The financial industry has undergone a significant transformation in recent years, driven largely by advancements in artificial intelligence (AI) and machine learning (ML). These technologies have brought powerful predictive analytics to the forefront, allowing financial institutions and businesses to make smarter, data-driven decisions.
The Role of AI and Machine Learning in Finance
Artificial intelligence and machine learning are not just buzzwords in the finance world; they are fundamental tools that are reshaping how financial institutions operate. AI involves creating systems that can perform tasks typically requiring human intelligence, such as learning, reasoning, and problem-solving. Machine learning, a subset of AI, is the process by which computers improve their performance over time by analyzing data and recognizing patterns without explicit programming.
These technologies have already had a profound impact on various aspects of finance, including fraud detection, algorithmic trading, risk management, and credit scoring. By leveraging massive datasets, AI and machine learning algorithms can process and analyze information at speeds and scales far beyond human capability.
AI & Machine Learning in Finance:
The Heart of AI and Machine Learning in Finance
At the core of AI and machine learning applications in finance is predictive analytics. Predictive analytics uses historical data, statistical algorithms, and machine learning techniques to identify the likelihood of future outcomes. In the finance sector, this capability is invaluable as it allows institutions to forecast market trends, predict stock prices, assess risks, and make more informed investment decisions.
Market Predictions and Forecasting
AI-powered predictive models can analyze vast amounts of market data, including historical trends, economic indicators, and real-time information, to forecast market movements. These models can predict asset prices, stock movements, and market volatility with a high degree of accuracy. By using AI and machine learning to analyze patterns and correlations in financial data, financial institutions can gain a competitive edge in decision-making, making more informed predictions about market conditions and asset performance.
For example, hedge funds and investment banks rely heavily on predictive analytics to gain insights into future market trends. These tools help them make strategic decisions, allocate capital effectively, and adjust their investment portfolios based on projected market movements. Predictive analytics can also help identify emerging market trends, giving firms an advantage in positioning themselves for future opportunities.
Risk Management and Fraud Prevention
One of the most critical applications of predictive analytics in finance is in the area of risk management. By analyzing historical data and recognizing patterns, AI and machine learning models can identify potential risks before they become critical issues. This allows financial institutions to proactively manage risks related to credit, market fluctuations, and even operational challenges.
In the case of credit risk, machine learning models can analyze borrowers’ credit histories, spending behaviors, and other relevant factors to predict the likelihood of default. This helps lenders make better decisions when approving loans, reducing the risk of non-performing loans. Predictive analytics can also assess the risk of market fluctuations, enabling institutions to hedge against potential losses and optimize their portfolios.
Fraud detection is another area where AI and machine learning are proving invaluable. Machine learning algorithms can analyze transaction data in real time, identifying suspicious behavior and flagging potentially fraudulent transactions. By learning from past fraud cases and continuously improving, these systems become increasingly effective at detecting new and evolving fraud tactics, offering a more robust defense against financial crime.
Customer Personalization and Experience
In addition to enhancing decision-making and risk management, AI and machine learning are also playing a pivotal role in improving customer experiences. Predictive analytics can be used to personalize financial services and products based on individual customer behaviors, preferences, and needs. By analyzing customer data, financial institutions can offer tailored recommendations, investment strategies, and even personalized loan offers.
For instance, banks are using AI-powered chatbots to provide personalized assistance to customers, answering queries, recommending products, and even helping with budgeting. These chatbots learn from each interaction and become increasingly adept at understanding customer preferences, enabling them to offer more accurate advice and solutions. Predictive analytics also helps banks predict customer needs, allowing them to offer relevant products at the right time. For example, a customer nearing retirement might receive suggestions for investment plans or insurance products tailored to their future goals.
How AI and Machine Learning Are Enhancing Financial Decision-Making
Financial decision-making is complex, involving various factors such as market conditions, risk appetite, and financial goals. AI and machine learning offer powerful tools to enhance this process, making decisions faster, more accurate, and more data-driven.
Algorithmic Trading
One of the most well-known applications of AI and machine learning in finance is algorithmic trading. AI-driven trading algorithms can analyze market data, identify trends, and execute trades at high speeds and volumes. These algorithms use predictive models to forecast short-term price movements, enabling traders to make real-time decisions based on data-driven insights. This type of trading is far more efficient than traditional methods and has revolutionized the way financial markets operate.
Machine learning models can adapt to changing market conditions, learning from past trades and adjusting their strategies accordingly. By leveraging predictive analytics, these algorithms can identify profitable trading opportunities and minimize risks, all while operating with a speed and precision that humans cannot match.
Portfolio Optimization
Another area where AI and machine learning are making a significant impact is in portfolio optimization. Traditional portfolio management methods rely on human judgment and static strategies, often missing out on opportunities or failing to adequately mitigate risks. AI and machine learning, on the other hand, can continuously monitor portfolio performance and market conditions, adjusting asset allocations in real time based on predictive analytics.
Using machine learning algorithms, financial institutions can create optimized portfolios that maximize returns while minimizing risk. These models analyze a variety of factors, such as historical performance, economic indicators, and market conditions, to forecast how different assets will perform in the future. This allows investors to make more informed decisions about which assets to include in their portfolios, ultimately leading to better long-term performance.
The Future of AI and Machine Learning in Finance
The role of AI and machine learning in finance is only set to grow in the coming years. As these technologies continue to evolve, their potential to transform the industry will only expand. Predictive analytics will become even more sophisticated, with AI models becoming capable of processing even larger datasets and making more accurate predictions. The use of alternative data sources, such as social media sentiment or satellite imagery, will provide deeper insights into market trends and financial behavior.
AI and machine learning will also play a critical role in improving regulatory compliance and transparency. As financial institutions face increasing scrutiny from regulators, AI-powered tools can help ensure compliance with ever-changing regulations. These tools can automate compliance tasks, monitor transactions for suspicious activities, and provide real-time reports, ensuring that institutions stay ahead of regulatory requirements.
Ethical Considerations and Challenges
While the benefits of AI and machine learning in finance are clear, there are also ethical considerations and challenges that need to be addressed. One of the main concerns is the potential for bias in predictive models. If the data used to train AI algorithms is biased, the resulting predictions and decisions may also be biased, leading to unfair treatment of certain individuals or groups. Ensuring that AI models are trained on diverse and representative data is essential for minimizing this risk.
Additionally, there is a need for greater transparency in AI-driven decision-making processes. As financial institutions increasingly rely on AI and machine learning, it is crucial that these systems are explainable and understandable to stakeholders. This transparency will help build trust in AI-powered decision-making and ensure that these technologies are used responsibly.
Conclusion
AI and machine learning are transforming the finance industry, particularly through predictive analytics that drive smarter, more informed decision-making. From enhancing market forecasting to improving risk management and personalizing customer experiences, these technologies are enabling financial institutions to make data-driven decisions faster and with greater accuracy. Their impact on finance will only deepen, offering new opportunities for innovation and growth. However, it is essential to address ethical challenges and ensure that these technologies are used responsibly, ensuring that the financial industry remains transparent, fair, and trustworthy.
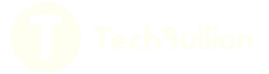