In the modern digital transformation era, automotive safety has undergone a paradigm shift with the rise of vision-based safety systems. Mayank Rai, an expert in automotive technology, presents a comprehensive statistical evaluation of these systems, revealing their effectiveness in crash prevention and safety enhancement. His research explores the intricate technological advancements that make vehicles smarter and safer.
From Passive to Proactive Safety Measures
Traditional vehicle safety features have primarily been reactive, mitigating crash consequences rather than preventing collisions. Vision-based systems, however, employ real-time image processing and artificial intelligence to detect hazards before they escalate. By integrating computer vision, these systems significantly enhance vehicle perception, enabling early intervention strategies such as automatic emergency braking and lane departure prevention.
Evolution of Vision-Based Systems in Vehicles
Vision-based automotive safety began in the 1990s with basic lane departure warnings, constrained by limited computing power. As deep learning and sensor technology advanced, these systems evolved significantly. By the 2010s, real-time object detection and comprehensive environmental awareness became standard, enhancing road safety. Modern vehicles now feature sophisticated vision-based solutions, integrating AI to detect pedestrians, monitor blind spots, and prevent collisions. Continuous improvements in machine learning, camera resolution, and sensor fusion have revolutionized vehicle safety, enabling autonomous decision-making. The evolution of vision-based systems has transformed driving, reducing accidents and paving the way for fully autonomous transportation.
The Role of Advanced Driver Assistance Systems (ADAS)
ADASs, incorporating vision-based safety systems, is now a critical component in modern vehicles. These systems include adaptive cruise control, pedestrian detection, and blind-spot monitoring. Research indicates that by 2026, over 70% of new vehicles will integrate vision-based ADAS, driven by regulatory policies, consumer demand, and technological feasibility.
Statistical Evidence of Safety Improvements
A robust analysis of crash data from sources like the National Highway Traffic Safety Administration (NHTSA) reveals that vision-based safety features significantly reduce crash frequency. Vehicles equipped with forward collision warning and automatic emergency braking have demonstrated a reduction in rear-end collisions by 38% to 53%. Similarly, lane departure warning systems contribute to a 21% reduction in single-vehicle crashes, increasing to 36% when combined with lane-keeping assistance.
Technological Innovations Driving Accuracy
Three major innovations drive the efficacy of vision-based systems:
- High-Resolution Cameras: Systems with cameras exceeding 2MP resolution improve detection accuracy, particularly in low-light conditions.
- Deep Learning Algorithms: Advanced AI models like convolutional neural networks (CNNs) enhance object recognition, ensuring rapid and precise detection.
- Sensor Fusion: Integrating radar and LiDAR with vision systems minimizes environmental limitations, improving reliability in adverse weather conditions.
Challenges in Real-World Applications
While vision-based safety systems hold great promise, they face notable challenges. Performance can drop by up to 34% in low-light conditions, while rain, snow, and fog can reduce effectiveness by 5% to 50%. False positives and negatives further impact reliability, raising safety concerns. Detecting small road users, such as motorcyclists and pedestrians, remains particularly difficult due to occlusions and varying visibility. These limitations highlight the need for continuous advancements in sensor fusion, AI-driven enhancements, and adaptive algorithms to improve real-world performance.
Regulatory and Industry Considerations
Global regulatory bodies are striving to standardize safety assessments for autonomous and advanced driver-assistance systems. The European Union now mandates automatic emergency braking in new vehicles, while U.S. regulations are gradually evolving. Industry experts advocate shifting from traditional pass/fail safety evaluations to performance-based assessments that better reflect real-world driving conditions. This transition aims to enhance vehicle safety by ensuring that autonomous systems are rigorously tested under diverse scenarios, improving reliability and overall road safety.
Future Developments in Automotive Vision Systems
Emerging technologies such as neuromorphic vision sensors, transformer-based AI models, and self-supervised learning are shaping the future of automotive safety. These innovations aim to enhance perception in complex environments, reduce system errors, and improve reaction times.
In conclusion, Mayank Rai‘s research underscores the transformative impact of vision-based safety systems on road safety. As these technologies continue to advance, their widespread adoption will contribute to reducing traffic fatalities and enhancing driver and pedestrian safety. The integration of AI, high-resolution imaging, and sensor fusion is paving the way for safer and more autonomous vehicles, making roads more secure for everyone.
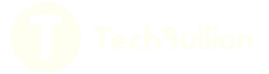