In this rapidly growing digital world, Venkata Krishna Reddy Kovvuri, a leading expert in data engineering, explores how Artificial Intelligence (AI) is revolutionizing cloud computing. With organizations managing vast data volumes daily, traditional methods are becoming inadequate. AI tackles these challenges through automation and innovations like intelligent pipelines, schema matching, and real-time anomaly detection. These advancements significantly enhance efficiency, data accuracy, and scalability, enabling organizations to focus on insights and driving success in sectors such as finance, healthcare, and e-commerce.
Automated Pipelines: Efficiency Reimagined
One of the most significant advancements AI brings to data engineering is the automation of pipeline generation. Traditionally, building data pipelines required manual configurations, a process prone to errors and inefficiencies. AI-driven systems analyze historical data flows and infrastructure patterns to automatically generate optimized configurations, reducing manual effort by up to 75%.
These intelligent pipelines feature dynamic resource allocation, self-healing mechanisms for error recovery, and automated workload distribution, all of which contribute to a 40% reduction in processing time. Organizations benefit from greater reliability and scalability, as AI-enabled pipelines adapt to fluctuating workloads and data complexities in real time. This innovation allows businesses to seamlessly handle the increasing demands of data integration without compromising performance.
Precision with Intelligent Schema Matching
Schema matching—the process of aligning disparate data structures—is critical for data integration, especially in environments with diverse data sources. Traditional methods of schema matching relied on predefined rules, which were often inflexible and error-prone. AI has revolutionized this process by employing deep learning models for semantic analysis, achieving unprecedented accuracy of up to 92%.
AI-powered schema matching systems analyze content contextually, using neural networks to identify relationships and patterns within data. These systems automate field mapping, recognize historical matching patterns, and adapt to changes in data structures. Tasks that once took weeks can now be completed in hours, significantly enhancing operational efficiency. The precision offered by AI reduces integration errors and ensures that data flows seamlessly between systems, enabling more accurate analysis and decision-making.
Anomaly Detection for Enhanced Data Integrity
Ensuring the integrity of data is one of the biggest challenges in large-scale cloud environments. Traditional rule-based methods of anomaly detection are often reactive and prone to false positives, leading to inefficiencies. AI, however, excels in proactive anomaly detection by leveraging machine learning models that process billions of data points daily with nearly 100% accuracy.
These AI systems predict potential issues, assign quality scores, and cleanse data automatically. Advanced pattern recognition and real-time error correction mechanisms further reduce false positives by 85%, ensuring that only accurate and reliable data is processed. This capability not only improves data quality but also boosts confidence in the insights derived from it, enhancing operational outcomes for businesses across industries.
Real-Time Data Integration: The Speed Revolution
As the volume of streaming data continues to grow, real-time integration has become a cornerstone of modern data engineering. AI-enabled systems optimize stream processing, enabling organizations to handle hundreds of thousands of events per second with latencies under one millisecond.
Dynamic partitioning, automated scaling, and real-time data quality validation are key components of these systems, ensuring seamless and efficient integration. By reducing integration errors by 45% and improving processing efficiency by 60%, real-time AI solutions empower businesses to respond swiftly to market demands and gain a competitive edge.
Balancing Challenges and Benefits
While the advantages of AI integration are undeniable, the journey is not without its challenges. Legacy systems often struggle to accommodate AI-driven solutions, and regulatory compliance can add layers of complexity. Initial implementation costs and resource requirements for training AI models can also deter adoption. However, the benefits—enhanced efficiency, significant cost savings, and improved data quality—far outweigh these challenges. Organizations that successfully implement AI-driven systems report up to a 45% reduction in operational costs and a 67% decrease in data processing times, demonstrating the long-term value of AI integration.
To conclude, Venkata Krishna Reddy Kovvuri underscores the transformative power of AI in data engineering and cloud integration. Through innovations such as automated pipelines, intelligent schema matching, and real-time integration, AI is setting a new standard for scalability, precision, and efficiency. Despite challenges, the future of AI-driven data engineering holds immense promise, offering unprecedented opportunities for organizations to unlock the full potential of their data.
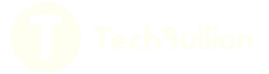