In the ever-expanding world of artificial intelligence, computer vision stands out as a transformative field. From autonomous vehicles and facial recognition to medical diagnostics and agricultural monitoring, computer vision applications are becoming increasingly sophisticated. At the heart of this progress lies a crucial enabler: data annotation services.
These services form the backbone of training datasets, allowing AI systems to “see” and interpret the world in a way that mirrors human perception. Without accurate, consistent, and scalable annotation, even the most advanced algorithms would falter in real-world deployment.
Understanding Data Annotation in Computer Vision
Computer vision systems depend on vast quantities of labeled data to learn how to recognize, classify, and interpret visual information. Data annotation is the process by which this labeling is carried out. It involves tagging images or video frames with metadata that defines the objects, scenes, or actions depicted. Whether it’s bounding boxes around pedestrians, pixel-level segmentation of roadways, or identifying anomalies in medical scans, annotation gives context to raw visual data.
Data annotation services specialize in this critical task. By leveraging trained annotators, advanced tools, and strict quality control protocols, these services ensure that annotated data meets the high standards required for machine learning training.
From Pixels to Predictions: Why Annotation Matters
Imagine an AI model designed to detect tumors in MRI scans. It can only learn what a tumor looks like by analyzing thousands of annotated images where the tumors have been precisely marked by experts. Similarly, an autonomous vehicle learns to distinguish between a traffic sign and a pedestrian by studying labeled examples across varied scenarios.
The accuracy of the model’s predictions is directly linked to the quality of the annotated data it was trained on. Poor annotation leads to unreliable models, which in high-stakes environments like healthcare or autonomous navigation, can result in serious consequences. This is why data annotation services are not just a technical requirement — they are a foundational element of successful AI deployments.
The Human-in-the-Loop Advantage
One of the distinguishing aspects of high-quality data annotation services is the integration of human intelligence into the annotation pipeline. While some annotation can be automated using algorithms, complex scenarios — such as interpreting subtle facial expressions or identifying overlapping objects — still require human judgment.
These services often rely on trained personnel who work within structured frameworks to deliver consistent, accurate results. Through iterative feedback loops, annotators refine their labeling, continuously enhancing dataset quality. The human-in-the-loop model ensures that even nuanced and subjective labeling tasks are performed with care and precision.
Scalability Meets Consistency
Computer vision projects often demand the annotation of millions of images, especially in sectors like retail, agriculture, and automotive technology. Achieving this at scale while maintaining consistency is a monumental challenge.
This is where professional data annotation services demonstrate their strength. With large, distributed teams of annotators and scalable workflows, these services can handle massive data volumes without compromising quality. Advanced annotation platforms, equipped with version control, auditing features, and collaborative tools, further streamline the process.
Moreover, a well-organized service ensures consistency across the dataset. Annotation guidelines, regular quality checks, and reviewer hierarchies are embedded into the workflow to minimize discrepancies and uphold data integrity.
Adaptability Across Domains
Computer vision is not confined to a single industry. It’s used in security surveillance, industrial inspection, agriculture, retail analytics, and beyond. Each domain has unique annotation needs. For instance, annotating plant diseases in agricultural datasets requires different expertise and labeling techniques than annotating products on a store shelf.
Effective data annotation services are adaptable. They tailor their approaches to the specific demands of each project, selecting the right annotation techniques — whether it’s polygon annotation for irregular shapes, keypoint annotation for gesture recognition, or semantic segmentation for complex scenes. Their flexibility ensures that domain-specific requirements are met without compromising accuracy or speed.
Empowering AI Through Ethical Workforce Development
Beyond the technical aspects, some annotation services are also making a meaningful social impact. By employing individuals from underserved communities and investing in their training, these services not only provide dignified employment but also build a workforce capable of contributing to cutting-edge technologies.
This ethical dimension adds value to the annotation process. Annotators are not treated as transient labor but as skilled professionals whose contributions are vital to the success of AI projects. This approach fosters higher engagement, lower turnover, and ultimately, better data outcomes.
Final Thoughts
As the demand for intelligent vision systems continues to rise, so does the need for precise and scalable data annotation services. These services do more than label images — they provide the structured foundation upon which machine learning models are built. Their role is central, not supplementary, in the development of effective computer vision solutions.
By combining human intelligence, technological tools, domain expertise, and ethical employment practices, leading data annotation providers are shaping the future of AI. For any organization embarking on a computer vision initiative, partnering with a trusted annotation service is not just a tactical decision — it’s a strategic investment in long-term success.
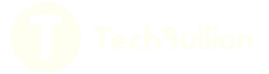