Every time your GPS navigation system asks you to take a detour to avoid traffic, realize that such precise analysis and results come after several hundreds of hours of training. Whenever your Google Lens app accurately identifies an object or a product, understand that thousands after thousands of images have been processed by its AI (Artificial Intelligence) module for exact identification.
What we experience in our everyday lives through AI-powered apps, gadgets and systems are all outputs that the systems have learnt to deliver at superb-precisions. To get here, we spent almost a decade just teaching the systems how to analyze and process such massive volumes of data.
In this tedious journey, what has been the backbone is the process called data annotation. Machines don’t have a brain or mind of their own. They can’t think like their human counterparts. They need to be spoon fed information to make them understand what every single object or element in this world is. It’s only after continuous learning that they get better at processing data and churning out results and insights out of it.
In this post, we will explore the crucial phase of machine learning called data annotation and understand why it is inevitable for AI projects.
Let’s get started.
What Is Data Annotation?
In simple words, data annotation is all about labeling or tagging information in a dataset to let machines understand what they are. The dataset could be an image, an audio file, a video footage or even text. When we tag elements in data, machine learning modules accurately understand what they are going to process and keep that knowledge or information to autonomously process newer information, build on existing knowledge and take timely decisions based on their application or purpose.
For the uninitiated, data annotation is a time-consuming process. It takes countless hours of manual labor to sit and label every single word, pixel, object or second to tag and make the data machine-understandable.
All this is done by data annotation experts and specialists, who exactly know the best ways to annotate data with the most sophisticated tools available.
Different Types of Data Annotation
Like you know, data is of different types. With so many different types of data, it is difficult to have one way to annotate data. That’s why there are different types of data annotation techniques. Let’s look at what they are.
Data Annotation Type |
Purpose |
Semantic Annotation |
The basic technique, where elements are classified into categories with text. It could be marking proper nouns as Names in sentences, cars as vehicles in images and more. This technique is helpful in training chatbots or search engines to help them retrieve the most relevant and useful information to seekers.
|
Text Categorization |
The most abundant form of data lies in its unstructured state. In simple terms, unstructured data is what you will find in images, surveys, reviews, PDFs and more. In text classification, unstructured data is extracted and categorized into relevant classifications for machines to understand. The application of this technique could be as simple as assisting visitors better with website or app navigation.
|
Video And Image Annotation |
This is more complex and time consuming. Mainly used in computer vision, image annotation involves labeling every single pixel, object and shape in an image for machine comprehension. This is done through techniques like bounding boxes, polygonal annotation, cuboid and more.
|
Video annotation is similar to image annotation but differs in the fact that footages are annotated instead of one single image.
|
|
Sentiment Annotation |
This technique is used to tag or label the sentiments and emotions associated with texts, images or videos to better understand human dynamics, attitudes and opinions. For instance, sentiment annotation helps machines differentiate sarcasm from compliment.
|
Data Annotation Use Cases
There are tons of use cases of data annotation. Like we mentioned, any AI or machine learning driven process requires data annotation for precise results. To give you a better idea of what the most prominent use cases are, here’s a list.
- Data annotation helps in delivering better search engine results no matter how vague or complex a search is.
- This process is inevitable in concepts like autonomous cars, where datasets in the form of images, video and from sensors all have to be processed every single second with superlative precision for the most accurate driving decisions. Without the machine knowing what it’s doing (in the absence of data annotation), it will lead to property damage and threat to life.
- It’s helpful in predictive text to help users easily finish sentences without having to type them out.
- It allows stakeholders to make better business decisions thanks to the intelligence that stems from data annotation.
- Data annotation lies at the heart of automation, where predictive and prescriptive analytics modules are deployed.
Wrapping Up
So, this is everything you need to know about data annotation as a beginner. While we’ve simplified the overall gist of the concept, every single aspect we discussed here deserves a content piece of its own. There are several layers and subsets of techniques and approaches that differ based on purpose, application and requirement.
For now, this is ideal to get started with understanding data annotation and its role in machine learning. If you have any questions, comment below.
Description: If you’re a beginner in the AI space, chances are likely that you would have stumbled upon the term data annotation a lot. What does it actually mean? Read to find out.
Social Media Copy: What do data scientists mean when they say they’re annotating data? What is the process all about? Well, here’s an extensive article on data annotation that will help you understand the fundamentals.
Vatsal Ghiya is a serial entrepreneur with more than 20 years of experience in healthcare AI software and services. He is a CEO and co-founder of Shaip, which enables the on-demand scaling of our platform, processes, and people for companies with the most demanding machine learning and artificial intelligence initiatives.
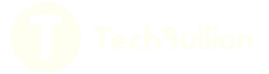