In this modern era, Artificial Intelligence (AI) is transforming software testing, especially in complex system integrations. SrikanthPerla, an expert in AI-driven test automation, highlights how AI boosts efficiency, reduces maintenance efforts, and enhances defect detection, shaping the future of intelligent testing solutions.
Transforming Test Automation with AI
AI integration in test automation has greatly enhanced software testing speed and accuracy. Traditional methods struggle with reliability in dynamic environments, but AI-driven solutions predict failures, adapt to changes, and optimize execution. Organizations using AI-powered frameworks report a 65% boost in defect detection and a 43% reduction in testing cycles, revolutionizing quality assurance.
Smart Test Selection for Efficient Execution
A key innovation in AI-driven testing is smart test selection. By analyzing historical data, system usage, and code changes, AI pinpoints critical test cases, reducing regression testing time by 57% while maintaining a 95.8% defect detection rate. This optimizes resource allocation, ensuring testing focuses on high-impact areas and enhances overall efficiency.
Risk-Based Analysis: Predicting Failures Before They Occur
AI-driven risk-based analysis improves testing by detecting high-risk areas in integrated systems. Machine learning processes thousands of dependencies per minute with 93% accuracy in predicting failures. Organizations using AI report a 72% drop in production incidents and a 63% boost in early defect detection, enabling proactive vulnerability resolution for seamless software performance.
Self-Healing Locators: Reducing Test Maintenance Efforts
Traditional test automation faces challenges with dynamic UI changes, causing frequent failures due to broken locators. AI-powered self-healing mechanisms solve this by dynamically adjusting locators as UI changes occur. With a 92.5% success rate, they enhance test stability and reduce maintenance overhead by 69%, minimizing script updates. This allows teams to focus on strategic testing initiatives rather than constant script maintenance.
Context-Aware Element Identification for Reliable Testing
AI-driven context-aware selection enhances test reliability by accurately identifying stable UI elements across dynamic web applications. By processing thousands of DOM elements per second, AI-powered systems achieve an 89.5% accuracy rate in identifying stable locators, leading to a 75% improvement in test reliability. This capability ensures that automated tests remain robust even in the face of frequent UI updates, reducing false positives and improving overall test stability.
Predictive Error Detection: Enhancing Software Quality
AI-powered predictive error detection revolutionizes bug identification by analyzing vast amounts of test execution data. With a 92.5% accuracy rate in detecting potential failure patterns, AI significantly improves software quality. Organizations utilizing AI-driven pattern recognition have reported a 72% reduction in critical production bugs and a 65% improvement in mean time to resolution (MTTR). By identifying defects early in the development cycle, AI-powered testing minimizes post-deployment issues and enhances overall software reliability.
Anomaly Detection for Improved Security and Stability
Machine learning-based anomaly detection plays a crucial role in identifying security and integrity issues in software applications. AI systems process thousands of data points per second, achieving a 95.2% accuracy rate in detecting anomalies before they lead to security breaches. Studies indicate that AI-powered anomaly detection reduces false positives by 70% and improves early threat detection times by over 222%. These advancements bolster software security by enabling organizations to proactively address vulnerabilities before they are exploited.
Future of AI in Test Automation
The evolution of AI-powered test automation is set to bring even more sophisticated capabilities. Predictive analytics will continue to improve, with AI models expected to achieve 92% accuracy in identifying flaky tests by 2025. Enhanced self-healing capabilities will further reduce script maintenance efforts, while AI-driven test execution optimization is projected to reduce overall testing time by 87%. As AI continues to evolve, organizations can expect more efficient, reliable, and intelligent testing solutions that align with the complexities of modern software development.
In conclusion, AI-driven test automation has revolutionized software quality assurance. From smart test selection and self-healing locators to predictive error and anomaly detection, AI enhances efficiency and reliability. SrikanthPerlaemphasizes AI’s growing role in reducing testing efforts, minimizing defects, and improving software quality. As AI advances, it will continue to shape the future of software testing and system integration, ensuring more robust and efficient testing processes.
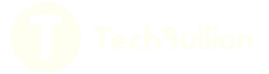