In the ever-evolving landscape of digital payments, security remains a paramount concern for enterprises worldwide. With rising cyber threats and sophisticated fraud tactics, organizations are embracing artificial intelligence (AI) and behavioral analytics to enhance fraud prevention mechanisms. A leader in this transformation, Nagaraju Velur explores how AI-driven security frameworks are redefining enterprise fraud detection.
From Static Rules to Adaptive Intelligence
Traditional fraud detection relied on static, rule-based systems that flagged transactions based on predefined criteria. While effective against known threats, these systems struggled with evolving fraud tactics and generated excessive false positives, often disrupting legitimate transactions. AI-powered fraud detection transforms this approach by leveraging machine learning models that adapt in real-time.
These models analyze vast datasets, identify emerging fraud patterns, and enhance accuracy while reducing false positives. This adaptive intelligence ensures more robust, efficient fraud prevention, keeping pace with sophisticated cyber threats and improving transaction security.
Multi-Layered Machine Learning Frameworks
Modern fraud prevention architectures leverage multi-layered machine learning frameworks to detect complex transaction patterns across vast datasets. These AI-driven systems incorporate deep learning models, neural networks, and ensemble learning techniques to improve detection accuracy.
By continuously analyzing and refining fraud patterns, they enhance real-time threat identification while minimizing false positives. This adaptive approach ensures a seamless user experience without compromising security. The result is an intelligent fraud detection mechanism that evolves dynamically, identifying and mitigating fraudulent activities with increasing efficiency and precision over time.
Behavioral Analytics: Understanding User Patterns
Behavioral analytics is revolutionizing fraud prevention by focusing on user interactions rather than just transactions. AI-driven models analyze browsing behavior, device usage, and transaction frequency to establish a baseline of normal activity. When deviations occur, real-time security measures are triggered, making it harder for fraudsters to bypass detection.
Unlike traditional methods that rely on static rules, behavioral analytics continuously adapts, identifying subtle anomalies indicative of fraudulent activity. This proactive approach enhances security by detecting threats early, reducing false positives, and improving user trust. As cyber threats evolve, behavioral analytics remains a critical tool in safeguarding digital transactions and identities.
Real-Time Anomaly Detection
AI-driven security frameworks process millions of data points per second, enabling real-time anomaly detection. These systems monitor every aspect of a transaction, from device characteristics to network behaviors, and apply advanced correlation techniques to assess risk levels. This proactive approach allows enterprises to detect and block fraudulent transactions before they are completed, significantly reducing financial losses.
Reducing False Positives with Context-Aware AI
Minimizing false positives is a key challenge in fraud detection, as legitimate transactions are often mistakenly flagged as fraudulent. AI-driven fraud prevention systems improve accuracy by leveraging contextual authentication, adaptive decision-making, and multi-stage risk evaluation. These systems analyze transaction context, user behavior, and risk patterns to distinguish between genuine and suspicious activities. As a result, legitimate transactions proceed smoothly, while only high-risk actions undergo further scrutiny, enhancing both security and user experience.
Seamless Integration Across Digital Ecosystems
AI-driven security architectures seamlessly integrate with payment platforms, leveraging transaction metadata, device fingerprints, and user behavioral patterns for real-time threat detection. This comprehensive approach enhances fraud prevention while maintaining a frictionless payment experience.
By continuously analyzing data from multiple sources, these systems adapt to evolving attack vectors without disrupting legitimate transactions. Additionally, dynamic model updates ensure ongoing protection against emerging cyber threats, allowing financial institutions to mitigate risks proactively. This seamless integration strengthens security across digital ecosystems, fostering trust and reliability in modern payment infrastructures.
Future Directions: AI, Quantum Security, and Federated Learning
The future of digital payment security lies in further advancements in AI, including quantum-resistant cryptography and federated learning. While AI continues to evolve, security frameworks must also incorporate privacy-preserving techniques to ensure data protection. Organizations are exploring ways to implement decentralized AI models that can detect fraud across networks while maintaining user privacy.
In conclusion, AI-powered security frameworks play a pivotal role in safeguarding enterprises against financial fraud. By integrating machine learning and behavioral analytics, organizations can achieve a balance between stringent security and seamless user experiences. Nagaraju Velur’s insights into AI-driven fraud prevention highlight the future of digital payments, where intelligent and adaptive security measures redefine enterprise protection.
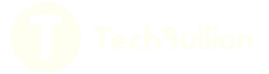