting new standards for manufacturing excellence.
The Road Ahead
The future of AI-powered quality control shows remarkable promise as new technological advances emerge. These innovations are creating unprecedented opportunities for implementation and optimization across manufacturing sectors. The technology continues to evolve, offering enhanced capabilities for quality assurance while making implementation more accessible and cost-effective for organizations of all sizes.
As Divyansh Jain concludes, successful adoption requires sustained commitment to research and development, balanced with practical considerations for implementation and organizational needs. This strategic approach will help industries maximize the benefits of AI in quality control while managing implementation challenges effectively.
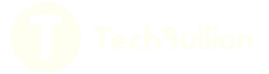