In a world increasingly reliant on real-time data and uninterrupted digital services, Prabhu Govindasamy Varadaraj presents a transformative vision for system observability. With expertise rooted in data-driven intelligence and automation, Varadaraj’s latest technical exposition brings to light the power of machine learning (ML) and artificial intelligence (AI) in revolutionizing digital system monitoring.
Smart Systems, Smarter Monitoring: Advancing Observability with Machine Learning
In a world increasingly reliant on real-time data and uninterrupted digital services, He presents a transformative vision for system observability. With deep expertise in data-driven intelligence and automation, Varadaraj’s latest technical exposition explores how machine learning (ML) and artificial intelligence (AI) are revolutionizing digital system monitoring.
From Monitoring to Understanding
Modern digital infrastructures have outgrown traditional, reactive monitoring systems. Legacy models, built on static thresholds and manual dashboards, struggle to cope with the vast streams of data generated by today’s applications. Recognizing this challenge, He highlights a paradigm shift—leveraging ML and AI to evolve observability platforms beyond simple monitoring toward deeper system understanding. This transformation enables proactive anomaly detection, intelligent alerting, and significantly faster issue resolution, moving from raw data collection to operational intelligence.
Decoding Observability: Metrics That Matter
At the core of observability lies a triad—metrics, traces, and logs. Together, they offer a comprehensive view of system health and performance. The article emphasizes key metrics such as system latency, throughput, error rates, resource utilization, and availability. By aggregating and analyzing these metrics through AI-powered platforms, organizations can shift from reactive responses to predictive insights.
An Architecture for Automation
The backbone of intelligent observability lies in its architecture. The article delves into how platforms like Splunk integrate machine learning into system monitoring. Splunk’s Machine Learning Toolkit (MLTK) enables predictive modeling and pattern recognition, while IT Service Intelligence (ITSI) facilitates service health visualization and correlation. These tools transform raw data into actionable insights, allowing enterprises to detect, predict, and mitigate operational risks in real time.
Engineering Intelligence: From Data to Decisions
Building an intelligent observability system requires more than just adopting tools—it demands a strategic approach. He also outlines a systematic framework that includes data collection, feature engineering, model training, and deployment. The process begins with structured data preprocessing—parsing timestamps, handling anomalies, and extracting features—before feeding it into ML algorithms capable of classification, regression, and time-series analysis. These models integrate into dashboards and alerting mechanisms, enabling teams to take swift, informed actions.
Performance in Practice
Organizations adopting ML-driven observability solutions report significant improvements in mean time to detection (MTTD) and mean time to resolution (MTTR). Proper configuration and infrastructure sizing are crucial to ensuring real-time data ingestion and analysis without performance degradation. The article presents evidence that AI-powered observability platforms efficiently handle large-scale data, optimizing resource allocation and minimizing downtime.
Challenges on the Path to Intelligence
Despite its advantages, intelligent observability is not without challenges. Security integration, hybrid environment monitoring, and log management remain common pain points. He emphasizes the need for a strategic deployment architecture supported by security information and event management (SIEM) solutions. Proactive planning, unified data pipelines, and automated incident response systems are essential for overcoming these complexities. Organizations must also address data sovereignty concerns and bridge skill gaps among DevOps teams to maximize the benefits of AI-driven monitoring.
The Road Ahead: Proactive, Predictive, Unified
The future of observability lies in enhanced automation, adoption of open standards like OpenTelemetry, and the convergence of security and performance monitoring. Emerging platforms are evolving toward a unified “single pane of glass” for metrics, logs, and traces—ushering in an era of holistic observability. Predictive analytics and automated remediation are no longer futuristic goals but are becoming fundamental expectations for enterprise monitoring systems. As digital infrastructures grow increasingly complex, AI-driven insights, real-time anomaly detection, and cross-platform correlation capabilities will define next-generation observability solutions.
In conclusion, Prabhu Govindasamy Varadaraj’s insights illustrate not just a technological shift but a strategic imperative for modern organizations. As digital infrastructures become more intricate, AI-powered observability systems will serve as the backbone of reliable and resilient operations. With intelligent monitoring becoming integral to business continuity, this innovation marks a significant step toward operational excellence in the digital age.
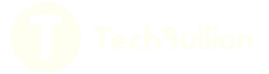