TechBullion invited Maksim Kariagin, an expert and former Director of Research and Analysis, to share his insights on the transformative impact of AI and data science within the music industry. In this article, Mr. Kariagin delves into how these technologies are revolutionizing music production, distribution, and audience engagement, offering readers a comprehensive view of AI’s pivotal role in shaping the future of entertainment. Through his expertise, the article illuminates key advancements and trends that are setting new standards in music innovation and accessibility.
***
The music industry is experiencing a profound transformation, driven by advancements in artificial intelligence (AI) and data science. These technologies are reshaping everything from how music is created and distributed to how fans engage with their favorite artists. In this article, we will explore how AI and data science are revolutionizing the music industry, delving into areas such as AI-driven music composition, personalized listening experiences, predictive analytics, and even the ethical considerations of AI’s growing influence.
AI in Music Creation: New Frontiers of Creativity
One of the most exciting applications of AI in music is in music composition. AI-powered platforms can now assist artists in generating melodies, harmonizing tracks, and even composing entire songs. These tools open up new creative possibilities for musicians of all experience levels, enabling them to experiment with different genres, styles, and structures.
Boomy: Boomy instantly lets users-even those who have never taken musical training-make songs. The AI models on the website help them compose a full song; hence, users can select a style and tone they want and publish their works on Spotify. Boomy democratized music creation and let tools previously out of reach be given to more creators.
Amper Music: Amper is an AI-powered platform that helps in composing and producing music. It targets creators looking for royalty-free music; hence, it produces soundtracks for video content, games, and advertisements. Having a simple interface, it allows users to edit the composition by selecting tempo, genre, and mood, thus allowing creativity in the fastest time.
- Another innovative platform worth the mention is AIVA (Artificial Intelligence Virtual Artist), which allows musicians to collaborate with AI in creating new works. Initially designed for producing classical music, AIVA has expanded to generate compositions for films, video games, and commercials. With AI handling much of the technical aspects of composition, artists can focus more on the creative vision behind their music. AIVA’s creations have been featured in several notable projects, demonstrating its utility in professional music production.
Python Librosa Library: Librosa is an open-source Python library that is mainly utilized for audio and music analysis. It sees a large number of applications in research related to MIR and is useful while developing AI-based music applications. Some of the key features of librosa include:
- Audio manipulation and processing: It provides various time-domain operations such as resampling, trimming silences, and loading audio, and frequency-domain operations like computing a spectrogram.
- Feature Extraction: Librosa contains a variety of methods for the extraction of the most salient features from audio.
- It includes Mel-frequency cepstral coefficients (MFCC) features, which are some of the most usable features for the classification of audios.
- Chroma features, which are useful for analyzing harmonic content;
- Tempo and tracking of beats.
- Music theoretical analysis, which basically helps analyze rhythm, harmony, and structure useful for understanding musical composition. Visualization: Integration with Matplotlib allows for the visualization of waveforms, spectrograms, and other relevant features for further analysis.
Librosa finds very relevant applications in AI-based generation and composition analysis of music and is also being used to develop AI-based mechanisms to recommend and enhance music. Some other related libraries include the following.
Echo Nest: is a music intelligence platform and was acquired by Spotify back in 2014. It was extremely important in studying and analyzing music in a manner to help facilitate better user experiences with the music streaming websites. The main functionalities of Echo Nest include the following :
- Music Recommendation/Discovery: It analyzed large volumes of music data to provide each listener with personalized recommendations, using algorithms to evaluate variables such as rhythm, key, and tempo, along with song popularity.
- Music Data Analytics: It provides users with exhaustive information on tracks, including acoustic features and metadata about the artist and song.
- Predictive analytics: It would predict which songs are likely to hit the charts based on data from streaming patterns, social media mentions, and many other sources.
Echo Nest provides the core of the recommendation algorithms in such systems as Spotify, which build your playlists with content on tastes similar to that from your Discover Weekly playlists. The platform advanced the idea of tailoring music discovery through data science, which still today is a very important backbone for the industry.
These AI-powered platforms are democratizing music creation. With advanced composition tools now available to a global audience, independent musicians can produce professional-quality music without the need for expensive studio equipment or industry connections. AI thus levels the playing field, allowing more creators to share their art with the world.
AI-Driven Audio Engineering and Sound Enhancement
AI is also making waves in the technical side of music production, particularly in audio engineering and sound enhancement. This technology can automate processes such as mixing and mastering, tasks that traditionally require years of experience and specialized equipment.
LANDR is a well-known AI platform that offers automated mastering services. By analyzing the frequency and dynamic range of tracks, LANDR can apply professional-grade mastering techniques to improve sound quality. This makes mastering more accessible and affordable for independent artists who may not have access to high-end audio engineering studios.
LANDR is a multicultural community of creators, consisting of more than 1.8 million creators spanning over 100 countries, major labels such as Warner Music Group, Disney, and Atlantic Records; famous songwriters like Diane Warren; musicians such as MachineDrum and Caleb Groh.The platform includes DJs, artists, engineers, musicians, producers, and record labels. Many people reported using LANDR’s AI driven mastering platform in order to enhance their music, these two individuals shared their story on their experience with it. Further, AI mastering at LANDR can help independent artists achieve great improvements in not only sound quality but also commercial success with many high increases in streaming metrics.
AI is also being used to restore and enhance old recordings, clean up live performances, and even isolate vocals or instruments from existing tracks. This is especially valuable for sound engineers working with archival recordings or live music, where achieving studio-quality sound is a challenge. Tools like RX 9 Audio Editor from iZotope use AI to repair distorted audio and remove background noise, making it easier to restore vintage recordings to their original clarity. Find out more about iZotope RX 9.
Personalized Listening Experiences Powered by AI
AI is not just transforming music creation; it’s also changing how listeners discover and engage with music. Streaming platforms like Spotify, Apple Music, and YouTube Music rely heavily on AI and machine learning algorithms to provide personalized recommendations that cater to individual tastes.
Spotify’s Discover Weekly playlist is a standout example of how AI can curate personalized listening experiences. The platform uses collaborative filtering algorithms to analyze users’ listening habits, combining this data with the preferences of similar users to suggest new tracks and artists. By leveraging machine learning, Discover Weekly creates a highly personalized playlist for each user, introducing them to music they might not have otherwise discovered.
AI also enhances the listening experience through natural language processing (NLP), which can analyze the lyrics and emotional tone of songs. This allows platforms to recommend music that aligns with a listener’s mood or current emotional state. For example, the Mood Playlist feature on Apple Music curates tracks based on users’ feelings, providing a deeply personalized musical experience. AI algorithms analyze the tempo, key, and lyrics of songs to match specific moods such as “chill,” “energetic,” or “sad.”
Predictive Analytics and Market Trends: Shaping the Future of Music
In addition to transforming how music is made and consumed, AI is becoming an essential tool for predicting market trends and shaping the future of the music industry. Record labels, streaming platforms, and marketers use AI-powered analytics to forecast emerging trends, identify breakout artists, and predict which songs are likely to become hits.
Chartmetric, for example, is a data analytics platform that uses AI to track artist performance across streaming platforms and social media. By analyzing vast amounts of data—such as streaming statistics, playlist placements, and social media engagement—Chartmetric can provide valuable insights into market trends. Record labels and managers use these insights to identify rising stars and make data-driven decisions about promotions and marketing strategies.
Predictive analytics can also be used to forecast concert attendance and optimize touring schedules. By analyzing ticket sales, social media engagement, and past performance data, AI can predict which locations are most likely to sell out, helping promoters maximize profitability. AI tools can also identify the best times for artists to release new music or plan tours based on fan engagement trends.
AI and Ethical Considerations in Music
While AI offers incredible opportunities for innovation, it also raises important ethical considerations. As AI becomes more integrated into music creation and distribution, questions arise about ownership, creativity, and bias.
With AI opening unimaginable prospects for creativity, there are some critical ethical issues, especially with the recent trend in AI-generated music. The most basic and larger question resides over ownership and rights to intellectual property as AI technologies such as Suno AI take center stage in creating music indistinguishable from that made by a human. Such services raise questions such as: who, in fact, owns AI-generated music, and do human artists, whose works were used as training material for AI algorithms, have any claims for compensation?
For instance, Suno AI lets its users create music in various styles and genres by scanning reams of already-published music. The point of contention arises when those models, trained without any permission from the artists or labels, use copyrighted music. Consequently, the disputes arise where more traditional creators of music claim this is the misuse of their intellectual property with neither recognition nor financial reward.
These issues are by no means limited to Suno AI: Composers such as Amper Music and AIVA that have been mentioned earlier in this article may be derived from already existing styles or subjects of copyright. These types of AI-generated music can be quite advanced, leaving music labels and artists more concerned with AI technologies that could devalue human artistry by pushing into more robust enforcement over copyrights and equitable compensation in the utilization of existing music within AI models.
The above debate automatically indicates that, along with the intellectual property of human creators, there should be legal and ethical frameworks regarding the protection of AI innovation.
The other prominent disputes that have evolved relate to ownership and creative rights of music produced with the help of AI while it has taken over the creation of music. It could involve the most outstanding issue regarding training issues concerning AI models with copyright material, and also the replacement of human artists.
Examples include Sony Music, Universal Music Group, and Warner Music Group, each weighing an alliance with other majors that reportedly seek to file a class action against AI-generated music content platforms. The labels argue that the AI platforms violate intellectual property rights by using copyrighted songs in their training datasets without proper permission or license. In turn, this raises concerns over ownership and payments to rights holders of artists whose music is used to train the AI without permission. Though the labels have not filed a formal lawsuit, they have been quite vocal concerning the ethical uses of AI-generated content and how it could devalue original human creativity.
This raises a conflicted evolution that repeats larger debates within industries reliant on creative works where AI tools are framed both as opportunities and as threats to traditional business models. As the music industry navigates its way through these changes, debates about creativity, copyright, and bias will undoubtedly continue to form the vanguard of disputes and court battles.
What this underlines is the greater ethical challenge at large: how to balance innovation with protection of human creators and their intellectual property.
Another complex issue when it comes to AI-generated music is Intellectual property (IP) ownership. Who owns the rights to a song composed by an AI— the developer of the AI system or the musician who provided the input? Current legal frameworks struggle to address this issue, leading to calls for new laws that account for AI’s role in creative industries.
Another concern is that AI-driven recommendation algorithms may reinforce existing biases, giving preference to mainstream genres and established artists while underexposing emerging or experimental music. For example, if an artist’s music doesn’t fit neatly into predefined categories, they may struggle to gain exposure on platforms dominated by AI-curated playlists. Addressing these biases is crucial to ensuring AI-driven systems promote diversity and innovation in music, rather than homogenizing the industry.
Conclusion: A Harmonious Future for AI and Music
We can conclude that AI has transformed the music industry in ways unimaginable only a few years ago. From AI-generated compositions and audio engineering to personalized listening experiences and predictive analytics, data science is changing every point in how music is made, distributed, and consumed. But as technologies advance, issues of ethics, intellectual property rights, among a host of other challenges, need to be addressed.
A committee that sorts out all the issues with AI alignment could, perhaps, be one way out in the controversies surrounding AI-generated music. It would be an amalgamation of industry stakeholders like artists, labels, AI developers, and lawyers who put together a framework that sees AI-driven innovations benefit all parties. That would be the committee that could balance technological innovation with the protection of human creators through guidelines on the use of copyright and ownership of works created with AI, while leaving artists with fair compensation whose music is used in AI training models.
With such frameworks set up, the integration of AI in music therefore forms a powerful new partnership between technology and art. With this AI power at their fingertips, artists, producers, and listeners will be well-positioned to enjoy more creative, personalized, and efficient music experiences.
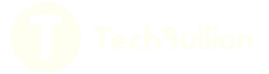