In the modern era, fraud detection has transformed with artificial intelligence (AI), replacing traditional rule-based systems with advanced machine learning models. Danish Khan, a leading expert in AI-driven fraud prevention, examines the evolution of AI-powered security solutions, their influence across various industries, and the future of fraud detection.
The Shift from Rule-Based to AI-Driven Fraud Prevention
The landscape of fraud detection has undergone a major transformation over the past few decades. Initially, static rule-based systems required manual oversight, making fraud detection a time-consuming process. However, as fraudulent tactics became more sophisticated, AI-powered solutions emerged as a more efficient alternative. These advanced systems use machine learning algorithms to analyze extensive datasets, detect irregular patterns, and prevent fraudulent transactions in real time. By leveraging AI, organizations have significantly reduced false positives while improving detection accuracy, allowing them to identify threats faster and more effectively than ever before.
Real-Time Detection Through Pattern Recognition
One of the significant advancements in AI-powered fraud detection is real-time pattern recognition. Unlike conventional methods relying on predefined rules, AI models dynamically learn and adapt to evolving data patterns. These advanced systems analyze vast amounts of transactional history, user behaviors, and interaction patterns to detect fraudulent activities before they escalate. This adaptability makes AI-driven fraud detection especially valuable in high-risk environments such as digital transactions, online marketplaces, and gaming platforms. By leveraging real-time insights, businesses can proactively mitigate risks and enhance security, ensuring a safer and more trustworthy digital ecosystem.
Enhancing Security in Financial Services
Financial fraud remains a major concern as cybercriminals constantly evolve their tactics. AI-driven fraud detection has transformed financial security by enabling institutions to monitor transactions at scale. These systems process millions of transactions per second, identifying suspicious activities with high accuracy. AI-powered tools also reduce investigation times, allowing financial organizations to respond swiftly to mitigate risks and minimize losses. By leveraging machine learning, institutions enhance fraud prevention strategies, ensuring proactive security measures and reducing the impact of fraudulent activities on financial systems.
The Role of AI in E-Commerce Fraud Prevention
E-commerce platforms have increasingly adopted AI-driven fraud detection mechanisms to combat unauthorized transactions, identity theft, and payment fraud. By analyzing behavioral patterns, AI systems can differentiate between legitimate users and fraudulent actors. Advanced AI models have significantly reduced false positives, ensuring a smoother shopping experience for genuine customers while preventing fraudsters from exploiting loopholes in online payment systems.
AI’s Impact on Healthcare Fraud Detection
Fraudulent activities in the healthcare sector, such as false claims and billing fraud, have prompted the need for AI-driven solutions. AI-powered fraud detection models help healthcare organizations analyze claims data, identify irregularities, and prevent fraudulent claims from being processed. These systems have demonstrated high accuracy rates in detecting unusual prescription patterns and billing anomalies, leading to substantial cost savings and improved security.
Overcoming Technical Challenges
While AI-driven fraud detection systems offer numerous advantages, they also face several challenges. Data quality remains a critical factor, as inaccuracies in input data can impact detection efficiency. Additionally, integrating AI models with legacy systems poses compatibility issues for many organizations. To address these challenges, companies are investing in real-time data pipelines, feature engineering, and scalable computing infrastructure to optimize AI fraud detection capabilities.
The Future of Fraud Prevention: Quantum AI and Federated Learning
As AI continues to evolve, the future of fraud detection is expected to incorporate advanced technologies such as quantum computing and federated learning. Quantum-enhanced AI solutions promise faster processing speeds and higher accuracy rates, while federated learning enables organizations to share fraud detection insights without compromising data privacy. These advancements will further strengthen AI-driven fraud prevention systems, making them more efficient and adaptable to emerging threats.
In conclusion, AI integration in fraud detection has strengthened security across industries such as finance, e-commerce, and healthcare. Danish Khan highlights how AI-driven innovations enhance fraud prevention by reducing losses and improving detection efficiency. As organizations implement advanced AI models, fraud detection is becoming more resilient, ensuring a safer digital environment. With ongoing technological advancements, AI-powered fraud detection systems will continue to evolve, providing robust protection against emerging threats and reinforcing trust in digital transactions.
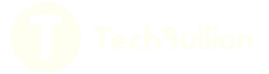