Introduction
The current era of artificial intelligence and big data development cause algorithm to dominate various fields including financial organizations along with recruitment agency and police force and academic institutions. The efficient operations of algorithms face critical challenge because they operate without clear method and continue to reproduce existing social biases. The modern legal system need updates to establish innovative capability alongside protection for discriminated population groups. This complete research examine bias management regulations monitoring transparency feature and fairness requirements and evaluates GDPR and CCPA’s ability to deliver proper protection.
Understanding Algorithmic Bias and Its Impact
AI-control system discriminate certain people or groups by producing negative outcome based on demographic detail such as race, gender, age and social position repeatedly. Several origins causes this bias effect including the training data quality along with algorithm design structure and system deployment environment. An algorithm trained with discriminatory historical data tensor its patterns into recommendations thus worsening established gender and ethnic limitations. Buolamwini and Gebru (2018) performed research on commercial facial recognition systems thus proving their systems failed to recognize black women properly yet accurately recognized male faces with white skin. The maintenance of existing inequalities alongside diminished public confidence in automated life-deciding systems exists as major drawbacks of biases in automated systems.
Current automated systems and their biased algorithms create detrimental effects in essential areas such as hiring and law enforcement and medical treatment and academic programs and these effects primarily target vulnerable ethnic groups. The implementation of automated decision-making systems at work through resume screening and performance evaluation tasks ends up actively promoting discrimination (Todolí-Signes, 2019). Studies reveal that COMPAS among other risk assessment instruments in criminal justice unfairly classify Black defendants as high-risk offenses that impact judicial proceedings (Angwin et al., 2022). The examples show algorithms do not remain neutral because they bring to light and deepen the encoded prejudices and values and priorities from their creators. The complex nature of these systems (Zarsky, 2016) produces detection challenges which demands a framework that secures legal and ethical standards for algorithmic governance.
Algorithmic Transparency and the Right to Explanation
Users along with affected individuals need full visibility into algorithm operations to obtain algorithmic transparency. The right to ensure fair decisions as well as accountability rests on solving this critical matter for AI systems to operate without biases. The availability of transparency makes it possible for people to see how decisions form and which data gets used and how discrimination plays out. Hiring system along with criminal justice operation and lending services requires prompt transparency solution because algorithmic decision determine what outcomes people receive in these area. Machine learning model need complete transparency although achieving this level become challenging for deep learning network since their internal process remain impossible to understand. People need way to challenge algorithmic decisions affecting them and transparency in process explanation ensure this possibility to maintain faith in automation thus people avoid discarding trust in algorithm (Doshi-Velez et al., 2017). Transparency maintain its ethical position beyond technological requirements by facilitating assurance that automation maintain social principles and safeguards fundamental human right.
The right to explanation function as top legal tool to handle algorithmic opacity because current privacy protection law such as the GDPR in the EU enforce it. People have the right under Article 22 of GDPR to get information about automated choice that lead to significant outcome such as profiling or automated credit scoring. Organization must enhance their accountability through explainable reason provision when making automated judgments under GDPR requirement. Complex algorithm limit effectiveness of GDPR transparency standard (Todolí-Signes, 2019). The combination of legal transparency tools through explanation capabilities leads to more effective algorithmic bias defense within society. The legal-technological relationship governing algorithmic accountability needs flexible adjustment to defend individual rights while allowing AI system advancement in (Prinsloo et al., 2023).
Fairness and Accountability in Algorithmic Systems
Systemic programs with implications for human life functions including hiring practices and loan decisions and law enforcement applications. When algorithms operate without proper management they increase existing biases along with producing discriminatory decisions. An algorithm trained on discriminatory data tends to duplicate the contained prejudices which lead to unacceptable mistreatment targeting racial groups and women. The accuracy problem in facial recognition systems and predictive policing algorithms becomes especially problematic because studies demonstrate that different social groups exhibit varying levels of performance (Buolamwini & Gebru, 2018). For fair algorithmic decision making it is essential to study data as well as algorithms to verify that no specific group faces unintentional disadvantages. Zarsky (2016) explains fairness needs to comprise a set of multiple fairness dimensions that include diverse forms of equity. Achieving fairness involves actively working to minimize biases, test systems for adverse impacts, and involve affected groups in the development and evaluation of algorithms.
Algorithmic system accountability links directly to fairness through responsibilities because it necessitates accountable parties to bear liability for their decision outcomes. The principle plays an important role in solving doubts regarding the unclear patterns and unpredictable nature of algorithmic decision policies. Accountability mechanisms provide the means to make both individuals and organizations accountable whenever they create discriminatory results or violate rights. The GDPR along with the CCPA gives individuals affected by algorithms protection because it requires transparency from algorithms and permits people to contest automated decisions (Todolí-Signes, 2019). Complex algorithms and proprietary nature of systems present the main hurdle in enforcing accountability because it becomes hard to determine responsibility. When harmful outcomes occur in particular situations the assignment of blame becomes impossible because decision-making algorithms lack clarity and following their trajectory is excessively complicated (Doshi-Velez et al., 2017).
Legal Instruments: GDPR and CCPA
The General Data Protection Regulation serves as the largest legal framework protecting privacy and data operations and regulating the use of algorithmic systems in all EU member states. Under GDPR officials have established specific procedures to decrease risks of algorithmic bias and establish better communication between systems and end users. Under GDPR every European citizen maintains the right to seek details about significant automated system effects (Todolí-Signes, 2019). This provision ensures effective control over complicated algorithm-based decisions through the ability of individuals to protest but also boosts organizational transparency together with accountability measures. Under the GDPR personal data processed by algorithmic systems must be handled fairly as well as transparently while having specific use purposes defined. The GDPR regulates personal data processing through obligation addressing discriminatory outcomes related to biased data and algorithms which forces data controllers to develop suitable measures for preventing unjust decisions (Zarsky, 2016).
California Consumer Privacy Act protects California residents by providing privacy rights equivalent to several GDPR principles without being applicable outside the region. Under CCPA residents obtain three main rights which include the ability to review their business-collected data while receiving the option to have their data removed and the choice to stop personal data sales. The CCPA establishes foundations for comprehensive data protection measures even though such provisions do not concern algorithmic transparency directly but they could affect automated systems indirectly. Through its data protection system the CCPA gives users control over their information which creates a framework for showing responsibility in algorithmic modeling procedures. The regulations in the act about “consumer rights” support an emerging global trend to equip people with means against algorithmic discrimination (Angwin et al., 2022).
Toward Proactive Governance
The implementation of proactive governance measures for algorithmic decision-making involves predicting and reducing potential damages including algorithmic bias before they appear as operational issues. The design implementation of algorithms should initially address fairness and transparency alongside accountability instead of reactive post-event reaction (Prinsloo et al., 2023). A vital proactive governance approach includes the creation of monitoring tools known as algorithmic auditing frameworks which enable permanent oversight of decision-making fairness and precision. The goal of these audits is to detect biases during development so algorithms become equitable by design and developers and organizations maintain their responsibility to handle discriminatory results. The proposed EU AI Act demonstrates the current focus on proactive governance by setting specific rules for artificial intelligence technological development programs to combat discrimination elements and system hidings (Todolí-Signes, 2019).
Conclusion
The regulation of algorithmic bias require combining transparency with fairness with legal accountability under proactive governance. Society demand urgent intervention to stop newly emerging social inequality problem due to continuous algorithmic decision-making power across employment and education and healthcare and criminal justice sector. The GDPR and CCPA establishes fundamental legal requirements yet require security technical solution as well as consistent ethical tracking plus broad community participation to govern their implementation.
For successful development of AI system there needs to be systematic process because trustworthy public-serving technology require ongoing examination together with responsible management practices. The construct of ethical principle must be incorporated into algorithm development phases and updated regulation serve as principal mechanism to achieve this integration. A combination of well-structured relationship between technological expertise and both legislative professional and ordinary citizen is essential for successful human rights integration and technological advancement. Algorithm can only produce important contribution to fair choice decisions when following complete human-centered framework.
References
Angwin, J., Larson, J., Mattu, S., & Kirchner, L. (2022). Machine bias. In K. Martin (Ed.), Ethics of data and analytics (pp. 254–264). Boca Raton: Auerbach Publications.
Buolamwini, J., & Gebru, T. (2018). Gender shades: Intersectional accuracy disparities in commercial gender classification. In Conference on Fairness, Accountability and Transparency (pp. 77–91). PMLR.
Doshi-Velez, F., Kortz, M., Budish, R., Bavitz, C., Gershman, S., O’Brien, D., et al. (2017). Accountability of AI under the law: The role of explanation. arXiv. https://doi.org/10.48550/arXiv.1711.01134
Joamets, K. (2022). Plagiarism as a legal phenomenon and algorithm-based decision making. TalTech Journal of European Studies, 12(1), 146–164. https://doi.org/10.2478/bjes-2022-0015
Liu, Y., Liu, J., & Tan, S. (2023). Decision space partition based surrogate-assisted evolutionary algorithm for expensive optimization. Expert Systems with Applications, 214, 119075. https://doi.org/10.1016/j.eswa.2022.119075
Prinsloo, P., Slade, S., & Khalil, M. (2023). At the intersection of human and algorithmic decision-making in distributed learning. Journal of Research on Technology in Education, 55(1), 34–47. https://doi.org/10.1080/15391523.2022.2121343
Todolí-Signes, A. (2019). Algorithms, artificial intelligence and automated decisions concerning workers and the risks of discrimination: The necessary collective governance of data protection. Transfer: European Review of Labour and Research, 25(4), 465–481. https://doi.org/10.1177/1024258919876416
Zarsky, T. (2016). The trouble with algorithmic decisions: An analytic road map to examine efficiency and fairness in automated and opaque decision making. Science, Technology, & Human Values, 41(1), 118–132. https://doi.org/10.1177/0162243915605575
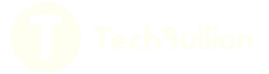