In the current dynamic world, where much of an organization’s decision-making is data-driven, companies from all sectors are faced with enormous challenges as they need to manage huge volumes of data while meeting security, compliance, and efficiency goals. With the ever-increasing number of businesses using insights extracted from data to inform strategic business decisions, the demand for new solutions capable of streamlining data engineering processes is essential. AI bots have rapidly become the driving force behind a new era in data engineering practices, powered by advanced machine learning and Large Language Models (LLM). Sai Arundeep Aetukuri, a pioneering data engineer at the forefront of change, has optimized AI-powered data pipelines, real-time analytics dashboards, and cloud cost management for major industries including healthcare and finance. In this article, we explore how AI bots automate extraction, transformation, and loading processes, enhance governance of information assets, and optimize cloud operation.
Automating ETL Pipelines And Data Workflows With AI Bots
AI bots are changing the game in data pipeline management, automating complex ETL tasks like extraction, transformation, and loading, all done in real-time. AI bots have slashed labor costs by using machine learning algorithms to analyze large datasets, optimize data flow, and make decisions that conventionally required data engineers. Using his knowledge of big data processing and cloud-native architectures, Sai Arundeep Aetukuri objectively designs open-source automated and scalable ETL workflows on AWS, Azure, and GCP, to improve efficiency and minimize manual effort.
AI-Powered Data Governance And Compliance
Data governance tools using AI, play a critical role in regulatory compliance like GDPR, HIPAA, and CCPA. These tools automatically classify, track and monitor sensitive data, minimizing the manual oversight required. In federated learning systems, AI bots guarantee data integrity and prevent tampering or errors across decentralized data sources. An innovative development by Sai Arundeep – “Decentralized Data Integrity Monitor for Federated Learning with Automated Recovery Functions,” strengthens security and reliability, ensuring access to accurate and secure data remains available across all nodes.
AI In Cloud Cost Optimization And Performance Monitoring
Managing cloud resources is tricky and AI bots play an integral role in optimizing costs and performance. AI monitors resource usage to determine where costs can be cut – for example, downscaling unused resources or moving workloads to less-expensive cloud regions. Sai Arundeep Aetukuri is one of the pioneers of AI-based solutions that can automate all these tasks and pave the way to building cost-efficient, high-performing systems in domains like healthcare and finance, where reliability can never be at stake.
Real-World Use Cases Of AI In DataOps, Cloud Engineering
AI bots have many potential applications in DataOps and cloud engineering, and Aetukuri work shows the real-world use cases of such technology.
- In Healthcare: AI bots can process large volumes of patient records and medical data, assisting healthcare providers with accurate information to make effective treatment decisions in a timely manner.
- In Finance Industry: AI bots also aid in the automation of fraud detection and risk management by evaluating transactional data in real time. These bots assist with decision-making and minimize the risk of financial crime by identifying anomalies and flagging potentially suspicious activity in real-time. A notable contribution of Sai in this regard is “Automating Data Labeling and Annotation Pipelines for Large Language Models (LLMs) in the Financial Industry using Machine Learning”- a research work that is crucial in identifying trends in financial datasets using Principal Component Analysis (PCA).
- In Manufacturing: It can be used for predictive maintenance of machinery and production lines. AI models analyze sensor data from machinery predicting when equipment is about to fail thereby reducing downtime. Additionally, these systems are responsible for optimizing cloud infrastructure to process big chunks of real-time data coming from sensors, automatic scaling of resources and performance tuning of the entire system.
- Retail & ECommerce: Supply chain operations and inventory management are optimized through AI-powered systems. Through examining sales data and customer behavior, AI models provide predictive analytics on demand spikes, ensuring sufficient products are in stock without over-provisioning. This helps minimize costs, better manage inventory, and offer optimum customer experience
Integrating AI Automation Into Existing Cloud Infrastructure
For organizations adding AI-powered automation within their current cloud ecosystem, the journey starts with distinguishing the right automatable areas. Data engineering teams should take stock of whether there is still a heavy manual lift, whether it is managing complex ETL pipelines, keeping tabs on cloud costs, or ensuring data governance and consider AI solutions for where these tasks can be done better, faster, or at less cost.
Sai’s experience around scale cloud-native architectures across AWS/Azure/GCP shows exactly how to go about achieving this level of seamless integration. Companies should also train their data scientists and engineers to fine-tune the AI models to creativity shape them for their unique needs and further enhance their organizations. He is revolutionizing data engineering for organizations by simplifying risk management, data storage, and data protection with AI. His creations across the areas of ETL pipeline automation, data governance, cloud cost optimization and secure cloud ops are disruptive in industries such as healthcare and finance.
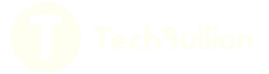