In the face of the ever-growing data explosion, organizations have struggled to keep up with traditional data analytics methods. Ramesh Mohana Murugan’s article offers a breakthrough solution: deterministic sampling. This innovative framework provides a scalable and systematic approach to analytics, allowing businesses to extract meaningful insights while minimizing computational inefficiencies.
A Paradigm Shift in Data Sampling
Data overload is a pressing issue in today’s digital economy. As the volume of global data expands exponentially, organizations find themselves battling against the growing cost of processing large datasets. The traditional methods of handling such massive amounts of data are increasingly becoming impractical, leading to slower performance and higher costs. Deterministic sampling provides a powerful solution by offering a way to efficiently handle big data without sacrificing accuracy..
The Mechanics Behind Deterministic Sampling
What sets deterministic sampling apart from traditional methods is its predictable, reproducible nature. Unlike probabilistic sampling, which relies on random selection, deterministic techniques apply consistent criteria for data selection, such as modulo-based filtering. This ensures that the same data subset is always selected under the same conditions, guaranteeing reliable and consistent results. This predictability is vital for organizations that need stable and comparable insights over time.
The Efficiency Boost: From Hours to Seconds
One of the most remarkable benefits of deterministic sampling is its ability to drastically reduce processing times. Take the case of Instagram, where deterministic sampling was employed to analyze user engagement patterns. By sampling just 0.1% of the data, Instagram was able to reduce processing times by 200 times, from several hours to just seconds, without sacrificing accuracy. This kind of efficiency is a game-changer for businesses struggling with long query times and high computational costs.
Maintaining Accuracy While Reducing Volume
One of the common challenges with data sampling is ensuring that the sample remains statistically representative of the whole dataset. Deterministic sampling addresses this concern by maintaining the essential properties of the full dataset. This method consistently delivers accurate insights, even with reduced data volumes.
For instance, in association rule mining, deterministic sampling preserves up to 92% of the frequent patterns in the original data, while analyzing just 15-20% of the records. This is a significant improvement over random sampling, which typically retains only 78-85% of these patterns. By carefully selecting data points, deterministic sampling ensures that businesses can still extract meaningful insights without the burden of processing vast amounts of data.
Tailoring Sampling for Specific Needs
Deterministic sampling is not a one-size-fits-all solution; it can be customized to fit specific data types and business needs. For example, stratified sampling is particularly useful for datasets that contain multiple subgroups, ensuring that each subgroup is represented proportionally. Cluster-based deterministic sampling is ideal for hierarchical or nested data, preserving relationships within groups.
Implementing Deterministic Sampling: Challenges and Considerations
While deterministic sampling offers substantial advantages, it is not without its challenges. The approach can struggle with highly skewed or irregular data distributions, and organizations must be mindful of ensuring that rare events or outliers are adequately represented. Additionally, as data characteristics evolve over time, organizations must adjust their sampling parameters to maintain accuracy.
Moreover, integrating deterministic sampling into existing systems can be complex. It requires careful planning, validation, and ongoing monitoring to ensure that the sampling process remains effective across all analytical platforms and use cases.
A New Era of Data Analytics
Deterministic sampling represents a significant leap forward in data analytics. It allows organizations to process vast amounts of data efficiently, preserving both accuracy and statistical integrity. By implementing this innovative framework, businesses can navigate the challenges posed by the data explosion, achieving faster insights, better decision-making, and improved operational efficiency.
In conclusion, as data continues to grow at an exponential rate, deterministic sampling stands as a cornerstone of scalable, efficient analytics. Organizations adopting this approach will find themselves better equipped to handle the complexities of modern data landscapes, transforming raw data into actionable insights that drive competitive advantage. Ramesh Mohana Murugan’s framework offers a promising path forward in the era of big data, helping organizations stay ahead in an increasingly data-driven world.
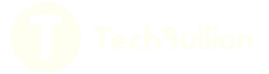