In today’s competitive mobile app landscape, maximizing return on investment (ROI) is crucial for app developers and marketers. With the vast amounts of data generated by app users, app analytics has become a vital tool for understanding user behavior, identifying areas for improvement, and making data-driven decisions. However, with the increasing complexity of app analytics, it’s essential to stay ahead of the curve by adopting advanced analytics mobile app techniques that can help maximize ROI.
In this article, we’ll delve into the world of advanced app analytics techniques, exploring how to leverage data to drive revenue growth, optimize user engagement, and improve overall app performance.
Advanced Segmentation
Segmentation is a fundamental concept in the process of adoption of advanced analytics mobile app. It allows you to group users based on specific characteristics, such as demographics, interests, or behavior. Advanced segmentation takes this concept to the next level by using machine learning algorithms to identify complex patterns and relationships within your user data.
By using advanced segmentation, you can:
- Identify high-value users and target them with personalized marketing campaigns
- Create targeted user personas to inform product development and marketing strategies
- Optimize user retention by identifying and addressing the needs of specific user segments
Predictive Analytics
Predictive analytics uses statistical models to forecast future behavior and predict user outcomes. By leveraging machine learning algorithms and large datasets, predictive analytics can help you:
- Identify users who are likely to churn or uninstall your app
- Predict which users are most likely to convert or make a purchase
- Optimize user engagement by targeting specific users with personalized marketing campaigns
Here’s a more detailed breakdown of how predictive analytics can be used to achieve these goals:
Identify users who are likely to churn or uninstall your app . Predictive analytics can help identify users who are at risk of churning or uninstalling your app by analyzing their behavior and patterns. Here are some ways this can be done:
- User retention modeling: By analyzing historical data on user retention, you can identify patterns and characteristics of users who are likely to stay or leave. This can help you identify early warning signs of churn and take targeted actions to prevent it.
- Feature engineering: By extracting relevant features from user data, such as frequency of usage, average session length, and device type, you can create a predictive model that identifies users who are at risk of churning.
- Machine learning algorithms: By using machine learning algorithms such as decision trees, random forests, or neural networks, you can train a model that predicts the likelihood of churn based on user behavior.
Predict which users are most likely to convert or make a purchase. Predictive analytics can help identify users who are most likely to convert or make a purchase by analyzing their behavior and preferences. Here are some ways this can be done:
- Segmentation: By segmenting users based on their behavior, demographics, or preferences, you can create targeted marketing campaigns that resonate with specific groups.
- Personalization: By using predictive analytics to predict user behavior and preferences, you can create personalized marketing campaigns that are tailored to individual users.
- Dynamic messaging: By using machine learning algorithms to analyze user behavior and preferences in real-time, you can create dynamic messaging that is tailored to individual users and optimized for maximum engagement.
By using predictive analytics in these ways, you can gain a deeper understanding of your users and make data-driven decisions to optimize user engagement and conversion rates.
A/B Testing and Experimentation
A/B testing is a crucial aspect of app analytics, allowing you to test different versions of your app or marketing campaigns to determine which performs better. Advanced A/B testing techniques include:
- Multivariate testing: Testing multiple variables simultaneously to determine their impact on user behavior
- Personalized testing: Testing different versions of your app or marketing campaigns based on individual user behavior
- Real-time testing: Testing different versions of your app or marketing campaigns in real-time to optimize user engagement
Event-Triggered Analytics
Event-triggered analytics allows you to track specific events or actions within your app and trigger analytics events accordingly. This technique can help you:
- Track user behavior in real-time, such as button clicks or form submissions
- Trigger targeted marketing campaigns based on specific user behaviors
- Optimize user engagement by identifying and addressing specific pain points
User Flow Analysis
User flow analysis involves tracking how users navigate through your app, identifying areas where users are getting stuck or losing interest. By analyzing user flow, you can:
- Identify areas where users are dropping off and optimize the user experience accordingly
- Create targeted marketing campaigns to re-engage disinterested users
- Optimize conversion rates by identifying and addressing specific pain points
Attribution Modeling
Attribution modeling involves assigning credit to specific touchpoints or marketing channels for driving conversions or revenue. By using attribution modeling, you can:
- Identify which marketing channels are most effective at driving conversions
- Optimize marketing spend by allocating budget to high-performing channels
- Improve conversion rates by identifying and addressing specific pain points
Real-Time Data Analysis
Real-time data analysis involves tracking and analyzing user behavior in real-time, allowing you to respond quickly to changes in user behavior. By using real-time data analysis, you can:
- Identify and respond to changes in user behavior in real-time
- Optimize user engagement by targeting specific users with personalized marketing campaigns
- Improve conversion rates by identifying and addressing specific pain points
Conclusion
In today’s competitive mobile app landscape, maximizing ROI is crucial for app developers and marketers. By adopting advanced techniques in app analytics, such as advanced segmentation, predictive analytics, A/B testing, event-triggered analytics, user flow analysis, attribution modeling, and real-time data analysis, you can gain a deeper understanding of your users, optimize their experience, and drive revenue growth.
By staying ahead of the curve and leveraging these advanced techniques, you can:
- Increase user engagement and retention
- Improve conversion rates and revenue
- Gain a competitive edge in the market
Remember, the key to maximizing ROI is to continually analyze and optimize your app’s performance using data-driven insights. By staying focused on the needs of your users and leveraging advanced techniques in app analytics, you can achieve long-term success in the competitive mobile app landscape.
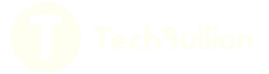