In today’s digital transformation era, artificial intelligence (AI) is revolutionizing healthcare operations, streamlining administrative processes, and enhancing patient care. Manuel Joy, one of the pioneers in this domain, presents compelling insights into agentic workflows AI-driven automation frameworks designed to tackle healthcare challenges. His research explores how these intelligent systems transform clinical efficiency, reduce administrative burdens, and improve decision-making accuracy.
Redefining Healthcare Automation with Agentic Workflows
Agentic workflows mark a significant advancement in healthcare automation, moving beyond traditional rule-based systems to dynamic, AI-driven processes. These intelligent systems utilize machine learning to interpret context, make adaptive decisions, and efficiently manage unstructured data and complex clinical pathways. By minimizing manual intervention, they accelerate administrative and clinical workflows, thereby reducing clinician burnout and improving diagnostic accuracy. Leveraging domain-specific language models and Retrieval-Augmented Generation (RAG), agentic AI streamlines documentation and patient management. This seamless integration not only enhances operational efficiency and decision-making but also transforms healthcare delivery into a more responsive, accurate, and patient-centered system.
AI in Clinical Documentation: Lightening the Workload
Clinician burnout, worsened by documentation overload, is a major healthcare issue. AI-driven workflows, leveraging NLP and agentic systems, automate up to 80% of routine tasks, streamlining patient encounters and enhancing care. Machine learning boosts diagnostic support, predicts health trends, and enables real-time patient monitoring. Domain-specific LLMs and RAG technologies reduce cognitive load, improve accuracy, and ensure evidence-based clinical decisions, significantly advancing healthcare delivery efficiency and outcomes
Enhancing Administrative Efficiency Through Automation
Agentic AI automates scheduling, coding, and insurance tasks, reducing errors and admin load. Real-time updates and approvals boost efficiency. Machine learning predicts complications and supports diagnostics, while domain-specific LLMs and RAG systems enhance documentation and enable accurate, context-aware clinical decisions.
AI-Powered Decision Support: Improving Diagnostic Accuracy
AI-driven agentic workflows enhance clinical decision-making by integrating domain-specific LLMs and RAG technology. These systems retrieve medical knowledge and analyze patient data in real-time, enabling faster, more accurate diagnoses. Physicians benefit from AI support, which improves treatment precision and reduces diagnostic errors.
Retrieval-Augmented Generation: Ensuring Evidence-Based Healthcare
RAG technology revolutionizes AI in healthcare by retrieving and synthesizing evidence-based insights, ensuring accurate, context-aware content that supports clinicians with trustworthy, misinformation-free decision-making from vast medical literature.
Optimizing Patient Management Through AI Integration
AI-driven workflows are transforming patient management by enabling intelligent monitoring that predicts complications and issues early warnings. These systems are especially valuable in chronic disease care, reducing hospital readmissions through timely interventions and enhancing long-term outcomes with continuous condition assessment.
Evaluating AI Performance in Healthcare
The effectiveness of agentic workflows in healthcare is measured through technical and clinical performance indicators. Key metrics include documentation accuracy, system reliability, and processing speed. AI achieving over 90% accuracy and near-perfect uptime proves viability. Clinically, these systems enhance provider satisfaction, improve patient care quality, and optimize resource utilization, demonstrating significant real-world impact.
The Future of AI in Healthcare: Emerging Innovations
AI in healthcare is rapidly evolving, with specialized models enhancing diagnostics and treatment in medical subspecialties. Advances in NLP and ambient clinical intelligence will streamline documentation by autonomously capturing patient-clinician interactions, reducing administrative tasks and improving efficiency, ultimately allowing healthcare professionals to focus more on patient care.
Predictive Analytics: A New Era of Population Health Management
Predictive analytics will transform healthcare by enabling AI models to assess risks, analyze social factors, and deliver insights. This proactive approach fosters early intervention, reduces hospitalizations, and enhances population health through a focus on preventive care and timely management.
Interoperability: Creating a Seamless Healthcare Ecosystem
A major hurdle in healthcare AI adoption is achieving interoperability. Future AI solutions will focus on seamless integration with existing IT systems, enabling efficient data exchange, reducing redundant tests, enhancing care coordination, and improving overall patient management and outcomes.
In conclusion, Manuel Joy’s research highlights the transformative potential of agentic workflows in healthcare, showcasing AI’s ability to optimize efficiency, improve clinical outcomes, and reduce provider burnout. Integrating domain-specific models, advanced NLP capabilities, and predictive analytics will further redefine the healthcare landscape as AI technologies evolve. The future of healthcare automation lies in intelligent, adaptive systems that enhance decision-making and streamline operations, ultimately leading to a more efficient, patient-centered healthcare ecosystem.
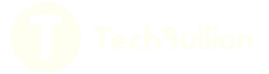