A new paradigm has emerged in the global automotive industry as it continues to find ways for achieving leaner, smarter, and more sustainable operations. Agentic AI is considered to be an excellent tool for driving autonomous optimization, real-time decision-making, and resilient supply chains. Recent work by data engineering and AI-enabled manufacturing expert Anil Lokesh Gadi demonstrates how the future of automotive production systems can be reshaped by Agentic AI.
Gadi has over a decade of cross-sectoral experience in cloud migration, advanced data analytics, and real-time processing architectures. His co-authored research paper titled “Emerging Technologies in Smart Computing, Sustainable Energy, and Next-Generation Mobility” discusses the importance of emerging intelligent systems in building self-adaptive and agile industrial processes. His work focuses particularly on Agentic AI, which may be defined as advanced AI systems capable of functioning as agents or autonomous decision makers. Gadi’s research highlights how these advanced systems are extremely relevant for the automotive sector struggling with growing demands for reduced emissions, rapid customization, and zero-defect manufacturing.
Agent-Based Intelligence in Automotive Manufacturing
According to Gadi, Agent-based intelligence has the potential to transform how modern automotive factories operate and evolve. All operational activities in traditional models of manufacturing are governed by centralized control systems. Though these systems are quite effective in stable conditions, they tend to struggle when there is a need to respond quickly to real-time disruptions such as unexpected surges in demand, machinery breakdowns, or real-time disruptions.
With the help of Agentic Ai, this centralized rigidity can be replaced by distributed intelligence across all system components including machine, sensor, workstation, and software module. This enables all components to operate as autonomous agents that can make independent decisions. It is also possible to empower these agents with the ability to communicate with other agents, assess operational parameters, observe their local environment, and execute decisions aligned with broader production goals.
Gadi informs that this autonomy is one of the most important enablers of adaptive and flexible manufacturing. For example, when a deviation in part alignment is detected by a robotic arm, instead of waiting for commands from a centralized system, it performs real-time recalibration and communicates the problem.
“Agentic AI creates a hive-like intelligence in the factory, where thousands of micro-decisions are made every minute without creating bottlenecks,” says Gadi. This distributed intelligence framework not only reduces latency in decision-making but also builds resilience, allowing the system to continue functioning even when certain components fail or encounter anomalies.
Agentic AI also fosters modularity and scalability by decentralizing control. Without any extensive reconfiguration, new processes and machines can be added to the production line. New agents added to the system start contributing autonomously after learning to operate independently within the existing environment. With this approach, the automotive industry can transition towards a high-variety, low-volume, and hyper-customized production.
Data Engineering and AI-Powered Factories
The entire concept of Agentic AI revolves around data. All the actions, reasoning, and perceptions of AI agents are fueled by data. It is important, however, to remember that even the most advanced AI algorithms falter without a strong foundation in data engineering.
Gadi has actively participated in developing large-scale, cloud-native data pipelines that allow continuous ingestion, enrichment, distribution, and cleansing of manufacturing data. These pipelines integrate data from many different sources such as quality inspection tools, robotic sensors, environmental monitors, supply chain platforms, and ERP systems. As a result, a unique data fabric is created, supporting AI-powered decision-making throughout the factory.
He emphasizes that handling latency-sensitive tasks require real-time data processing at the edge. On the other hand, long-term data such as supplier performance metrics and historical defect patterns is aggregated in the cloud for visualization, model training, and predictive analytics. To ensure scalability and reliability, he has incorporated schema evolution, data versioning, and streaming analytics frameworks utilizing tools such as Spark, Apache Kafka, and cloud-native data lakes.
Sustainability Goals
Agentic AI not only plays a vital role in driving efficiency, but also supports sustainability and environmental stewardship. Intelligent energy management is one of its most impactful contributions. AI agents can be used for monitoring energy consumptions continuously at the line, machine, and facility levels. Based on grid pricing, production schedules, and real-time demand, they independently make decisions to shift non-critical operations to off-peak hours, power down idle equipment, and redistribute loads to minimize peak energy use. This can help reduce electricity cost, carbon emissions, and energy wastage.
Agentic AI has an ever bigger role in facilities powered by renewable energy. It allows for smart integration with battery storage systems, solar panels, and transactive energy markets. In his research paper, Gadi mentions that privacy-preserving blockchain protocols can ensure transparency and security of transactions in distributed energy networks.
Final Thoughts
The automotive industry is under tremendous pressure to deliver cleaner, smarter, and faster vehicles. In this scenario, integration of Agentic AI is certainly a very promising way forward. With efforts from distinguished technology leaders such as Anil Lokesh Gadi, this vision can become a reality in the near future.
“Agentic AI brings us closer to a future where manufacturing systems are not just automated-but intelligent, resilient, and adaptive,” Gadi concludes.
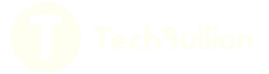