Traditional extraction methods in ETL (Extract, Transform, Load) systems often struggle with the constantly changing formats and sources in today’s digital environments. AI has stepped in to make this phase more intelligent and resilient. Through intelligent source detection, organizations now discover and integrate new data sources significantly faster—often in days instead of weeks. Sudhakar Kandhikonda AI-powered systems also offer adaptive scheduling that optimizes extraction windows in real time, based on source system performance and business priority. In some instances, these algorithms have cut extraction failures by over 70%, responding to source changes within seconds—something traditional systems could never match.
Transformation Reimagined: Machine Learning in Action
The transformation stage, traditionally dominated by rigid, hand-coded rules, has been revitalized by machine learning. AI models now recognize repetitive patterns and suggest or automate data transformations with high precision. This approach not only reduces failures but also slashes the time needed to implement new business logic by nearly half.
Another breakthrough is predictive cleansing, which anticipates data quality issues and applies remediation before they impact downstream processes. These innovations ensure data consistency and integrity across massive, heterogeneous datasets—empowering analytics teams to focus on insights rather than fixing errors.
Intelligent Loading: Strategic Placement with AI
Loading data used to be a straightforward process, but AI has added layers of sophistication. Machine learning algorithms now determine the optimal placement of data across diverse storage environments based on usage patterns, performance needs, and cost efficiency.By employing techniques such as dynamic partitioning and real-time optimization, AI-enhanced loading mechanisms have improved query performance dramatically while reducing storage expenses. This intelligent decision-making transforms the loading phase from a routine task into a strategic operation that directly influences data accessibility and usability.
AI-Powered Governance: Smarter Compliance and Security
Data governance is critical, especially in regulated environments. AI plays a pivotal role in automating compliance through intelligent classification of sensitive information, predictive risk analysis, and anomaly detection in data usage patterns.
Deep learning models now recognize and categorize sensitive data with nearly perfect accuracy, while graph-based lineage tracing uncovers unauthorized access or leaks that might otherwise go unnoticed.
Intelligent Orchestration: Efficiency Through Optimization
AI orchestration engines bring together data processes by dynamically allocating tasks across on-premises and cloud resources. These systems balance performance, cost, and compliance in real time, making thousands of decisions per day to optimize ETL workflows.They predict resource requirements and adjust workloads to current infrastructure conditions, reducing compute costs and ensuring high performance.
A Blueprint for Modern Architectures
A fully AI-enhanced ETL system consists of interlocking components: a rich metadata repository, a machine learning core, real-time monitoring, an orchestration engine, and an adaptive feedback mechanism. Each part feeds into the next, forming a closed-loop system that learns, adapts, and evolves continuously.Metadata acts as the memory of the system, enabling smarter predictions and pattern recognition.
Implementation Realities: Strategy Before Scale
While the technology is promising, successful adoption requires a strategic approach. Organizations that start with high-value, high-pain use cases see the quickest return. Building a robust metadata foundation, implementing feedback loops, and maintaining human oversight where necessary all contribute to higher success rates.
Skill development is also crucial. Teams must be trained not just in AI tools but also in how these tools integrate with domain-specific data needs. Without this dual expertise, the transition to intelligent ETL can fall short of its potential.
Looking Ahead: Toward Autonomous Data Ecosystems
As AI continues to evolve, ETL systems are heading toward autonomy. Reinforcement learning is unlocking new levels of optimization, natural language interfaces are making ETL accessible to non-technical users, and self-organizing pipelines are reshaping data management into a proactive, intelligent ecosystem.
By 2030, many organizations will operate data ecosystems that function with minimal human input, capable of discovering, integrating, and governing data assets autonomously. These developments position AI not as a supporting technology, but as the central engine driving modern data infrastructure.
In conclusion, Sudhakar Kandhikonda’s research illustrates how AI is revolutionizing data integration through smarter, more resilient ETL pipelines. As organizations embrace these innovations, those that align technology with thoughtful implementation strategies will unlock new levels of performance, agility, and insight.
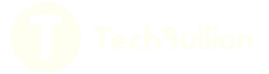