In the era of rapid technological evolution, artificial intelligence (AI) is playing a pivotal role in optimizing next-generation wireless networks. Sai Charan Madugula, a researcher specializing in AI-driven networking solutions, introduces an advanced architecture for managing reusable AI-based applications in 6G networks. His work emphasizes enhancing scalability, minimizing redundancy, and improving efficiency by seamlessly integrating AI into network management frameworks.
Challenges in AI Integration for 6G Networks
Traditional AI implementations in network management have created isolated solutions, leading to inefficiencies. Each AI-based application typically develops its own data collection and feature extraction process, preventing the sharing of insights across systems. This fragmentation results in high computational costs and scalability limitations. Without a unified framework, AI models are often restricted to specific tasks, making reusability difficult.
A Unified Network Architecture for AI Applications
To address these challenges, the proposed architecture introduces a structured approach to integrating AI across 6G networks. It consists of four core components:
- Unified Data Collection Layer – Standardizes data acquisition processes, reducing redundancy and ensuring consistency across AI applications.
- Shared Feature Repository – Enables reusable features to minimize computational overhead.
- Model Management Framework – Supports distributed learning paradigms such as federated and split learning, allowing scalable AI deployment.
- Application Integration Layer – Facilitates cross-domain AI utilization, ensuring interoperability between network components.
By implementing these components, networks can efficiently manage AI resources, reduce duplication, and support cross-application intelligence.
Enhancing Network Performance with AI
AI-driven automation plays a crucial role in optimizing network performance. By leveraging machine learning for traffic prediction, resource allocation, and anomaly detection, networks can enhance efficiency and minimize downtime. Studies indicate that AI-based optimizations improve spectrum efficiency by up to 2x, reduce latency to sub-millisecond levels, and increase network throughput by 10x.
Additionally, AI-enabled resource allocation dynamically adjusts network resources based on real-time demand. Reinforcement learning models have demonstrated a 47.8% increase in dynamic network optimization while reducing energy consumption by 32.5%.
Strengthening Network Security with AI
Cybersecurity remains a major concern for 6G networks due to the increasing number of connected devices. AI-powered security mechanisms provide real-time threat detection and response, significantly improving network resilience. Research indicates that AI-enhanced security frameworks detect 95% of known cyber threats while maintaining a false positive rate below 1%.
The integration of AI-based anomaly detection allows for adaptive threat prevention, leveraging machine learning to identify suspicious patterns. These predictive security models reduce response times to under 100 milliseconds, enabling networks to mitigate risks before they escalate.
AI-Driven Service Quality Management
Ensuring high service quality in 6G networks requires real-time monitoring and adaptive optimization. AI-powered performance monitoring systems track network health, identify bottlenecks, and suggest improvements based on predictive analytics. Implementing AI-driven predictive maintenance reduces network downtime by 45%, improving service reliability.
The application of machine learning models in network slicing enhances service delivery by dynamically adjusting bandwidth allocation. This ensures optimal performance across applications, including ultra-reliable low-latency communications (URLLC) and enhanced mobile broadband (eMBB) services.
Benefits of AI-Powered 6G Network Architecture
The adoption of AI-driven architecture in 6G networks offers several advantages:
- Improved Efficiency – Standardizing AI workflows reduces development time for new network services.
- Scalability – AI models can be reused and adapted across multiple applications.
- Cost Reduction – Centralized AI resource management lowers operational costs.
- Enhanced Security – AI-driven threat detection improves resilience against cyber threats.
- Optimized Resource Utilization – Intelligent traffic management and predictive analytics reduce network congestion.
These benefits contribute to a more resilient network infrastructure, capable of supporting the growing demands of next-generation wireless technology.
Future Directions in AI-Enabled 6G Networks
As AI and 6G technologies continue to evolve, future advancements will focus on improving AI governance, enhancing interoperability, and integrating edge computing for real-time processing. The incorporation of blockchain-based security frameworks will further strengthen AI-driven network architectures, ensuring data integrity.
In conclusion, Sai Charan Madugula’s research highlights the transformative impact of AI on 6G network management. By introducing a scalable and reusable AI-based architecture, his work addresses critical challenges in network optimization, security, and service quality. As the demand for intelligent network management grows, AI-driven frameworks will shape the future of wireless communication, driving efficiency, security, and innovation.
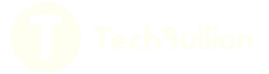