Digital finance is quickly emerging now as the face of global economies by enhancing the speed, cost-effectiveness, and reach of financial services. While its benefits are undeniable, digital finance has also made it extremely important to predict, detect, and manage risks. Heavily dependent on periodic assessments and historical data analysis, traditional risk management models are often ineffective against the rising sophistication of financial fraud, complex regulatory environments, and evolving cyber threats. With the globalization of financial services and ever-increasing volume of digital transactions, financial institutions must improve their risk detection frameworks and find solutions that are more dynamic and real-time.
A noted expert in predictive analytics, AI-driven automation, and enterprise integration, Avinash Pamisetty has outlined a pragmatic roadmap that enhances proactive risk detection and compliance strategies in the digital financial ecosystem by leveraging artificial intelligence (AI). His research titled “AI Powered Predictive Analytics in Digital Banking and Finance: A Deep Dive into Risk Detection, Fraud Prevention, and Customer Experience Management” paves the way for a more secure and future-ready financial sector
Risk Management in the Era of Digital Finance
Addressing the complexities of today’s digital finance landscape is beyond the capacity of traditional risk management models because of their dependence on static assessments and historical data. With the advent of open banking, fintech, and globalized transaction networks, new vectors have emerged for cybersecurity, credit, and operational risks. Unfortunately, static models are not efficient enough to adapt to the dynamic nature of digital transactions, which can cause delayed responses and heightened vulnerability.
Pamisetty informs that integration of AI-powered predictive analytics can bridge this gap. Unlike traditional models, systems powered by AI can continuously learn from real-time data, adapt to emerging threats, and identify hidden patterns. As a result of this dynamic capability, financial institutions can not only detect risks in advance, but also anticipate and mitigate problems before they escalate.
AI-Powered Predictive Analytics
Strategic deployment of AI-powered predictive analytics tools is the core concept of Avinash Pamisetty’s roadmap. By analyzing vast volumes of structured and unstructured financial data, these technologies can forecast risks across multiple domains, from operational glitches and credit defaults to cybersecurity breaches.
Three critical pillars of AI-driven risk detection, as mentioned in his research are
- Behavioral Pattern Recognition: AI systems can flag anomalies indicative of compliance breaches, account hijacking, or fraud by understanding customer behavior.
- Continuous Data Monitoring and Learning: AI models enable continuous updates to risk profiles by ingesting and analyzing transactional data in real-time.
- Contextual and Predictive Risk Scoring: Based on evolving contextual factors, AI tools can generate predictive risk scores, which enable proactive interventions.
Enhancing Fraud Detection and Prevention
In his research, Pamisetty has underscored the critical role of AI in strengthening fraud detection mechanisms. The introduction of digital banking has brought up a wide array of sophisticated fraud techniques such as universal transaction fraud, rogue applications, identity theft, and more. Traditional rule-based detection systems often lag behind the rapidly evolving tactics of cybercriminals.
Pamisetty recommends AI-driven systems combining supervised and unsupervised machine learning models that are capable of detecting known fraud patterns as well as previously unseen anomalies. With techniques such as deep neural networks and adversarial machine learning, systems can dynamically adjust to new fraud tactics without requiring extensive manual recalibration.
Predictive analytics can also reduce response times significantly and minimize financial and reputational damages by enabling real-time fraud detection at the transactional level.
Operational Risk Management
Operational risks such as cybersecurity breaches and system outages are significant threats to financial institutions. Pamisetty argues that prediction and mitigation of these risks requires AI models with natural language processing (NLP) and advanced data mining capabilities.
AI systems can identify early indicators of operational disruptions by analyzing data from diverse sources including regulatory updates, social media, internal systems, and news feeds. Pamisetty also recommends banks to integrate AI into their incident response systems so that alerts are contextualized, prioritized, and actioned swiftly.
Compliance and Regulatory Intelligence
With regulations continuously evolving across jurisdictions, compliance is one of the most complex challenges for financial institutions. Pamisetty envisions AI-powered regulatory intelligence platforms that automatically track, interpret, and operationalize compliance mandates. By embedding AI into compliance workflows, organizations can move towards a state of continuous compliance, which can enhance regulatory relationships and reduce legal risks.
Final Thoughts
Avinash Pamisetty’s research highlights how financial institutions can move beyond reactive risk management and embrace transparent, predictive, and adaptive models to navigate an increasingly complex landscape. Financial institutions can achieve a new era of security, efficiency, and sustainable growth with strategic integration of AI.
Summarizing his research, Pamisetty said, “AI-powered predictive analytics empowers institutions to foresee threats, reinforce compliance, and build resilient customer relationships. As we stand at the crossroads of innovation and trust, financial leaders must harness AI not merely as a tool but as a transformative ally in securing the future of digital finance.
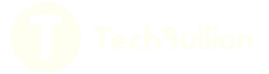