In the modern digital transformation era, Artificial Intelligence (AI) is reshaping agriculture, offering smarter, data-driven solutions to improve productivity, sustainability, and efficiency. Sandeep Reddy Pakeer, a researcher in AI-driven farming technologies, explores the profound impact of AI on agriculture, highlighting its applications in drone surveillance, soil analytics, and predictive modeling.
Revolutionizing Crop Monitoring with Aerial AI Systems
AI-powered drones are revolutionizing crop monitoring with advanced computer vision and multispectral imaging. These aerial systems capture high-resolution, real-time images to detect crop stress, diseases, and nutrient deficiencies with precision. Leveraging deep learning models, the drones analyze collected data, generating actionable insights for farmers. Real-time processing enables swift identification of potential issues, reducing reliance on manual scouting while improving efficiency. By enhancing precision agriculture, these drones optimize resource use, improve yields, and support sustainable farming practices. With their ability to cover vast fields quickly and accurately, AI-driven aerial monitoring is transforming modern agriculture, making farming smarter, more data-driven, and highly efficient.
Smart Soil Analytics with IoT Integration
AI-driven IoT networks are redefining soil monitoring by deploying smart sensors that measure moisture levels, pH balance, and nutrient content. By leveraging LoRaWAN technology, these sensors transmit data across vast agricultural landscapes with minimal power consumption. Advanced edge computing systems process this data locally, reducing the need for continuous cloud connectivity. This dynamic approach allows real-time adjustments in irrigation and fertilization, optimizing resource utilization and minimizing environmental impact.
AI-Powered Weather Forecasting for Risk Management
AI-driven weather prediction models are crucial for mitigating agricultural risks. Utilizing Long Short-Term Memory (LSTM) networks and gradient boosting algorithms, these systems analyze historical climate data to forecast droughts, frost, and storms. Bayesian neural networks quantify prediction uncertainty, allowing farmers to make informed decisions based on risk assessments. This proactive approach aids in implementing preventive measures, safeguarding crops against unpredictable climatic conditions.
Decision Support Systems for Precision Farming
AI-driven decision support systems revolutionize precision farming by integrating diverse data sources like satellite imagery and soil health analytics. These systems employ deep-learning models to optimize planting schedules, irrigation cycles, and fertilization strategies. Continuously refining predictions with real-time field data, AI enhances accuracy in agricultural decision-making. This technology maximizes crop yields while minimizing resource waste, improving efficiency and sustainability. By leveraging advanced analytics, farmers gain actionable insights, enabling smarter, data-driven farming practices that reduce costs and environmental impact while boosting productivity and resilience.
Sustainable Farming through AI-driven irrigation
Intelligent irrigation systems powered by AI optimize water distribution by analyzing soil moisture data, weather forecasts, and crop water requirements. These systems adjust watering schedules dynamically, preventing over-irrigation and conserving water. The integration of AI-based moisture prediction models ensures efficient water usage, reducing costs while promoting sustainable agricultural practices.
Optimizing Pest and Disease Control with AI
Machine learning models trained on vast datasets of plant disease images enable early detection of crop infections. AI-driven pest monitoring systems analyze insect behavior and environmental conditions to predict infestations before they occur. These predictive capabilities allow for targeted pesticide application, minimizing chemical use and reducing ecological impact.
Building an AI-Driven Agricultural Framework
A structured AI-driven architecture enhances farming efficiency through a multi-layered approach. The data collection layer gathers field insights from drones and IoT sensors, while edge computing units process real-time information. Cloud-based analytics refine predictive models, delivering actionable insights through user-friendly applications. By integrating AI with existing farm management systems, farmers can achieve seamless automation and data-driven decision-making.
The Road Ahead for AI in Agriculture
The continued evolution of AI in agriculture is paving the way for more adaptive and resilient farming techniques. With advancements in federated learning and distributed computing, AI systems will become more efficient and accessible to farms of all sizes. By addressing challenges related to data integration and model adaptation, AI-driven solutions will further enhance global food security and environmental sustainability.
In conclusion, Sandeep Reddy Pakeer’s research highlights the transformative impact of AI in agriculture, showcasing how innovative technologies are reshaping traditional farming practices. With the growing adoption of AI-driven solutions, the agricultural sector is poised to achieve unprecedented levels of efficiency, sustainability, and productivity in the coming years.
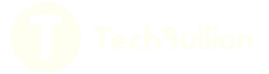