Mrs. Sukanya Konatam is an IT professional boasting an extensive career of over 18 years, enriched by five years dedicated to specializing in AI and AI Governance. Her expertise spans a comprehensive range of IT disciplines, underscored by a profound depth of knowledge in the governance of artificial intelligence. This unique combination of experience positions her as a leading figure in the field, adept at navigating the intricacies of AI technology with a strategic and ethical approach. She has implemented data centric solutions for several industries including banking & financials, telecom, health care, automobile, criminal justice and many more.
Currently, Sukanya is leading Enterprise Data Governance Modernization efforts at Vialto Partners with multiple teams of data engineers, data scientists & analysts. With her proven record of strategic thinking and Solutions Architecture Design, she is particularly responsible to help create a 360-degree view for the system, data security, master data management, enrich data sets, build modern dashboards, automate manual data processes. She defines indicators of performance and quality metrics and ensures compliance with data, defines roles and responsibilities related to data governance and ensures clear accountability. She facilitates the development and implementation of data quality standards, data protection standards and adoption requirements across Vialto Partners.
Prior to her role at Vialto Partners, Sukanya was leading data science, data analytics, automation, and administration teams at EFG Corporate Finance that supported each line of business, CFO and the operating committee with Financial Reporting, Budget & Forecasting automation, and Strategic Oversight. Sukanya helped create periodic KPI Metrics reports to the management and it helped identify new business opportunities pertaining to the use of information assets to achieve efficiency and effectiveness by representing data as a strategic business asset at the senior management table for critical decision making to gauge business critical initiatives, objectives, or goals. She was responsible for rolling out an enterprise-wide data governance framework, with a focus on improvement of data quality and the protection of sensitive data through modifications to organization behavior policies and standards, principles, governance metrics, processes, related tools, and data architecture.
Can you explain what AI Governance models are, key principles for ensuring Trustworthy AI and why they are important in today’s digital age?
AI Governance models refer to frameworks and guidelines designed to ensure that the development, deployment, and use of Artificial Intelligence (AI) technologies are ethical, transparent, accountable, and align with broader societal values and norms. These models aim to manage the risks associated with AI applications, ensuring they contribute positively to society while mitigating potential harms. As AI technologies become more integrated into various aspects of daily life and critical sectors, the need for robust AI governance becomes increasingly important.
Artificial Intelligence (AI) is rapidly transforming various aspects of our lives, from driverless cars to healthcare solutions. As this dual-use technology continues to advance, it brings both benefits and challenges that cannot be ignored by governments, private sectors, or individuals.
Several key principles are commonly cited as foundational for ensuring Trustworthy AI. These principles help guide the ethical development and application of AI technologies:
- Transparency: AI systems should be transparent, with clear explanations of how and why they make decisions. This includes understandable documentation of the AI’s design, development process, and decision-making criteria.
- Fairness: AI applications should be designed to avoid bias and ensure fairness, treating all users without discrimination. This involves actively identifying and mitigating biases in data, algorithms, and their outcomes.
- Accountability: There should be clear mechanisms in place for holding developers, deployers, and users of AI systems accountable for their outcomes. This includes establishing clear responsibilities and legal obligations.
- Privacy and Data Protection: AI systems must respect privacy rights and data protection laws, ensuring that personal data is used appropriately, securely, and with the consent of individuals.
- Safety and Security: AI technologies should be safe and secure, with safeguards against malicious use, cybersecurity threats, and unintended harmful consequences.
- Sustainability: AI should be developed with consideration for environmental impact and sustainability, promoting green AI initiatives to minimize carbon footprints and resource usage.
- Social and Environmental Well-being: AI developments should consider their impact on society and the environment, aiming to contribute positively to societal challenges and promoting human rights and dignity.
In today’s digital age, AI Governance and the principles of Trustworthy AI are crucial for several reasons:
- Ethical Considerations: As AI technologies play a more significant role in decision-making, it’s essential to ensure these decisions are made ethically and align with societal values.
- Preventing Harm: Proper governance can help prevent or mitigate potential harms caused by AI, such as discrimination, privacy breaches, or unintended consequences.
- Building Trust: Trustworthy AI is essential for public acceptance and confidence in AI technologies. When people trust that AI systems are fair, transparent, and ethical, they are more likely to support and adopt these technologies.
- Regulatory Compliance: Governments around the world are beginning to introduce regulations for AI, so adhering to AI Governance models is crucial for legal compliance and avoiding penalties.
- Future-proofing: Establishing robust AI governance frameworks ensures that AI technologies are developed with a long-term perspective, ready to adapt to future challenges and technological advancements.
In summary, AI Governance models and the principles of Trustworthy AI are foundational to ensuring that AI technologies benefit humanity while minimizing risks and ethical concerns, which is increasingly important as these technologies become pervasive in our lives.
Could you give us an overview of the AI market, the trends shaping this industry and what trends to look out for?
The AI market is a rapidly evolving landscape, marked by significant technological advancements, increased adoption across various industries, and an expanding range of applications. It encompasses everything from machine learning, natural language processing, and robotics to computer vision and deep learning technologies. The industry’s growth is propelled by the increasing availability of big data, advancements in algorithmic developments, and improvements in computing power and storage.
Overview of the AI Market
- Size and Growth: The global AI market has been experiencing robust growth, with projections suggesting continued expansion at a compound annual growth rate (CAGR) of over 20% in the coming years. This growth is driven by the escalating demand for AI technologies in healthcare, finance, retail, automotive, and more.
- Investment: Venture capital investment in AI startups continues to surge, reflecting confidence in the market’s future. Moreover, governments and private sector entities are significantly investing in AI research and development.
- Regional Hotspots: While the United States and China are leading the AI race, Europe and other parts of Asia are also becoming key players, with substantial investments in AI research, development, and deployment.
Key Trends Shaping the AI Industry
- AI in Healthcare: AI applications in healthcare, such as diagnostic tools, personalized medicine, and robotic surgery, are growing. The COVID-19 pandemic has further accelerated the adoption of AI in this sector.
- Ethical and Responsible AI: There is a growing emphasis on ethical AI development, focusing on fairness, transparency, and accountability. Governments and organizations are establishing guidelines and frameworks to govern AI use.
- AI and Automation: Automation, powered by AI, is transforming industries by enhancing efficiency, productivity, and innovation. This includes everything from manufacturing processes to administrative tasks.
- Advancements in Natural Language Processing (NLP): Progress in NLP is making it easier for machines to understand and generate human language, improving interactions between humans and AI systems.
- Edge AI: The rise of edge computing has led to the development of Edge AI, where AI algorithms are processed on local devices rather than in a centralized cloud-based system. This trend is crucial for real-time applications, such as in autonomous vehicles and IoT devices.
- AI in Cybersecurity: As cyber threats become more sophisticated, AI and machine learning are increasingly being used to detect, prevent, and respond to security breaches more efficiently.
Trends to Watch Out For
- Generative AI: Technologies like GPT (Generative Pre-trained Transformer) and generative adversarial networks (GANs) are advancing rapidly, offering new capabilities in content creation, data synthesis, and more.
- AI in Environmental Solutions: AI is playing a crucial role in addressing environmental challenges, from climate modeling and monitoring biodiversity to optimizing energy consumption.
- Quantum Computing and AI: The integration of quantum computing and AI has the potential to solve complex problems much faster than current technologies, opening up new frontiers in research and application.
- AI Governance and Regulation: As the impact of AI grows, so does the focus on governance and regulation to ensure safe, ethical, and equitable use of AI technologies.
The AI market’s trajectory is characterized by continual innovation, presenting both opportunities and challenges. Staying informed about these trends is crucial for businesses, policymakers, and technology enthusiasts to navigate the future of AI effectively.
On the topic of AI Governance, could you give us more insight into how AI Governance ensures safety in the implementation of AI technologies?
AI Governance plays a critical role in ensuring the safety of AI technologies throughout their lifecycle, from design and development to deployment and operation. It provides a structured framework to address the ethical, legal, and technical challenges that arise with AI applications. Here’s how AI Governance ensures safety in the implementation of AI technologies:
1. Ethical Design and Development
- Embedding Ethical Principles: AI Governance frameworks often start with the integration of ethical principles into the design and development process. This includes ensuring fairness, accountability, transparency, and respect for user privacy.
- Risk Assessment: Early and continuous assessments of potential risks associated with AI systems help identify and mitigate possible harms. This includes evaluating the impact on individuals, society, and the environment.
2. Regulatory Compliance and Standards
- Adhering to Regulations: Compliance with existing laws and regulations is a key aspect of AI Governance. This includes data protection laws (such as GDPR in Europe), industry-specific regulations, and emerging AI-specific legislation.
- Following Standards: International standards and guidelines, such as those proposed by IEEE or ISO, provide best practices for AI safety, quality management, and data handling.
3. Transparency and Explain ability
- Understanding AI Decisions: Governance models emphasize the importance of making AI systems understandable to users and stakeholders. This involves creating mechanisms for explaining AI decisions in accessible terms, which is crucial for trust and safety.
- Documenting Processes: Maintaining comprehensive documentation on AI systems’ development processes, data sources, and decision-making criteria helps ensure accountability and facilitates oversight.
4. Data Governance
- Secure and Responsible Data Use: Effective data governance ensures that the data used to train and operate AI systems is handled securely, ethically, and in compliance with privacy laws. This includes ensuring data accuracy, integrity, and protecting sensitive information.
- Bias Mitigation: Part of data governance involves identifying and mitigating biases in datasets to prevent discriminatory outcomes, enhancing the fairness and safety of AI applications.
5. Ongoing Monitoring and Evaluation
- Continuous Improvement: AI systems are subject to continuous monitoring to detect and address any issues promptly. This includes performance evaluation, safety monitoring, and the impact assessment of AI applications in real-world settings.
- Feedback Loops: Implementing feedback mechanisms allows for the ongoing improvement of AI systems, ensuring that they remain safe, effective, and aligned with ethical standards over time.
6. Stakeholder Engagement
- Inclusive Dialogue: Engaging a wide range of stakeholders, including ethicists, legal experts, technologists, and the public, ensures diverse perspectives are considered in AI governance. This collaborative approach helps identify potential safety issues and societal concerns.
- Public Trust: Transparent engagement with the public and clear communication about how AI systems are governed, their benefits, and their risks help build public trust in AI technologies.
7. Preparedness and Crisis Management
- Incident Response Plans: AI Governance frameworks include plans for responding to AI-related incidents, ensuring that any safety issues can be quickly and effectively addressed.
- Ethical Exit Strategies: Plans for decommissioning or modifying AI systems that present insurmountable safety risks or ethical concerns are also a component of comprehensive AI governance.
In summary, AI Governance ensures the safety of AI technologies by integrating ethical principles, adhering to legal standards, ensuring transparency and accountability, managing data responsibly, and engaging with stakeholders. These measures collectively help mitigate risks and enhance the trustworthiness of AI applications.
To illustrate the principles and impact of AI Governance in practice, here are three examples of its implementation across different sectors:
1. Healthcare: AI-Powered Diagnostic Tools
In healthcare, AI governance ensures the safe and ethical use of AI-powered diagnostic tools. For example, an AI system developed to diagnose diseases from medical imaging must undergo rigorous validation and testing to ensure accuracy and reliability. Governance frameworks mandate:
- Ethical Data Use: Ensuring patient data privacy and security while training AI models.
- Bias Mitigation: Actively working to remove biases in training data that could lead to unequal treatment of different demographic groups.
- Transparent Decision-Making: Providing clear explanations for diagnostic recommendations, allowing healthcare professionals to understand and trust AI insights.
- Continuous Monitoring: Regularly assessing the tool’s performance in real-world conditions to quickly address any safety or effectiveness issues.
2. Finance: AI in Fraud Detection Systems
In the financial sector, AI governance plays a crucial role in fraud detection systems, ensuring they operate effectively without unjustly penalizing legitimate transactions or customers. Implementation includes:
- Regulatory Compliance: Adhering to financial regulations and privacy laws, ensuring the AI system complies with legal standards for data handling and consumer protection.
- Explainable AI (XAI): Implementing XAI practices to make the decision-making process of the AI system transparent, so that users and regulators can understand how decisions are made.
- Real-Time Monitoring: Establishing mechanisms for the real-time monitoring of AI performance to quickly adapt to emerging fraud patterns and minimize false positives and negatives.
- Stakeholder Engagement: Engaging with customers to inform them about how AI is used in fraud detection and how it impacts their transactions, enhancing trust and cooperation.
3. Smart Cities: AI for Traffic Management Systems
AI governance in smart cities ensures that AI-driven traffic management systems enhance urban mobility safely and equitably. An example implementation might involve:
- Public Safety and Security: Ensuring the AI system prioritizes public safety, reducing the risk of accidents and efficiently managing emergency response.
- Fairness and Accessibility: Guaranteeing the system does not disadvantage any group of citizens, ensuring equitable improvements in traffic flow across different city areas.
- Environmental Consideration: Incorporating sustainable practices, such as reducing emissions through optimized traffic flows and promoting public transport.
- Community Involvement: Including community feedback in the development and iterative improvement of the system to ensure it meets the diverse needs of city residents.
Each of these examples demonstrates how AI Governance frameworks guide the development, deployment, and operation of AI systems across various sectors. By adhering to principles of ethics, legality, transparency, and societal well-being, AI technologies can be implemented safely and effectively, maximizing their benefits while minimizing risks.
Can you explain how fairness is maintained through AI Governance models, in what ways does AI Governance promote fairness and prevent bias in decision-making processes?
Fairness through AI Governance involves implementing strategies and practices that ensure AI systems do not perpetuate or amplify biases, discriminate against individuals or groups, and provide equitable outcomes for all users. AI Governance promotes fairness and prevents bias in several key ways:
1. Bias Detection and Mitigation
AI Governance models include processes for the identification and mitigation of biases in AI systems. This involves analyzing training datasets for historical biases and ensuring that the data representation is diverse and inclusive. For example, an AI model used in hiring processes must be trained on data representing a diverse range of candidates across genders, ethnicities, and backgrounds to prevent it from developing biases that could favor one group over others.
2. Diverse and Inclusive Development Teams
Ensuring that AI systems are developed by diverse teams can help reduce the risk of unconscious biases being built into these systems. Diverse teams bring a variety of perspectives that can identify and address potential fairness issues more effectively. For instance, a team developing a facial recognition technology would benefit from diverse perspectives to ensure the technology accurately recognizes faces from various racial and ethnic backgrounds, thus avoiding biases seen in earlier technologies that performed poorly with non-white individuals.
3. Transparent Decision-Making
AI Governance promotes transparency in how decisions are made by AI systems, making it easier to identify and correct any biases. This involves documenting and explaining the criteria and algorithms that AI systems use to make decisions. In the context of loan applications, for instance, an AI system should clearly explain the factors it considers in approving or rejecting applications. This transparency allows applicants to understand decisions and provides an opportunity to correct any inaccuracies or biases in the decision-making process.
4. Ethical and Legal Frameworks
AI Governance involves adhering to ethical and legal frameworks that emphasize fairness. This includes compliance with laws and regulations designed to prevent discrimination, such as the Equal Credit Opportunity Act in the U.S., which requires fair and equitable treatment of all individuals in credit decisions. AI systems used in credit scoring must, therefore, be designed and monitored to ensure they comply with these legal standards, actively preventing discriminatory outcomes.
5. Regular Auditing and Monitoring
Ongoing auditing and monitoring of AI systems are crucial for maintaining fairness over time. This involves regularly reviewing the outcomes of AI decisions to identify any patterns of bias or discrimination. For example, an AI system used in healthcare diagnostics could be regularly audited to ensure that it provides accurate and fair recommendations for patients of all demographics, adjusting the system as needed to correct any disparities in outcomes.
Example: AI in Recruitment
A practical example of promoting fairness through AI Governance can be seen in the use of AI for recruitment and hiring processes. Bias in hiring can lead to unequal opportunities and reinforce systemic inequalities. To address this, AI systems used in screening resumes can be governed by frameworks that:
- Ensure Diversity in Training Data: The AI is trained on a diverse set of resumes representing candidates from varied backgrounds, experiences, and demographic groups.
- Implement Bias Checks: The system undergoes regular checks for biases, where its recommendations are reviewed to identify any patterns of preference or exclusion based on irrelevant factors like name, gender, or ethnicity.
- Provide Decision Transparency: The AI system offers explanations for its screening decisions, allowing recruiters to understand why a candidate was recommended or not, and ensuring that the final hiring decision is made with human oversight.
Through these governance measures, AI in recruitment can help create a more fair and inclusive hiring process, reducing biases and promoting equality in employment opportunities
How does AI Governance respect and uphold human rights when it comes to deploying AI solutions, how can AI Governance models ensure respect for human rights when utilizing data analytics tools?
AI Governance plays a crucial role in ensuring that the deployment of AI solutions respects and upholds human rights by establishing frameworks that prioritize ethical considerations, transparency, accountability, and fairness. These governance models incorporate principles that safeguard privacy, freedom of expression, non-discrimination, and other fundamental rights in the context of AI development and use. Here’s how AI Governance models can ensure respect for human rights when utilizing data analytics tools, with examples to illustrate these practices:
1. Privacy and Data Protection
AI Governance models enforce strict data protection policies to ensure the privacy of individuals. This includes implementing measures such as data anonymization, encryption, and secure data storage practices. Additionally, governance frameworks require that data collection and processing activities are transparent and have explicit consent from individuals, adhering to regulations such as the General Data Protection Regulation (GDPR) in Europe.
- Example: A healthcare organization using AI to analyze patient data for personalized treatment plans must ensure that patient data is anonymized to protect privacy. It must also obtain consent from patients to use their data, providing information on how the data will be used and ensuring it is used solely for the intended purpose of improving healthcare outcomes.
2. Non-discrimination and Fairness
AI Governance models incorporate checks and balances to identify and mitigate biases in AI algorithms and training datasets, ensuring AI solutions do not discriminate against any individual or group. This involves regular audits of AI systems for biased outcomes, the implementation of bias-correction techniques, and the promotion of diversity in AI development teams to reduce unconscious biases.
- Example: An AI-powered job application screening tool must be regularly audited to ensure it does not unfairly discriminate against candidates based on gender, ethnicity, age, or other irrelevant criteria. If biases are detected, the tool must be adjusted or retrained with more diverse datasets to eliminate discriminatory biases.
3. Transparency and Accountability
AI Governance models emphasize the importance of transparency in AI operations, ensuring that individuals understand how their data is used and how AI-driven decisions are made. This includes providing accessible explanations of AI decision-making processes and maintaining clear accountability structures for AI actions, so there is always a human entity responsible for AI-driven decisions.
- Example: A financial institution using AI for credit scoring must transparently disclose how the AI model makes decisions, including the factors it considers. This allows individuals to challenge and seek redress for decisions they believe are unfair or incorrect, ensuring accountability.
4. Right to Freedom of Expression
In the context of AI governance, safeguarding the right to freedom of expression involves ensuring that AI tools, such as content moderation systems on social platforms, operate in a way that respects this right. Governance frameworks require that these systems are designed to minimize overreach, preventing unjust suppression of speech while still effectively identifying and mitigating harmful content.
- Example: Social media platforms employing AI for content moderation must balance between removing harmful content and upholding freedom of expression. This requires transparent criteria for content removal, avenues for appeal against content decisions, and regular oversight to ensure the AI does not unjustly censor legitimate expressions.
5. Participatory Governance
AI Governance encourages the involvement of a broad spectrum of stakeholders, including civil society, in the development, deployment, and monitoring of AI systems. This participatory approach ensures that diverse perspectives are considered, particularly those of marginalized or vulnerable groups, in shaping AI policies and practices.
- Example: A city planning to deploy AI surveillance systems for public safety must engage with community members, civil rights groups, and other stakeholders to discuss and address concerns related to privacy and surveillance. This ensures that the deployment of such technologies respects the rights and freedoms of the community.
By incorporating these principles, AI Governance models play a vital role in ensuring that AI technologies respect and uphold human rights. These models provide a structured approach to navigating the complex ethical landscape of AI application, ensuring that technologies serve humanity’s best interests while safeguarding individual rights and freedoms.
What role do ethical considerations play in establishing effective AI Governance models, how can organizations effectively implement and enforce AI Governance policies to maintain ethical standards in their use of artificial intelligence technology?
Ethical considerations are at the core of establishing effective AI Governance models, guiding organizations in the responsible development, deployment, and use of artificial intelligence technology. These considerations ensure that AI systems align with societal values, respect individual rights, and contribute positively to humanity. Here’s how ethical considerations influence AI Governance and ways organizations can implement and enforce governance policies to maintain these standards, along with examples:
Role of Ethical Considerations in AI Governance
Principle-Based Frameworks: Ethical principles such as fairness, transparency, accountability, privacy, and safety form the foundation of AI governance models. These principles guide the design and deployment of AI systems to avoid harm, discrimination, and ensure the well-being of individuals and society.
Stakeholder Engagement: Involving a diverse group of stakeholders in the AI governance process ensures that multiple perspectives are considered, including those of potentially affected communities, thus embedding ethical considerations into decision-making processes.
Human-Centric Approach: Ethical AI Governance focuses on the impact of AI on human rights and values, ensuring that technologies enhance, rather than undermine, human dignity, autonomy, and rights.
Implementing and Enforcing AI Governance Policies
Establish Clear Policies and Procedures: Organizations should develop clear AI governance policies that incorporate ethical principles, detailing the processes for design, development, deployment, and monitoring of AI systems. These policies should include mechanisms for ethical risk assessment and mitigation.
- Example: A financial institution using AI for credit scoring should establish policies that require bias detection and mitigation efforts, ensuring that the AI system does not unfairly disadvantage any group of applicants based on race, gender, or socioeconomic status.
Ethics and Compliance Training: Employees and developers involved in AI projects should undergo training on the organization’s AI ethics and governance policies. This includes understanding the ethical implications of their work and how to address ethical dilemmas.
- Example: A tech company might provide mandatory training for its AI development teams on recognizing and mitigating biases in AI models, emphasizing the importance of fairness and transparency in AI applications.
Ethical Review Boards: Establishing an AI ethics review board within the organization can provide oversight and guidance on AI projects, ensuring they align with ethical standards and governance policies. The board can review proposals for new AI projects, monitor ongoing projects for compliance, and advise on ethical issues.
- Example: A healthcare organization deploying AI for patient diagnosis can have an ethics review board that includes ethicists, clinicians, patient advocates, and AI experts to review and approve AI projects, ensuring they respect patient rights and privacy.
Regular Auditing and Monitoring: Continuous monitoring and periodic auditing of AI systems help ensure they operate as intended and adhere to ethical standards over time. This can identify and correct any issues related to bias, fairness, or unintended consequences.
- Example: A retail company using AI for personalized marketing should regularly audit its algorithms to ensure they do not inadvertently discriminate or invade the privacy of consumers. This could involve checking for biases in the data that might lead to exclusionary practices.
Transparency and Reporting: Organizations should be transparent about their use of AI technologies, including the governance frameworks in place. Public reporting on AI governance efforts, including challenges and how they were addressed, builds trust and accountability.
- Example: A public sector agency implementing AI for social services could publish an annual report detailing its AI governance practices, including how it has addressed ethical concerns and the impact of AI on service delivery outcomes.
By integrating ethical considerations into the fabric of AI governance models and implementing robust policies and procedures, organizations can navigate the complex ethical landscape of AI technology. This ensures that their use of AI not only drives innovation and efficiency but also respects human rights and contributes to the common good.
What steps can companies take to ensure their AI deliverables meet these governance standards and What role do Data Scientists play in upholding the principles of Trustworthy AI within an organization’s governance framework?
Companies can adopt several strategic steps to ensure their AI deliverables meet governance standards, focusing on embedding ethical considerations, transparency, fairness, and accountability within their AI systems. Data scientists play a critical role in this process, as they are often at the forefront of developing, deploying, and monitoring AI applications. Here’s how companies can align their AI deliverables with governance standards, including the pivotal role of data scientists, illustrated with examples:
Steps for Companies
Develop a Comprehensive AI Governance Framework: Establish clear guidelines that encompass ethical principles, legal compliance, and technical standards for AI development and use.
- Example: A multinational corporation could create a centralized AI ethics board that develops and oversees the implementation of AI governance policies across all business units, ensuring consistent adherence to ethical standards.
Implement Ethics and Bias Training: Provide ongoing education for employees, especially those in technical roles, about the ethical implications of AI technologies and how to identify and mitigate biases.
- Example: A tech startup might offer workshops and training sessions for its data scientists and engineers on ethical AI development, covering topics like fairness in machine learning models and privacy-preserving data practices.
Conduct Regular AI Audits: Perform periodic internal and external audits of AI systems to assess compliance with governance standards, identify risks, and implement necessary improvements.
- Example: An online retail company could conduct bi-annual audits of its AI-driven recommendation system to ensure it doesn’t inadvertently promote discriminatory practices or expose sensitive customer data.
Engage in Transparent Reporting: Publicly disclose information about AI projects, including their objectives, methodologies, and impact assessments, to promote transparency and accountability.
- Example: A financial services firm might release an annual transparency report detailing its use of AI in credit scoring, including efforts to eliminate bias and ensure equitable treatment of all applicants.
Role of Data Scientists
Ethical AI Design and Development: Data scientists are responsible for incorporating ethical considerations into the AI development process, selecting unbiased datasets, and designing algorithms that prioritize fairness and transparency.
- Example: In developing a job screening tool, data scientists ensure the algorithm doesn’t unfairly penalize applicants based on gender, ethnicity, or age by carefully curating training data and implementing fairness-enhancing techniques.
Bias Detection and Mitigation: They play a crucial role in identifying and addressing biases in AI models. This includes analyzing data sources, testing algorithms for discriminatory outcomes, and refining models to reduce bias.
- Example: Data scientists at a healthcare company developing an AI tool for diagnosing diseases might use techniques like adversarial testing to uncover and correct biases that could lead to unequal treatment of patients from different demographic backgrounds.
Monitoring and Evaluation: Continuous monitoring of AI systems to ensure they perform as intended and adhere to ethical standards. Data scientists analyze performance metrics, user feedback, and impact assessments to identify and rectify any issues.
- Example: In a customer service chatbot application, data scientists monitor interactions for instances of inappropriate responses or failures to respect user privacy, adjusting the AI models as needed to address these concerns.
Promoting Transparency and Explainability: Data scientists are tasked with making AI systems understandable to non-technical stakeholders. This involves developing clear explanations of how AI models make decisions and the factors influencing those decisions.
- Example: For an AI-powered investment platform, data scientists create a feature that explains to users in simple terms how the AI recommends certain investments, including the risk factors and historical data considered.
By taking these steps and leveraging the expertise of data scientists, companies can ensure their AI deliverables meet governance standards and uphold the principles of Trustworthy AI. This not only enhances the ethical integrity of their AI applications but also builds trust with users, regulators, and the wider community.
Can you provide examples of successful applications of comprehensive AI governance frameworks in real-world scenarios, any use cases and success stories to share with us?
Implementing comprehensive AI governance frameworks successfully requires thoughtful integration of ethical principles, technical standards, and regulatory compliance into the lifecycle of AI systems. While many organizations are still navigating the complexities of effective AI governance, there are notable examples and use cases where governance frameworks have led to positive outcomes, enhancing trust, fairness, and accountability. Here are a few examples:
1. IBM’s AI Ethics Board
Use Case: IBM established an AI Ethics Board, tasked with overseeing the ethical development and deployment of AI technologies across the company. This board is responsible for setting out IBM’s principles for Trust and Transparency in AI, guiding research, development, and commercialization efforts to ensure they align with the company’s ethical standards.
Success Story: The board’s work has led to the development of tools like AI Fairness 360, Watson OpenScale, and FactSheets 360, which help users understand, explain, and mitigate bias in AI models. By prioritizing ethical considerations and transparency, IBM has become a leader in developing trustworthy AI solutions.
2. DeepMind’s AI Safety and Ethics Research
Use Case: DeepMind, a leading AI research company, has invested significantly in AI safety and ethics research to ensure its advancements in AI, especially in areas like healthcare and autonomous systems, are developed responsibly.
Success Story: DeepMind’s collaboration with Moorfields Eye Hospital in London to use AI for diagnosing eye diseases from scans is a prime example. The project emphasized patient safety, data protection, and transparent research methodologies, leading to AI systems that can potentially transform eye care by providing quick, accurate diagnoses while ensuring the ethical use of patient data.
3. City of Amsterdam’s Algorithm Register
Use Case: The City of Amsterdam has implemented an Algorithm Register, an innovative governance tool designed to increase transparency around the AI and algorithms used by the city government. This register details the algorithms’ purposes, the data they use, and measures taken to ensure fairness and privacy.
Success Story: This register makes Amsterdam one of the first cities worldwide to provide such a level of transparency in governmental AI use, fostering trust among citizens and promoting responsible AI use that respects citizen rights and privacy.
4. Salesforce’s Ethical Use of AI
Use Case: Salesforce introduced an Office of Ethical and Humane Use of technology, focusing on ensuring that its AI technologies, such as Einstein AI, are developed and used in ways that uphold ethical principles and human rights.
Success Story: Through its comprehensive governance framework, Salesforce has implemented guidelines and processes for ethical decision-making, training for employees on ethical AI use, and tools that help customers use AI in a responsible manner. Their proactive approach in ethical AI governance helps in maintaining trust with users and setting a standard in the industry for responsible AI development.
5. Google’s AI Principles
Use Case: Google has established a set of AI Principles and an internal AI Ethics Review process to guide responsible AI development and use across its products and services.
Success Story: Google’s commitment to these principles has led to the development of Explainable AI tools, which increase the transparency and understandability of AI systems for users. Moreover, Google has withdrawn or modified projects that do not align with its AI principles, demonstrating a commitment to ethical AI development beyond mere rhetoric.
These examples illustrate how comprehensive AI governance frameworks, when successfully applied, can lead to innovative and ethical AI applications that benefit both the organizations and their stakeholders. By prioritizing ethical standards, transparency, and accountability, companies can navigate the complexities of AI development responsibly, paving the way for sustainable and trustworthy AI ecosystems.
How do you see the landscape of Trustworthy AI evolving in the future, and what challenges may arise in its implementation? Do you have more tips for our readers today?
The landscape of Trustworthy AI is poised for significant evolution in the future, driven by rapid technological advancements, increasing societal reliance on AI systems, and growing awareness of the ethical, legal, and social implications of AI. As we move forward, the concept of Trustworthy AI will expand and deepen, incorporating emerging technologies and addressing new challenges. Here’s how this landscape might evolve and some of the challenges it may face:
Evolution of Trustworthy AI
Increased Regulation and Standardization: Governments and international bodies are likely to introduce more comprehensive regulations and standards for AI, aiming to ensure its ethical and safe use. This will drive organizations to adopt more rigorous governance frameworks to comply with these requirements.
Advancements in Explainable AI (XAI): As AI systems become more complex, the demand for transparency and explainability will grow. Advancements in XAI will help stakeholders understand AI decision-making processes better, fostering trust and enabling more informed discussions about AI ethics and governance.
Greater Focus on AI for Social Good: Trustworthy AI will increasingly be leveraged to address global challenges such as healthcare, environmental sustainability, and social inequality. This will necessitate AI governance models that prioritize social impact, fairness, and inclusivity.
Ethics by Design: Ethical considerations will become integral to the AI development process, with ethics by design approaches being adopted widely.
This means ethical principles will be embedded in AI systems from the ground up, rather than being an afterthought.
Challenges in Implementation
Balancing Innovation with Ethical Concerns: As AI technologies evolve, finding the right balance between fostering innovation and ensuring ethical, transparent, and fair AI use will be challenging. Striking this balance without stifling innovation will require nuanced policies and frameworks.
Global Standards vs. Local Contexts: Developing AI governance frameworks that are globally applicable yet flexible enough to adapt to local cultural, ethical, and legal contexts will be a significant challenge, given the diversity of societal norms and values.
Managing Bias and Discrimination: Despite efforts to mitigate bias in AI, the risk of discrimination remains a persistent challenge, especially as AI is applied in more critical areas. Continuous advancements in bias detection and mitigation techniques will be necessary.
Security and Privacy Concerns: As AI systems become more integrated into daily life, ensuring the security of these systems and the privacy of personal data processed by AI will be increasingly challenging. Developing robust security measures and privacy-preserving AI technologies will be crucial.
Tips for Navigating the Future Landscape
Stay Informed: Keep abreast of the latest developments in AI governance, ethical AI research, and regulations to ensure your AI practices remain compliant and ethically sound.
Foster Multi-disciplinary Collaboration: Encourage collaboration between technologists, ethicists, legal experts, and other stakeholders to address the multifaceted challenges of Trustworthy AI.
Invest in Education and Training: Educate your workforce about the importance of ethical AI development and use, and provide training on how to implement Trustworthy AI principles in their work.
Engage with Stakeholders: Regularly engage with users, communities, and other stakeholders to understand their concerns and expectations regarding AI. This feedback can inform more responsible AI development and deployment practices.
Prioritize Transparency: Develop mechanisms to explain how your AI systems work, the decisions they make, and the data they use. Transparency builds trust and facilitates more meaningful discussions about AI’s role in society.
As the landscape of Trustworthy AI continues to evolve, embracing these principles and preparing for the challenges ahead will be essential for organizations looking to harness the power of AI responsibly and ethically.
Please find my publications in Data Governance and Python here:
Data Governance: MDM and RDM (Part 3) – DZone
Data Governance: Data Architecture (Part 2) – DZone
Data Governance, Data Privacy and Security – Pt.1 – DZone
Unpivot Data Using Python – SQLServerCentral
MuleSoft Integration with Azure Key Vault – SQLServerCentral
Please connect with me on LinkedIn Sukanya Konatam | LinkedIn
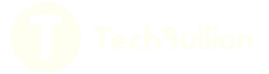