The advent of Artificial Intelligence (AI) has revolutionized numerous industries. It has significantly altered the way organizations function, leading to more efficient processes, accurate decision-making, and groundbreaking innovations. Behind the rise of AI lies the strategic field of AI Engineering, a multidisciplinary domain that involves designing, developing, and maintaining AI systems. These systems are critical for businesses looking to automate tasks, enhance customer experiences, and generate insights from vast amounts of data.
What is AI Engineering?
At its core, AI Engineering focuses on the design and creation of systems that can perform tasks requiring human intelligence. These tasks include natural language understanding, visual perception, speech recognition, decision-making, and more. AI engineers work across various aspects of AI development, from model training and data handling to deploying AI solutions in real-world applications.
The primary goal of AI Engineering is to develop systems capable of learning, reasoning, and adapting to changing conditions. These systems must also be scalable and flexible, allowing for continuous improvement and expansion. Building such systems requires collaboration across various disciplines, including computer science, data science, machine learning (ML), and software engineering.
As AI continues to evolve, the demand for AI Engineers skilled in developing cutting-edge solutions has grown significantly. This demand spans industries, from healthcare and finance to automotive and entertainment, where AI applications are becoming increasingly critical.
Key Components of AI Engineering
AI Engineering is a complex, multifaceted field that integrates several crucial components. Each component plays a vital role in ensuring that AI systems function as intended, delivering results that are both accurate and efficient.
Data Collection and Preprocessing
Data is the lifeblood of AI. Without data, AI systems cannot learn or improve over time. AI engineers are responsible for collecting vast amounts of data, which is then cleaned, labeled, and structured in a format suitable for machine learning algorithms. This step is crucial since the quality of data directly impacts the performance of AI models.
Model Development
Once data has been collected and preprocessed, the next step is to develop a machine learning or deep learning model. This involves selecting an appropriate algorithm, training the model on the dataset, and fine-tuning its parameters to achieve optimal performance. Model development requires a deep understanding of both statistical techniques and computational methods to ensure the system can learn from data efficiently.
Model Evaluation and Testing
Before an AI system can be deployed, it must undergo rigorous testing and evaluation. Engineers assess the performance of the model using various metrics, such as accuracy, precision, recall, and F1 score. This helps identify any issues, such as overfitting or underfitting, that could affect the system’s reliability. Thorough testing ensures that the AI system can handle real-world conditions without compromising accuracy.
Deployment and Integration
Once the model has been thoroughly tested, it is ready for deployment. AI engineers must ensure that the system integrates seamlessly into existing infrastructure, whether it’s for an enterprise solution, a mobile app, or an autonomous vehicle. This stage involves setting up APIs, managing data pipelines, and ensuring that the system can scale as required.
Monitoring and Maintenance
AI systems are not static; they require continuous monitoring and maintenance to ensure long-term success. Engineers track the performance of the system, gather new data, and retrain models to accommodate changes in the environment or data distribution. By doing so, AI systems can continue to improve and adapt over time.
Applications of AI Engineering Across Industries
AI Engineering has paved the way for the development of sophisticated systems across a wide range of industries. These applications are not only enhancing operational efficiency but are also driving innovation in areas that were previously thought to be out of reach for automation and intelligence.
Healthcare
AI-powered systems in healthcare are transforming patient care, diagnostics, and treatment. From AI algorithms that assist in interpreting medical images to robotic surgery systems, AI Engineering is at the forefront of medical advancements. For example, AI can analyze large datasets of patient records to identify patterns and recommend personalized treatment plans. Moreover, AI-based tools can help in early detection of diseases, such as cancer, by analyzing medical images with high accuracy.
Finance
In the financial sector, AI Engineering is enabling more accurate risk assessments, fraud detection, and customer service automation. Machine learning models can analyze vast datasets to identify potential fraud in real time, ensuring faster response times and reduced losses. Additionally, AI systems in finance are increasingly being used to build trading algorithms that predict market trends and make decisions faster than human traders.
Automotive and Transportation
The automotive industry is experiencing a revolution thanks to AI Engineering, particularly in the development of autonomous vehicles. AI systems in autonomous vehicles use data from cameras, sensors, and GPS systems to navigate roads, avoid obstacles, and make driving decisions. Engineers are working to enhance the safety and reliability of these systems, which are poised to become a major part of future transportation networks.
Retail and E-commerce
In retail, AI Engineering is driving personalized shopping experiences and inventory management. Machine learning models analyze customer behavior and preferences to recommend products tailored to individual needs. AI systems also assist in optimizing supply chains, predicting demand, and managing stock levels efficiently. By leveraging AI, retailers can enhance customer satisfaction while reducing operational costs.
Entertainment
AI-powered systems in the entertainment industry are being used for content recommendation, media creation, and even gaming. Streaming services like Netflix and Spotify rely on AI algorithms to recommend content based on users’ preferences. AI is also used in generating realistic graphics for video games and creating virtual characters that interact with players dynamically.
Challenges and Ethical Considerations in AI Engineering
Despite the immense potential of AI systems, AI Engineering comes with its fair share of challenges. These include technical issues, such as the need for large computational resources, and ethical concerns surrounding the use of AI in decision-making processes.
Data Privacy and Security
AI systems rely on vast amounts of data, often including sensitive personal information. Ensuring data privacy and security is one of the primary concerns in AI Engineering. Engineers must design systems that not only comply with regulations but also protect user data from breaches and misuse.
Bias in AI Systems
AI models learn from the data they are trained on, and if that data is biased, the AI system will likely exhibit similar biases. This can lead to unfair treatment of certain groups, especially in applications such as hiring, lending, and law enforcement. Addressing bias in AI is a major focus for engineers, as fairness and ethical responsibility are critical for building trustworthy systems.
Job Displacement
As AI systems automate tasks traditionally performed by humans, concerns over job displacement are rising. While AI Engineering creates new opportunities in areas such as AI development, data science, and system maintenance, it also poses challenges in terms of workforce adaptation. Engineers must balance the drive for innovation with the broader impact of AI on society.
The Future of AI Engineering
AI Engineering is evolving rapidly, and its impact on industries and society is expected to grow significantly in the coming years. Future advancements will likely focus on creating more efficient, transparent, and ethical AI systems. Engineers will continue to refine algorithms, enhance computational power, and address the ethical challenges associated with AI.
Additionally, the rise of explainable AI (XAI) aims to make AI systems more transparent by allowing humans to understand how decisions are made. This is particularly important in areas such as healthcare and finance, where accountability and trust are paramount. As AI Engineering advances, it will undoubtedly shape the future of technology, creating new possibilities for businesses and individuals alike.
Conclusion
AI Engineering plays a pivotal role in building advanced AI systems that are transforming industries and improving daily life. From healthcare and finance to transportation and entertainment, AI systems are solving complex problems and driving innovation. However, these systems also present unique challenges that must be addressed, such as ensuring data privacy, reducing bias, and balancing the societal impacts of automation. As AI continues to evolve, AI engineers will remain at the forefront, shaping a future where AI becomes an integral part of our everyday lives.
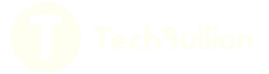