Digital advertising has transformed into an ecosystem of unprecedented scale, with over $1 trillion in global ad revenue. To adapt to this dynamic environment, innovation is paramount. In his recent study, Manish Kumar Keshri explores a new approach to ad ranking that integrates a hierarchical framework to improve ad targeting precision, computational efficiency, and user relevance.
Innovating Ad Ranking through Hierarchical Models
In traditional digital advertising, ad ranking systems focus on evaluating individual ads independently, which can limit their effectiveness. Keshri’s proposed hierarchical ad ranking framework shifts this paradigm by incorporating multiple levels of data: advertisers, campaigns, and individual ads. By recognizing that ads belong to larger campaign structures, which in turn belong to advertisers, the framework can capture richer context that improves prediction accuracy. This approach goes beyond assessing user-ad interaction, integrating the influence of the entire campaign and advertiser relationship with the user.
Enhancing Efficiency and Predictive Power
The hierarchical model stands out for its efficiency. By leveraging these structured levels, the system reduces model size by 94.6% compared to traditional models while maintaining prediction accuracy. This leads to faster processing speeds and a significant reduction in computational resources. The new model also improves cold-start performance—critical for ads that are newly introduced—by 47.3% through transfer learning, which draws on campaign and advertiser-level data to predict the behavior of new ads.
Stability and Relevance: Key Benefits for Advertisers
One of the major strengths of hierarchical ranking is the stability it brings to ad rankings. As Keshri’s framework integrates context from higher levels (advertiser and campaign), ad rankings are less susceptible to the fluctuations caused by sparse individual interactions. This results in more consistent performance, offering advertisers more reliable insights into their ad campaigns. Advertisers also benefit from better targeting and more relevant ad placements, which ultimately enhances user experience while ensuring higher click-through and conversion rates.
Adaptive and Strategic Marketing
Keshri’s hierarchical approach enables adaptive marketing strategies that respond to different audience segments more effectively. Campaigns focused on brand awareness versus direct response can be better differentiated, allowing marketers to adjust their strategies based on the campaign’s broader goals. By considering hierarchical relationships, the system provides a semi-supervised learning model that enhances how ad performance signals are interpreted, particularly in scenarios where data is sparse.
Overcoming Data Sparsity with Hierarchical Structures
Data sparsity remains a significant challenge in large-scale advertising systems. However, Keshri’s hierarchical model helps overcome this obstacle by reducing the data requirements for statistical significance. At the ad-level, where data is often sparse, hierarchical models can achieve statistical relevance with up to 80% less data compared to flat models. This means that even new or infrequently shown ads can perform at a higher level, thanks to the structure provided by campaign and advertiser-level data.
A Shift Towards a More Transparent Advertising Ecosystem
Transparency is a growing concern in digital advertising, particularly with the rise of AI-driven models that are often perceived as “black boxes.” The hierarchical approach addresses this by making the predictions more interpretable. By attributing the ad ranking process to its various hierarchical levels, advertisers can better understand why certain ads are shown or not, fostering greater trust in the system.
Looking Forward: The Future of Digital Ad Ranking
The future of digital advertising lies in the continued evolution of ranking models that adapt to the growing scale and complexity of the industry. His hierarchical framework presents a promising direction for the next generation of ad ranking systems, combining computational efficiency with improved prediction accuracy. As advertisers seek more stable and relevant ad placements, and platforms aim to optimize their revenue models, hierarchical approaches will become a core component of advertising technologies.
In conclusion, Manish Kumar Keshri’s groundbreaking hierarchical ad ranking approach represents a significant step forward in the digital advertising space. By recognizing the importance of ad, campaign, and advertiser relationships, this innovative model offers more stability, relevance, and efficiency, setting the stage for the future of advertising technology. As digital platforms scale and evolve, the adoption of these hierarchical systems will be crucial in balancing user experience, advertiser goals, and computational efficiency.
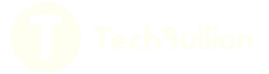