The increasing reliance on digital transactions in retail has made the sector a prime target for fraudsters, prompting the need for advanced security measures. In this article, Abhinav Chunchu delves into the innovative ways Artificial Intelligence (AI) is reshaping fraud detection in retail. By adapting to sophisticated fraud tactics, AI offers a dynamic defense that traditional rule-based systems cannot match. This exploration highlights how AI methodologies, real-time detection, and data-driven approaches are revolutionizing retail fraud prevention and enhancing customer experience.
The Evolving Threat of Retail Fraud
The rise in e-commerce has led to a parallel increase in fraudulent activities. Fraudsters employ advanced methods such as account takeover, card-not-present fraud, and synthetic identity fraud. Traditional rule-based fraud detection systems struggle to keep pace with these sophisticated tactics due to their reliance on static rules. In contrast, AI can dynamically adapt to new fraud patterns, offering a more robust defense. By analyzing thousands of data points in real-time, AI-powered systems enhance fraud detection and reduce false positives, thereby improving the customer experience.
AI Methodologies in Fraud Detection
AI employs a range of methodologies to detect and prevent fraud, including supervised, unsupervised, and reinforcement learning. Supervised learning uses labeled datasets to identify legitimate and fraudulent transactions, reducing fraud in e-commerce by up to 73% and detecting up to 98.9% of credit card fraud cases. Unsupervised learning identifies anomalies without prior labeling, effectively spotting new fraud types with a 95% detection rate. Reinforcement learning adapts to evolving patterns, improving detection rates by 20% over static models. Often, these approaches are combined into ensemble models to harness their strengths, offering a robust and adaptable defense against evolving fraud tactics in retail transactions.
Data: The Backbone of AI-Driven Fraud Detection
High-quality data is crucial for the success of AI-driven fraud detection systems. AI models analyze vast amounts of transaction data, behavioral data, and historical fraud data to identify fraudulent patterns. Feature engineering, a process of creating relevant features from raw data, plays a critical role in improving model accuracy by up to 25%. Preprocessing steps like data cleaning, normalization, and handling imbalanced data are essential to enhance the models’ effectiveness. With proper data preparation, organizations can significantly reduce fraudulent activities and financial losses.
Real-Time Fraud Detection: An AI-Driven Breakthrough
Real-time fraud detection, powered by AI, represents a major advancement in transaction security, enabling instant analysis and rapid decision-making. These systems excel in high-speed data processing, capable of analyzing vast transaction volumes in milliseconds—Visa’s AI-powered platform, for instance, processes over 500 million transactions daily, each in just about 1 millisecond. They dynamically adjust detection rules based on emerging patterns, significantly reducing false positives while maintaining high fraud detection rates. Adaptive learning is a core feature, allowing real-time systems to continually update their models, outperforming static models by up to 20% in identifying new fraud patterns. Despite challenges like data latency and model drift, these AI models uphold accuracy and speed through solutions like edge computing, automated model monitoring, and risk-based authentication.
Overcoming Challenges with AI
The implementation of AI in fraud detection poses challenges such as data quality, false positives, regulatory compliance, and system integration. However, innovative solutions are emerging to address these issues. Enhancing data quality through data cleaning and integration platforms improves model performance, while synthetic data generation using techniques like generative adversarial networks (GANs) helps mitigate data imbalance. To balance false positives and negatives, dynamic threshold tuning and ensemble methods are employed to reduce false positives, thereby minimizing customer friction while maintaining accurate fraud detection. Additionally, to meet regulatory compliance, privacy-preserving techniques like federated learning and explainable AI models are becoming essential, ensuring adherence to data privacy regulations without compromising fraud detection rates.
In conclusion, as Abhinav Chunchu highlights, AI is revolutionizing retail fraud detection by offering sophisticated and adaptable solutions to evolving threats. Through methodologies like supervised, unsupervised, and reinforcement learning, AI enhances detection accuracy and reduces false positives, thereby improving the overall customer experience. Real-time analysis and adaptive learning further strengthen this defense, ensuring rapid responses to emerging fraud patterns. By overcoming challenges related to data quality, compliance, and integration, AI solidifies its role as an essential tool for safeguarding digital transactions in the retail sector.
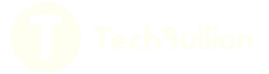