As technology continues to evolve, the integration of Artificial Intelligence (AI) and Machine Learning (ML) is fundamentally transforming the landscape of cloud computing. This transformation is explored in depth in the work of Venkatesh Kata, who sheds light on the power of intelligent cloud automation. With the rise of AI and ML, cloud systems are moving from static, predetermined infrastructures to highly adaptive, self-optimizing systems capable of unprecedented efficiency.
AI and ML: Shaping the Cloud Ecosystem
The traditional model of cloud infrastructure management is increasingly becoming outdated. Previously, cloud systems were managed using rigid, manual scripts that were reactive in nature. These legacy systems lacked the flexibility required to respond dynamically to the ever-changing needs of modern computational environments. With the advent of AI and ML, however, a revolutionary shift is occurring. Cloud management is no longer reactive but anticipatory, as intelligent systems predict and resolve potential issues before they impact operations. This shift allows cloud infrastructure to become smarter, more efficient, and, ultimately, more reliable.
From Static to Dynamic: The Evolution of Cloud Management
AI-driven cloud orchestration systems have revolutionized cloud management by enabling dynamic infrastructure evolution. Using advanced machine learning and neural networks, these systems analyze vast data to identify patterns and autonomously optimize resource allocation, ensuring optimal performance with minimal manual input. Additionally, predictive maintenance allows cloud environments to foresee and prevent failures, enhancing resilience and reducing downtime. This self-optimizing and self-healing capability minimizes human intervention while maintaining efficient and reliable cloud operations.
Intelligent Resource Allocation: The Heart of Automation
AI-driven resource allocation in cloud systems is revolutionizing resource management by adapting to real-time computational needs. Predictive analytics forecast resource demand, while machine learning optimizes allocation for cost-efficiency. This dynamic approach moves beyond static models, enabling efficient resource scaling and ensuring high performance. These innovations contribute to building more sustainable, adaptive cloud infrastructures, enhancing both efficiency and cost-effectiveness.
Autonomous Systems: Minimizing Human Intervention
Intelligent cloud automation is advancing with autonomous systems that minimize human intervention. These systems use reinforcement learning, anomaly detection, and predictive analytics to manage cloud infrastructures. Anomaly detection identifies unusual behavior, allowing systems to resolve issues before they escalate. By analyzing historical data, AI predicts potential failures and takes corrective actions autonomously, enhancing uptime and reliability. This self-healing capability is a game-changer for businesses requiring high availability.
Predictive Analytics: The Key to Resource Optimization
Predictive analytics enhances cloud management by forecasting future resource needs based on past data and performance trends. AI-driven systems can make real-time decisions to adjust workloads and optimize resource allocation. This capability aids in cost management by preventing over-provisioning, ensuring efficient resource use. As cloud infrastructures grow more complex, the importance of predictive analytics will continue to rise, helping businesses stay ahead of performance and financial challenges.
The Future: AI’s Role in Shaping the Cloud Landscape
Looking ahead, the trajectory of cloud computing is clear: AI and ML are set to dominate. As these technologies continue to advance, cloud systems will become more autonomous, capable of learning from experience and optimizing themselves based on evolving demands. The combination of predictive analytics, intelligent resource allocation, and autonomous problem resolution will define the future of cloud computing.
The potential of these innovations is immense, promising a future where cloud environments can not only optimize their own performance but also anticipate and prevent disruptions before they occur. This new wave of intelligent automation is laying the foundation for self-sustaining cloud ecosystems that will redefine how organizations approach computational infrastructure.
In conclusion, the integration of AI and ML into cloud computing is not just a trend—it’s a revolutionary shift that is changing the very nature of how we manage and optimize cloud infrastructures. The innovations discussed in Venkatesh Kata‘s work illustrate a future where cloud environments are more intelligent, responsive, and efficient. As AI-driven systems continue to evolve, they will play an increasingly pivotal role in shaping the future of cloud computing, making it more autonomous and capable of self-optimization with minimal human intervention. As we move forward, organizations will need to embrace these advancements to stay competitive and fully realize the potential of intelligent cloud automation.
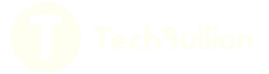