In this modern era, the evolution of artificial intelligence has brought significant advancements, with agentic frameworks at the forefront. Sreeram Reddy Thoom, a researcher specializing in AI development, explores how agentic frameworks revolutionize autonomous decision-making in industries like manufacturing, finance, and healthcare. His insights highlight innovations that enhance efficiency, adaptability, and safety in dynamic environments. These frameworks are paving the way for smarter, more responsive systems that can tackle increasingly complex challenges across industries.
The Core Principles of Agentic Frameworks
Agentic frameworks rely on advanced perception systems and hierarchical decision architectures to enable autonomous decision-making in complex settings. These systems achieve environmental sensing accuracy of 96.2%, processing 845 MB of data per second. Incorporating the Belief-Desire-Intention (BDI) model improves decision-making accuracy by 68.4% and reduces latency by 38.7%, ensuring faster, reliable responses in real-time operations. Additionally, these frameworks enhance adaptability, enabling seamless performance in unpredictable and dynamic environments.
Enhanced Perception and Contextual Awareness
Perception systems are critical to agentic frameworks, enabling precise environmental interactions. The integration of data acquisition layers and contextual processing enhances accuracy, achieving 94.7% pattern recognition and error rates as low as 0.042%. Contextual integration processes 175,000 data points per second, reducing decision latency by 65.7% and improving efficiency in real-time applications. These systems also adapt dynamically to changing conditions, ensuring consistent performance in unpredictable environments.
Decision Architecture: A Multi-Layered Approach
Agentic frameworks operate through a three-layered architecture:
- Strategic Layer: Focuses on long-term planning, achieving an 86.5% goal achievement rate and improving resource allocation efficiency by 38.4%.
- Tactical Layer: Manages medium-term decisions, optimizing resource utilization by 84.9% and reducing production bottlenecks by 43.2%.
- Operational Layer: Handles immediate responses, achieving reaction times of 1.8 milliseconds, reducing delays by 68.5%.
Advanced Learning Mechanisms
Agentic frameworks integrate reinforcement, transfer, and meta-learning to adapt quickly:
- Reinforcement Learning: Optimizes policies 3.2 times faster, reducing decision errors by 62.3%.
- Transfer Learning: Reduces training time by 73.2%, maintaining 93.5% accuracy across domains.
- Meta-Learning: Enhances optimization, cutting skill acquisition time by 76.8%, improving training efficiency.
These learning mechanisms enable rapid adaptation to changing conditions, ensuring performance consistency.
Industry Applications of Agentic Frameworks
Agentic frameworks have demonstrated transformative potential across industries:
- Manufacturing: Boost efficiency by 38.5%, reduce energy use by 24.3%, and cut downtime by 65.7%.
- Finance: Achieve trading latencies of 75 microseconds and improve risk assessments by 38.9%.
- Healthcare: Early detection systems achieve 94.5% accuracy, reducing diagnostic errors by 58.6%.
Addressing Scalability and Safety Challenges
Scalability and safety are critical for implementing agentic frameworks. Advanced resource management supports 35,000 concurrent operations, maintaining response times below 125 milliseconds. Testing frameworks ensure safety with 97.4% edge case coverage, reducing critical failures by 89.3% and enhancing system reliability in complex operational environments.
The Future of Agentic Frameworks
The future of agentic frameworks lies in enhanced autonomy, multi-agent collaboration, and human-AI integration. Next-generation systems aim to process 1.8 million parameters per second, improving adaptability by 142%. Multi-agent frameworks will coordinate up to 7,500 agents with sub-8ms synchronization, enabling seamless operations across complex networks. Human-AI collaboration will enhance task completion rates by 156%, fostering deeper interaction and enabling more intuitive teamwork between intelligent systems and human operators.
Expanding the Reach of Agentic Systems
Agentic frameworks will extend to fields like autonomous transportation, smart cities, and environmental monitoring. Autonomous vehicles powered by these systems will achieve response times below 10 milliseconds, ensuring safety in real-world traffic. Smart cities will benefit from adaptive traffic management, reducing congestion by 47% and improving energy efficiency by 35%. Environmental monitoring will enhance disaster response capabilities, reducing reaction times and mitigating damages.
In conclusion, Sreeram Reddy Thoom emphasizes the transformative potential of agentic frameworks in advancing autonomous decision-making. By integrating advanced perception systems, decision architectures, and adaptive learning mechanisms, these frameworks enhance efficiency, scalability, and safety. As research progresses, agentic frameworks will shape AI’s future, driving innovation while addressing the challenges of complex environments. Their ability to balance autonomy and reliability makes them indispensable to the evolution of intelligent systems.
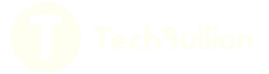