In a rapidly evolving digital landscape, organizations constantly seek innovative solutions to optimize cloud resource management. AI-driven predictive analytics has emerged as a game-changer, enabling businesses to move beyond traditional resource allocation methods. Siddharth Kumar Choudhary, a leading researcher in this domain, explores how artificial intelligence (AI) and machine learning (ML) revolutionize cloud infrastructure. His research presents a strategic framework that helps organizations maximize efficiency, reduce costs, and enhance overall performance.
The Limitations of Traditional Cloud Management
Cloud resource allocation relied on static thresholds and reactive scaling policies for years. However, such methods have proven inadequate in handling the complexities of modern workloads. Studies show that nearly 30% of cloud resources remain underutilized due to inefficiencies in traditional allocation strategies. As workloads fluctuate dynamically, manual adjustments often lead to over-provisioning or underutilization, increasing operational costs and reducing system efficiency.
AI and Machine Learning: The Game Changers
Adopting AI-driven solutions marks a shift from reactive to proactive resource management. AI models can analyze historical data patterns, predict future workload demands, and allocate resources dynamically. For instance, predictive models based on Long Short-Term Memory (LSTM) networks have achieved up to 92% accuracy in forecasting cloud resource demands. This level of precision allows organizations to significantly reduce over-provisioning while ensuring seamless performance.
Real-Time Monitoring for Smarter Allocation
One of the most significant innovations in cloud optimization is real-time monitoring. AI-powered systems continuously track key performance indicators such as CPU utilization, memory consumption, and network throughput. Advanced monitoring frameworks have led to a 55% reduction in response time for resource allocation, improving overall system reliability by 70%. With automated feedback loops, organizations can dynamically fine-tune their resource management strategies, maintaining efficiency even during unpredictable workload surges.
Cost Optimization Through AI Integration
AI-driven optimization strategies contribute significantly to cost savings. Studies indicate that organizations adopting predictive analytics for cloud management have reduced operational costs by 45%. Intelligent scheduling algorithms allocate resources based on priority and budget constraints, minimizing waste and maximizing efficiency. This cost-conscious approach ensures that businesses can maintain high performance without unnecessary expenditure.
Energy Efficiency and Sustainability
Sustainability is becoming a priority in cloud computing, with AI playing a crucial role in reducing energy consumption. Energy-aware load balancing techniques have enabled organizations to cut power usage by up to 40%. These AI-driven systems optimize workload distribution based on energy efficiency metrics, balancing performance and sustainability. As environmental concerns rise, such advancements are set to redefine cloud computing strategies globally.
Seamless Integration Across Cloud Environments
Cloud infrastructure has evolved beyond single-platform deployments, with hybrid and multi-cloud environments becoming the norm. This shift demands advanced integration strategies to ensure seamless interoperability. AI is crucial in optimizing data flow, automating workload distribution, and enhancing cross-platform communication. Research shows that standardized protocols and middleware solutions have reduced data transfer latency by 73% while improving system interoperability by 82%. By leveraging intelligent integration frameworks, organizations can maintain high performance across diverse cloud platforms while ensuring security and regulatory compliance. Embracing these innovations enables businesses to achieve flexibility, scalability, and resilience in an increasingly interconnected digital landscape.
The Future of AI-Driven Cloud Optimization
The future of cloud resource management is rapidly evolving, driven by automation and AI-driven intelligence. A major trend is the rise of edge computing, where AI optimizes resource allocation in real time across distributed network locations, reducing latency and improving efficiency. Additionally, quantum computing is being explored to handle complex workloads at unprecedented speeds, promising breakthroughs in optimization and problem-solving. These advancements enable organizations to scale dynamically, enhance performance, and reduce operational costs. Businesses that invest in these cutting-edge technologies will gain a competitive edge, ensuring resilience and adaptability in an increasingly digital and data-driven world.
In conclusion, Siddharth Kumar Choudhary’s research highlights the transformative impact of AI-driven predictive analytics on cloud resource optimization. Organizations can significantly enhance resource utilization, cost efficiency, and overall performance by integrating AI and machine learning. The shift from traditional to AI-driven approaches underscores the necessity of structured implementation frameworks for long-term sustainability. As cloud technologies evolve, businesses must embrace continuous innovation to remain competitive in the digital era.
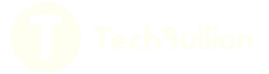