In today’s digital transformation era, scientific inquiry is increasingly guided by computational tools that uncover intricate biological mechanisms. Sarika Kondra, along with co-authors Feng Chen, Yixin Chen, Yuwu Chen, Caleb J. Collette, and Wu Xu, has contributed to a pioneering study that reimagines protein structure comparison through the TSR (Triangular Spatial Relationship)-based method. With a focus on geometric representation and functional motifs, their work sets a new benchmark for analyzing structural and functional relationships in proteins.
The TSR Method: Triangles as Structural Blueprints
At the heart of this research is the TSR (Triangular Spatial Relationship)-based method. Instead of viewing proteins as linear or folded sequences, this approach represents them as a collection of triangles formed by alpha carbon atoms. Each triangle is converted into an integer “key” that encodes geometric and biochemical information. This conversion allows for a novel and quantifiable way to compare protein structures, setting the stage for more nuanced structural classification and motif recognition.
Unpacking Structural Diversity Through Keys
The TSR methodology classifies protein structures using different types of keys: Common, common, Specific, specific, and uncommon. These keys act as digital fingerprints for protein substructures. By analyzing their distribution and frequency, the study reveals that while different protein families share large sets of structural components, they also possess uniquely distinguishing features. This hierarchical system enables a deeper understanding of how proteins evolve and diversify while maintaining core structural motifs.
Visualizing Protein Architecture in Two Dimensions
One of the standout innovations of the study is a new visualization technique that translates the TSR keys into pixel-based images. These 2D representations allow researchers to “see” the structural similarities and differences across protein families. The triplet combinations of amino acids are mapped onto a grid based on evolutionary similarity, and structural metrics like angle and distance are represented as pixel intensity. This method offers a unique visual insight into protein classification, potentially paving the way for advanced pattern recognition and image-based analysis in bioinformatics.
Clustering and Classification Enhanced by AI
To bolster the robustness of the TSR method, the research integrates machine learning—specifically, a 6-layer deep neural network—to classify proteins. Using the occurrence frequencies of keys as features, the model achieved classification accuracies as high as 95.77%. The authors also explored how sequence identity and sample size impact classification outcomes. Notably, structural similarity proved to be more reliable than sequence identity alone, reinforcing the value of 3D-based comparisons in functional prediction.
Size Filtering: A Novel Solution for Uneven Comparisons
The size filtering algorithm significantly improves protein structure comparisons by accounting for differences in protein length. It selectively excludes structural regions in larger proteins that fall outside the spatial scope of smaller ones. This normalization enhances the accuracy of similarity assessments, enabling more meaningful clustering. Consequently, proteins are grouped in a way that better reflects their actual biological functions, bridging structural variation and functional relevance with greater precision.
Motif Discovery in Ligand Binding Sites
A practical application of the TSR method is the identification of structure motifs in ligand binding sites. By isolating unique key patterns, the researchers successfully identified motifs specific to receptors such as androgen and progesterone receptors. These motifs were absent in unrelated protein families, highlighting the method’s sensitivity and specificity. This capability holds promise for drug discovery, where pinpointing exact binding pockets is crucial.
Exploring Amino Acid Preferences in Structural Cores
The study also uncovers interesting biochemical trends, such as the prevalence of leucine in common substructures across different protein families. This suggests a conserved role for certain amino acids in maintaining core protein stability, particularly in hydrophobic environments. Such findings provide further insight into protein folding and functional resilience.
In conclusion, Sarika Kondra’s TSR-based approach, developed in collaboration with her co-author, revolutionizes how protein structures are analyzed and interpreted. By integrating structural clustering, motif identification, advanced visualization techniques, and machine learning, this innovative platform offers a comprehensive framework for understanding the intricate architecture of proteins. The method not only deepens insights into protein function but also provides valuable tools for drug discovery, therapeutic innovation, and the advancement of precision medicine.
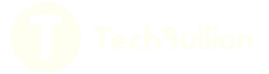