In an era defined by digital acceleration and mounting cybersecurity threats, Srinath Reddy Palla brings a pioneering perspective to the financial services sector. A noted thought leader, his work explores how artificial intelligence (AI) is revolutionizing security protocols, offering banks and financial institutions new ways to combat fraud.
Moving Beyond Traditional Defenses
Fraud detection in financial services has traditionally depended on rule-based systems with static thresholds and manual processes. However, these methods are no longer sufficient against today’s increasingly sophisticated fraud tactics. Cyber threats have evolved, overwhelming first-generation defenses and leading to a surge in identity theft, payment fraud, and account takeovers. Static approaches cannot keep pace with the speed and complexity of modern attacks. As a result, the financial industry faces a critical need for dynamic, intelligent security solutions that can adapt in real-time, ensuring stronger protection against ever-evolving threats and reducing vulnerabilities across digital platforms.
Artificial Intelligence as a Game-Changer
Artificial Intelligence is revolutionizing security, shifting frameworks from reactive defenses to proactive strategies. Using machine learning and behavioral analytics, institutions can now develop dynamic, real-time risk profiles that adapt as customer behavior evolves. Neural networks, for example, achieve a remarkable 93.5% accuracy in detecting card-not-present fraud, significantly outperforming traditional systems. Adaptive AI models continuously learn from fresh data, enabling organizations to anticipate and counter emerging fraud tactics effectively. This continuous evolution ensures that security measures stay ahead of sophisticated threats, positioning AI not just as a tool but as a transformative force in modern fraud prevention and risk management.
The Power of Behavioral Analytics
Behavioral analytics has emerged as a powerful tool in detecting suspicious activities. Modern systems monitor hundreds of behavioral indicators, from navigation patterns to transaction behaviors, across various interaction channels. By examining subtle deviations from typical user behavior, organizations can now detect potential fraud with over 90% accuracy. These advanced technologies not only excel in anomaly detection but also support continuous authentication. This approach minimizes the need for disruptive security checks, maintaining strong protection while enhancing the user experience. As a result, institutions can proactively prevent fraud without compromising convenience or security.
NLP and the Fight Against Social Engineering
Natural Language Processing (NLP) has emerged as a crucial tool in detecting fraud that originates through communication channels. Modern NLP applications scrutinize linguistic patterns, emotional cues, and semantic inconsistencies across emails, chats, and voice calls. Transformer-based models, with detection rates exceeding 90%, now empower institutions to spot social engineering attacks like phishing and business email compromises much earlier and more accurately than keyword-based systems ever could.
Real-Time Transaction Monitoring: A New Standard
Real-time monitoring systems represent a leap forward in fraud prevention. By analyzing transactions against hundreds of dynamic risk indicators within milliseconds, institutions can intercept fraudulent activities before they result in monetary loss. These systems offer a dual advantage: they reduce false positives—an operational bottleneck for traditional systems—while boosting detection accuracy for both known and novel fraud patterns.
Integrated Security for Cloud Environments
Modern financial services rely heavily on cloud platforms, and securing these environments requires integrated, layered architectures. AI-driven security frameworks are designed to process massive data volumes, apply sophisticated risk analytics, and orchestrate rapid threat responses—all in real-time. Institutions leveraging such frameworks have achieved up to 82% higher fraud detection rates and 56% faster threat mitigation compared to those relying on fragmented, traditional systems.
Continuous Innovation and Future Directions
While AI has significantly strengthened financial security frameworks, there are still critical challenges to overcome. Model explainability remains a major concern, particularly for regulatory compliance and customer dispute resolution. Privacy-preserving machine learning, including federated learning and differential privacy techniques, is an area of active development, balancing security needs with customer data protection. Meanwhile, adversarial resilience is becoming a key frontier, as cybercriminals increasingly target AI systems themselves.
In conclusion, Srinath Reddy Palla‘s work shines a light on the transformative role of AI in safeguarding the future of finance. As financial institutions embrace these cutting-edge innovations, they not only protect their assets but also build deeper trust with customers. With continuous research and refinement, AI-driven fraud detection stands poised to deliver smarter, faster, and more secure financial ecosystems for the digital age.
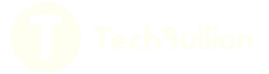