In an era of exponential data growth, enterprises face unprecedented challenges in managing and migrating vast amounts of information. A groundbreaking paper by Vishnu Iyengar, an industry expert with decades of experience in managing massive-scale systems and platforms, sheds light on innovative approaches to navigate these complex transitions. Published in the International Journal of Computer Engineering and Technology, the work offers a comprehensive roadmap for organizations undertaking large-scale data migrations.
The Data Dilemma
As businesses evolve, the need to transfer, update, and reorganize data grows increasingly vital. Whether due to technological modernization, regulatory mandates, or the pursuit of better performance, data migration is now a critical process for organizations of all sizes. However, this task is fraught with challenges—data integrity, security risks, downtime, and compliance concerns can pose significant threats. Missteps during migration can lead to costly errors or data loss, making careful planning and execution essential to ensure seamless transitions and maintain business continuity.
Bridging Old and New: The Hybrid Approach
Data migrations typically follow two approaches: the “big bang,” transferring data all at once, or gradual, occurring in stages. However, traditional methods aren’t suited for the scale of modern enterprises, requiring a hybrid strategy that combines both. This segments data by complexity, criticality, and volume, applying the best migration method for each. This approach offers greater flexibility, reduces risk, and minimizes disruption during large-scale data migrations.
AI: The Migration Maestro
One of the most exciting innovations highlighted in the study is the integration of artificial intelligence into the migration process. AI-driven tools are revolutionizing every phase of data migration:
- Pre-migration: AI systems analyze vast datasets with unprecedented speed and accuracy, identifying data quality issues and proposing optimal transformation strategies.
- Execution: During the migration, AI continuously monitors performance metrics, dynamically adjusting resource allocation to maintain peak efficiency.
- Post-migration: Even after the transition, AI plays a crucial role in documentation and knowledge management, ensuring that system knowledge remains accurate and comprehensive.
The Parallel Universe of Data
To minimize downtime and ensure seamless business continuity during data migration, the paper advocates for establishing parallel infrastructures. This approach allows legacy and new systems to operate simultaneously, facilitating real-time data synchronization between them. By enabling both systems to function concurrently, organizations can efficiently transition without disrupting ongoing operations. Additionally, this setup provides a crucial safety mechanism for quick rollbacks, allowing businesses to revert to the old system if issues arise, minimizing risks and ensuring smoother transitions during large-scale migrations.
The ETL Triad: Extract, Transform, Load
At the core of successful data migration efforts is the ETL (Extract, Transform, Load) process. The research advocates for a streaming or pipeline-based approach, where data flows continuously in smaller, manageable batches rather than being processed in bulk. This technique significantly reduces storage overhead, as data does not accumulate in large volumes awaiting processing. Moreover, the pipeline model facilitates early detection of errors, which is crucial for mitigating risks and ensuring smoother, more efficient large-scale migrations by addressing issues in real time.
Validation: The Unsung Hero
Often overlooked, the validation phase is crucial for ensuring data integrity. The study emphasizes the importance of comprehensive checks for completeness, accuracy, and consistency. Automated comparison tools and statistical sampling techniques are recommended for efficiently handling large datasets.
The Graceful Cutover
Perhaps one of the most innovative aspects of the proposed methodology is the approach to the final transition or “cutover” phase. Instead of a sudden switch, the study suggests a gradual redirection of traffic to the new system. This process begins with read operations, slowly increasing the proportion of queries directed to the new system as confidence builds.
Lessons for the Future
As data environments continue to evolve and scale, the importance of refining migration strategies cannot be overstated. The research underscores the need for organizations to invest in AI-driven solutions and automated processes, preparing them for the challenges of future data migrations.
In conclusion, Vishnu Iyengar‘s comprehensive study offers a blueprint for organizations navigating the complex world of large-scale data migration. By embracing innovative strategies and cutting-edge technologies, enterprises can ensure both data integrity and business continuity in an ever-changing technological landscape.
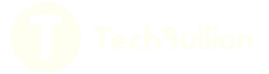