Cybersecurity is undergoing a major transformation with the rise of artificial intelligence. Deepak Gandham, a thought leader in AI-driven security, explores the latest advancements in threat detection and response in his latest research. His work delves into the integration of machine learning architectures, behavioral analytics, and automated response mechanisms, setting the stage for the future of cybersecurity.
The Evolution of AI in Cybersecurity
The rapid increase in cyber threats has necessitated the adoption of more sophisticated security measures. Traditional rule-based systems are struggling to keep up with the complexity and volume of attacks. AI-powered threat detection systems leverage machine learning to analyze vast amounts of data in real time, identifying potential threats with unparalleled accuracy. These systems can detect previously unknown threats within seconds, significantly reducing the time to detection compared to traditional models.
Multi-Tiered Machine Learning Architectures
One of the most notable innovations in AI-driven cybersecurity is the implementation of multi-tiered machine learning architectures. These systems utilize a combination of supervised and unsupervised learning techniques to enhance threat detection. Supervised models can identify known attack patterns with remarkable precision, while unsupervised models detect anomalies that may indicate novel threats. Deep learning networks further enhance security by identifying complex, multi-stage attack scenarios with exceptional accuracy.
Behavioral Analytics: A Game Changer in Threat Detection
AI-driven security frameworks now incorporate behavioral analytics to monitor user and device activity. These systems establish behavioral baselines and detect deviations that may signal a security breach. By analyzing millions of user activities, AI-powered User and Entity Behavior Analytics (UEBA) can detect insider threats within minutes, drastically reducing false positives compared to conventional rule-based systems. Device behavior profiling also plays a crucial role, identifying anomalies in resource utilization and network activity, ensuring compromised systems are detected and quarantined swiftly.
Automated Threat Response: The Future of Cyber Defense
The integration of AI into threat response mechanisms has revolutionized cybersecurity operations. Modern AI-driven response systems can automatically contain threats within milliseconds of detection, significantly mitigating potential damage. These systems employ network isolation, adaptive defense mechanisms, and AI-driven traffic analysis to prevent the lateral movement of attackers. The automation of security protocols not only enhances efficiency but also reduces the burden on security teams, allowing them to focus on strategic threat mitigation.
Performance Optimization and Scalability
A key challenge in cybersecurity is balancing performance with accuracy. AI-driven systems optimize security operations by reducing false positives while maintaining high detection rates. Advanced risk scoring algorithms and contextual validation mechanisms ensure that legitimate user activities are not mistakenly flagged as threats. Furthermore, AI-driven systems process vast amounts of security telemetry data in real time, enabling organizations to maintain robust security postures with minimal latency.
Integration with Enterprise Security Infrastructure
Seamless integration of AI-driven security systems with existing enterprise infrastructures is crucial for widespread adoption. Modern AI security platforms integrate with Security Information and Event Management (SIEM) and Security Orchestration, Automation, and Response (SOAR) systems, ensuring comprehensive threat intelligence and automated incident response. These integrations enhance data fidelity and reduce response times, making AI-driven security solutions a vital component of enterprise cybersecurity strategies.
The Role of Quantum Computing in Cybersecurity
As cyber threats evolve, researchers are exploring the integration of quantum computing with AI-driven security systems. Quantum computing has the potential to revolutionize threat detection, enabling security platforms to process vast datasets at unprecedented speeds. Additionally, quantum-resistant encryption techniques are being developed to counteract the emerging threat of quantum-powered cyberattacks. These advancements will further strengthen the resilience of AI-driven security frameworks.
The Future of AI in Cybersecurity
AI-driven threat detection and response systems have already demonstrated remarkable success in enhancing cybersecurity defenses. The continuous evolution of AI technologies, including explainable AI, transfer learning, and federated learning, will further improve the efficiency and accuracy of security operations. As organizations face increasingly sophisticated cyber threats, the adoption of AI-powered security solutions will be crucial in maintaining robust defense mechanisms.
In conclusion, Deepak Gandham’s research underscores the transformative potential of AI in cybersecurity. With advancements in machine learning, behavioral analytics, and automated response mechanisms, AI-driven security systems are poised to redefine the future of cyber defense.
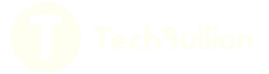