In the modern era, managing the complexities of large-scale infrastructure has become a critical challenge for enterprises. Saikiran Rallabandi, a recognized leader in AI-based systems, highlights the transformative potential of artificial intelligence in capacity planning. His research explores the application of Long Short-Term Memory (LSTM) networks and other advanced AI methodologies, demonstrating how they optimize resource allocation and improve operational efficiency.
Moving Beyond Traditional Forecasting Methods
Traditional capacity planning methods, which rely on static forecasting and historical data trends, often fail to meet the demands of dynamic modern systems. These methods struggle to accommodate fluctuating workloads, resulting in over-provisioning or resource shortages. In contrast, AI-driven systems analyze real-time data and adapt to changing usage patterns. By leveraging LSTM networks, prediction accuracy improves by 47%, while resource over-provisioning reduces by 31%. These advancements mark a significant leap forward in infrastructure management.
The Role of LSTM Networks in Predictive Analytics
LSTM networks are at the core of AI-driven capacity planning. Their ability to process sequential data and capture long-term dependencies makes them ideal for handling irregular workloads. With prediction accuracy reaching 93% for highly variable workloads, LSTM models outperform traditional statistical methods. These networks analyze up to 2,500 data points per minute, enabling precise resource allocation and improved decision-making. By integrating advanced machine learning models, organizations can reduce downtime, optimize costs, and enhance system reliability.
Cross-Layer Monitoring and Resource Optimization
One of the standout features of AI-driven capacity planning is its cross-layer monitoring capability. This approach allows AI systems to simultaneously analyze data across network, application, and infrastructure layers. Such integration results in a 45% improvement in overall resource utilization. For example, network optimization achieves a 37% cost reduction, while application layer improvements save 42% in compute resources. These efficiency gains extend to the infrastructure layer, where energy consumption decreases by 38%, supporting sustainability goals.
AI Integration with Emerging Technologies
The integration of AI with emerging technologies, such as 5G, further enhances its capabilities. Real-time monitoring and edge computing reduce latency, enabling faster response times and more efficient resource allocation. By combining AI with 5G, organizations can deploy smarter systems that adapt dynamically to evolving requirements. These advancements pave the way for innovations like network slicing and autonomous system management, which further optimize operations and improve scalability.
Overcoming Challenges in Implementation
Implementing AI-driven capacity planning systems is not without challenges. Organizations often face issues related to data quality, model training, and legacy system integration. His research emphasizes the importance of phased implementation strategies and adaptive training programs. By addressing skill gaps and ensuring robust data governance, businesses can successfully transition to AI-powered infrastructure management.
The Business Impact of AI-Driven Solutions
The adoption of AI-driven capacity planning yields substantial business benefits. Resource utilization improvements range from 30-40%, with financial analyses indicating a return on investment (ROI) of 312% within the first year. Additionally, incident detection and resolution times improve by 73%, while system reliability increases by 45%. These metrics highlight the tangible impact of AI on operational efficiency and cost-effectiveness.
Security and Privacy Considerations
As AI systems become increasingly integrated into infrastructure management, addressing security and privacy concerns has become a critical priority. He emphasizes the importance of implementing robust security measures such as end-to-end encryption, differential privacy techniques, and secure computation environments to protect sensitive data from potential threats and unauthorized access. These measures not only safeguard data but also enhance the resilience and reliability of AI-driven systems, ensuring they operate seamlessly even in the face of evolving cyber threats. Additionally, adopting advanced security frameworks helps organizations comply with global regulatory standards, maintain user trust, and foster confidence among stakeholders. By proactively addressing these challenges, AI-powered infrastructure management systems can deliver sustainable.
In conclusion, Saikiran Rallabandi underscores the transformative potential of AI-driven capacity planning in revolutionizing infrastructure management. By integrating advanced technologies like LSTM networks, cross-layer monitoring, and 5G, these systems enhance operational efficiency, reduce costs, and support sustainability. As organizations adopt AI-powered solutions, they position themselves for long-term success in a rapidly evolving technological landscape. These innovations not only address current challenges but also lay the groundwork for a more adaptive and efficient future.
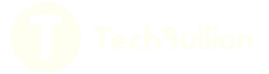