Payment systems are integral components of today’s increasingly digitized economy. Digital banking innovations, mobile payments, and online financial transactions have created unprecedented convenience for all. This transformation, however, has also resulted in the emergence of sophisticated fraud tactics capable of exploiting vulnerabilities in customer behavior, merchant systems, and payment networks. Unfortunately, as these techniques have evolved, traditional fraud detection methods have struggled to handle these threats effectively.
AI, machine learning, and data engineering expert Hara Krishna Reddy Koppolu has proposed a roadmap to address these challenges. By merging the capabilities of deep learning with the adaptability of agentic AI, his approach provides a roadmap to help merchant services achieve intelligent, autonomous, and resilient fraud detection systems. In addition to addressing the pressing needs of today’s digital economy, his research paper titled “Deep Learning and Agentic AI for Automated Payment Fraud Detection: Enhancing Merchant Services through Predictive Intelligence” also anticipates the future landscape of payment security.
The Evolving Payment Fraud Landscape
Payment fraud is a dynamic threat that exploits vulnerabilities in consumer interactions, merchant systems, and payment platforms. As a result of these fraudulent activities, merchants bear the brunt of operational burdens, financial losses, and reputational risks. Though there has been significant advances in recent years, existing fraud detection models often suffer from delayed responses, high false-positive rates, and limited adaptability to new fraud patterns.
According to Koppolu, most current models don’t have the real-time, autonomous learning capabilities required for outpacing modern fraudsters because they are either rules-based or reliant on traditional machine learning. He strongly believes that it is no longer sufficient for fraud detection systems to be predictive. The present landscape requires systems that are also explainable, adaptive, and capable of operating autonomously at scale.
Deep Learning and Agentic AI
The integration of deep learning and agentic AI is at the core of Koppolu’s research. Deep learning offers unparalleled fraud detection capabilities because of its ability to analyze vast datasets and detect complex patterns. On the other hand, agentic AI systems bring self-learning capabilities, autonomy, and decision-making authority. When these two are combined, a predictive and dynamically responsive framework is created.
By recognizing hidden patterns in transaction data, deep learning models can enable early detection of anomalies that may indicate fraud. However, for adapting to evolving fraud tactics, it depends heavily on retraining and human supervision. To bridge this gap and turn deep learning models into proactive fraud-fighting agents, agentic AI enables AI systems to learn in real-time from their environments, make independent decisions, and explain their actions.
Leveraging reinforcement learning techniques, multi-layered neural networks, and explainable AI mechanisms, Koppolu looks to build systems that continuously refine their detection models with each new interaction.
Smarter and Real-Time Fraud Detection Systems
Traditional fraud detection systems detect fraudulent activities after their occurrence because they often operate on delayed feedback loops. The integrated framework proposed by Koppolu aims to shift fraud detection into a predictive and real-time paradigm. Agentic deep learning systems ingest transaction data, monitor patterns, and make autonomous decisions to prevent fraudulent transactions before there is any impact on customers or merchants.
These systems prioritize
- Learning and evolving with new fraud strategies.
- Reducing dependence on human intervention.
- Operating across millions of transactions seamlessly without performance bottlenecks.
- Providing transparent decision-making processes to meet ethical and regulatory requirements.
Koppolu emphasizes that these goals can only be achieved by designing architectures capable of facilitating rapid ingestion of data, spontaneous model adjustments, and minimal latency between detection and decision-making.
Enhancing Merchant Services
By adopting deep learning and agentic AI based fraud detection systems, merchants can enjoy several tangible benefits.
- Minimizing chargebacks and fraudulent refunds by early detection and prevention.
- Autonomous decision-making reduces the need for large fraud investigation teams.
- Enhanced customer confidence through faster and more accurate fraud prevention.
- Explainable AI ensures transparency and accountability in fraud detection practices.
Bias, False Positives and System Fragility
Koppolu has also addressed the potential challenges while merging deep learning with agentic AI. The risk of bias in fraud detection models can unfairly target certain demographics or behaviors. To mitigate this problem, he recommends developing ethical agentic AI systems that prioritize fairness, transparency, and inclusivity.
False positives are another major concern that can flag legitimate transactions as fraudulent ones. Too many false positives can harm customer relationships and lead to loss of trust in the fraud detection system. Agentic AI systems can refine their predictive capabilities and strike a better balance between sensitivity and specificity by enabling continuous learning and feedback loops.
To address the problem of system fragility, Koppolu recommends that systems should be robust enough to withstand adversarial attacks and evolving fraud strategies. His framework proposes to fortify fraud detection mechanisms against sophisticated threats by incorporating adversarial training, ensemble modeling, and continuous system audits.
Shaping the Future of Digital Commerce
Adoption of agentic deep learning frameworks for fraud detection can play a significant role in strengthening trust in digital payment systems. This is critical to fueling the growth of e-commerce, fintech, and digital banking ecosystems.
“The future of fraud detection lies not just in identifying threats, but in anticipating them. By merging deep learning with agentic AI, we can create systems that think, learn, and adapt faster than fraudsters. It is about moving from reactive defense to proactive resilience, empowering merchants to deliver secure, seamless payment experiences. Ethical, explainable, and autonomous AI will be the cornerstone of tomorrow’s digital commerce landscape,” Koppolu concluded.
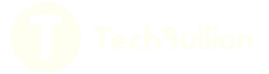