As digital systems grow more complex, the tools for monitoring and managing them are evolving rapidly. Traditional infrastructure monitoring services, which once relied heavily on manual oversight and static thresholds, are increasingly incorporating Artificial Intelligence (AI) to meet modern demands.
Among companies exploring this shift, firms like Sentineli.com are using AI to rethink how infrastructure and Kubernetes environments are monitored, aiming to address challenges such as alert fatigue, response delays, and operational inefficiencies.
The Growing Pressure on Monitoring Systems
With cloud-native architectures, microservices, and Kubernetes clusters becoming standard, IT environments have become highly dynamic. The sheer volume of alerts, notifications, and telemetry data often overwhelms traditional monitoring setups. Human operators, no matter how skilled, are increasingly challenged to triage and respond to issues in real time.
In response, many service providers are beginning to augment their monitoring platforms with AI-driven capabilities, aiming to automate repetitive tasks, filter noise from critical alerts, and improve response times.
Practical Applications of AI in Monitoring
One area where AI is making an impact is incident communication. Instead of relying solely on people to handle telephonic incident calls, AI systems can now automatically initiate, escalate, and document these communications. This reduces the delay between incident detection and acknowledgment, ensuring that on-call teams are informed faster and more consistently.
Another key application lies in notification management. Traditional monitoring tools often bombard teams with hundreds of alerts, many of which are redundant or low priority. AI-powered filtering systems analyze the context, correlate related events, and prioritize notifications, helping teams focus on the most urgent issues while reducing alert fatigue.
Companies like Sentineli.com are incorporating these approaches into their offerings, providing managed monitoring services that attempt to reduce manual overhead and improve operational resilience.
Addressing Kubernetes Observability Challenges
Kubernetes monitoring and observability present unique challenges due to the ephemeral nature of containers and the dynamic scaling of workloads. AI technologies can enhance Kubernetes observability by detecting patterns across clusters, forecasting resource bottlenecks, and identifying anomalies that might be missed through static monitoring rules.
Solutions that combine AI with Kubernetes monitoring provide DevOps teams with actionable insights, enabling faster root cause analysis and proactive infrastructure optimization.
As organizations scale their cloud-native deployments, the ability to maintain real-time visibility without being overwhelmed by data noise becomes increasingly critical.
What the Future Looks Like
The use of AI in monitoring is still at an early stage but is gaining momentum. Future developments are expected to include predictive analytics that detect incidents before they impact users, automated remediation workflows, and adaptive systems that can self-correct without human intervention.
While no single solution can eliminate the complexity of modern IT operations, the integration of AI into infrastructure and Kubernetes monitoring is a promising direction. Managed service providers experimenting with these technologies today are likely to set the standards for the next generation of observability platforms.
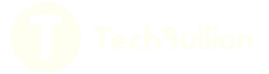