Neurodegenerative diseases such as Alzheimer’s, Parkinson’s, and Huntington’s have become increasingly prevalent with the aging of the global population. Naturally, this has posed serious challenges for healthcare systems across the globe. In addition to deteriorating the quality of life of millions of individuals, these chronic, progressive disorders also impose substantial social and economic burdens.
These conditions must be detected early and managed effectively to improve patient outcome, slow down disease progression, and alleviate healthcare burdens. A research conducted by Venkata Krishna Azith Teja Ganti provides valuable insights on improving understanding, diagnosis, and treatment of neurodegenerative diseases harnessing neural network models. His work makes a sincere effort to bridge the gap between clinical applications and emerging computational technologies.
Understanding the Challenge
Neurodegenerative diseases involve gradual degeneration of neurons, which results in progressive cognitive and motor dysfunction. Despite decades of research, understanding of the mechanisms underlying these conditions has remained incomplete. As a result, the process for developing effective therapies is quite complicated. Relying heavily on clinical assessments, imaging techniques, and post-mortem analysis, traditional diagnostic methods often fail to diagnose the condition until the occurrence of significant and irreversible brain damage.
In addition to limiting therapeutic options, this delay also reduces the potential effectiveness of interventions. Through his work, Ganti emphasizes that this critical gap can be addressed by leveraging advanced computational models that can identify subtle, early-stage biomarkers and forecast disease progression with high precision. Interventions become more effective when diagnosis is moved to much earlier stages. This also ensures that the dynamic processes of disease evolution can be targeted better by clinical research.
Neural Networks in Healthcare
Inspired by the structure of the human brain, Artificial Neural Networks (ANNs) are remarkably effective in pattern recognition and predictive modeling. In his study, Ganti has explained the potential of these models to revolutionize neurodegenerative disease management. He claims that neural networks can identify early indicators of disease by analyzing complex datasets encompassing genomic profiles, medical imaging, cognitive assessments, and electrophysiological recordings.
Ganti has explored how the application of ANNs can benefit various stages of neurodegenerative disease research, from prediction of risk to disease progression modeling. According to him, though there are many predictive models that can be used for conditions like Alzheimer’s and Parkinson’s, there is still an urgent need for models capable of accurately mapping disease stages and offering dynamic and personalized insights into patient trajectories.
Early Detection with Neural Networks
In the framework proposed by Ganti, one of the critical applications of neural networks is the early detection of diseases through multimodal data integration. By combining electrophysiological signals, cerebrospinal fluid biomarkers, and neuroimaging data, neural networks can detect patterns associated with the onset of Parkinson’s or Alzheimer’s even before the emergence of symptoms.
As an example, models trained on MRI scans of the temporal lobe and cerebrospinal fluid biomarker profiles have delivered promising results in predicting Alzheimer’s disease development. Similarly, early identification of schizophrenia and other cognitive disorders can be assisted significantly by deep learning models capable of analyzing gray and white matter distributions. Ganti’s research underscores that the predictive accuracy and generalizability of neural network models can be enhanced by integrating such diverse data types.
Disease Progression Modeling
Ganti’s research also discusses how the progression of neurodegenerative diseases can be modeled using neural networks. Traditionally, limited longitudinal data and heterogeneous patient presentations have hampered the understanding of disease progression. Neural networks can solve this problem by detecting dynamic changes in brain structure and function over time through analysis of longitudinal datasets.
Cortical surface changes have been modeled using advanced techniques such as spatiotemporal graph convolutional neural networks. This allows researchers to map how neurodegeneration spreads across different brain regions. By providing a “propagation score,” these models reveal how disease-related changes move through the brain’s networks over time. These insights can be extremely valuable for predicting clinical milestones, staging diseases, and tailoring interventions accordingly.
Challenges and Ethical Considerations
There is no denying the fact that the potential of neural networks is immense. However, Ganti also cautioned against inherent challenges such as noisy measurements, data scarcity, and the interpretability of neural network outputs. Widespread clinical adoption demands that the models are transparent and their predictions are clearly explained to patients and clinicians.
Ganti also emphasizes that ethical considerations are paramount. It is important to carefully manage issues such as data privacy, fairness, and potential biases in training data. He also mentions that there is an urgent need for robust governance frameworks to guide the responsible development and deployment of AI models in healthcare.
Final Thoughts
Venkata Krishna Azith Teja Ganti’s research provides a promising roadmap for the early detection and management of neurodegenerative diseases harnessing the power of neural networks. With deep understanding of big data, advanced machine learning techniques, and clinical challenges, his research paves the way for more proactive, personalized, and effective healthcare solutions.
“Advances in neural network modeling provide us with unprecedented tools to understand, detect, and manage neurodegenerative diseases. By integrating diverse data sources and focusing on interpretability, we can shift from reactive treatment to proactive intervention, ultimately improving patient lives and transforming healthcare delivery,” he concluded.
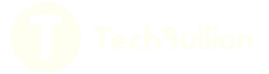