Artificial Intelligence (AI) has already made significant strides across various sectors, and finance is no exception. With the rapid development of machine learning (ML), AI is beginning to reshape the way investment strategies are developed, executed, and optimized. By leveraging vast amounts of data and sophisticated algorithms, AI is enhancing decision-making, improving risk management, and driving better returns for investors. The future of AI in finance is promising, with machine learning at the heart of this transformation. Here’s how AI is revolutionizing investment strategies and what we can expect moving forward.
One key way AI is changing investment strategies is through predictive analytics. AI can process and analyze enormous amounts of historical data, market trends, and economic indicators. Machine learning algorithms use this data to predict future market movements with a level of precision that traditional methods can’t match. As a result, investors can make more informed decisions, reducing risks and improving the chances of generating profits.
AI also allows investors to optimize portfolios by continuously monitoring assets and adjusting investment strategies based on real-time market conditions. This dynamic approach helps maximize returns while managing risks more effectively. For instance, many online platforms offer promotional opportunities like get $200 no deposit bonus 200 free spins real money, which AI-driven algorithms can use to attract new users and increase engagement.
AI and Machine Learning: The Backbone of Modern Investment Strategies
Machine learning, a subset of AI, has proven to be incredibly valuable for investment firms and individual investors alike. By using advanced algorithms to analyze historical data and recognize patterns, AI can predict trends and identify opportunities that would be impossible for a human to discern in a short amount of time. This is especially beneficial in fast-moving markets where timely, data-driven decisions are critical.
One of the primary applications of AI in finance is in portfolio management. AI-powered platforms can continuously assess and rebalance portfolios based on real-time market conditions and individual risk profiles. Machine learning models help to determine optimal asset allocation strategies and fine-tune them as market dynamics shift. This allows investors to adjust their strategies dynamically, ensuring their portfolios stay aligned with their goals without requiring constant manual intervention.
As the algorithms learn from ongoing market data, they also adapt to changing conditions, becoming more accurate over time. This makes machine learning models more robust than traditional investment strategies, where human biases and slower reaction times can limit performance.
Automated Trading Systems: Speed, Efficiency, and Precision
Automated trading systems powered by machine learning have become an essential part of the modern financial landscape. These systems are capable of executing trades at a pace and efficiency far beyond human traders, processing large volumes of data in real-time to make rapid decisions. AI algorithms can analyze multiple factors, such as stock prices, economic data, and geopolitical events, to pinpoint optimal trading opportunities. This level of precision enables quicker, more informed decisions and has transformed the way financial markets operate.
High-frequency trading (HFT) firms exemplify how machine learning enhances algorithmic trading. These firms rely on sophisticated systems that buy and sell assets within fractions of a second. Machine learning models help refine these systems by learning from previous trades, constantly adjusting strategies to adapt to evolving market conditions. This continuous adaptation leads to quicker trade executions, better profitability, and lower risk exposure.
For individual investors, automated trading platforms using AI have become increasingly available. These platforms enable users to automate their trading strategies, utilizing machine learning to execute trades based on pre-set criteria such as market trends or technical indicators. As a result, even amateur investors can benefit from AI’s ability to make rapid, data-backed decisions, creating a more level playing field. Here are a few benefits of automated trading systems:
- Faster Trade Execution: Algorithms can execute trades in fractions of a second, capitalizing on fleeting market opportunities that humans might miss.
- Reduced Emotional Bias: AI eliminates human emotions like fear and greed, allowing for objective, data-driven decisions.
- 24/7 Trading Capabilities: Unlike human traders, automated systems can operate round-the-clock, ensuring constant market analysis and execution.
With AI-powered systems becoming more accessible, even non-professional investors can benefit from the sophisticated technology previously reserved for institutional traders. Automated trading is rapidly becoming an indispensable tool in the financial sector.
Predictive Analytics: Enhancing Investment Decision-Making
Predictive analytics is another area where machine learning is transforming investment strategies. By using historical data to forecast future trends, AI models can offer insights into potential market movements and help investors make better-informed decisions. Machine learning algorithms can predict stock prices, bond yields, or commodity prices by analyzing a wide array of factors such as past performance, economic indicators, and market sentiment.
Investors are increasingly using machine learning to identify patterns in data that may signal an upcoming market shift, helping them to proactively adjust their strategies before major movements occur. Predictive analytics also enhances the ability to detect anomalies, such as potential market crashes or unforeseen events, which can help investors minimize losses by acting early.
In addition, AI-driven predictive tools are allowing investors to fine-tune their risk assessment models. By incorporating data from diverse sources—ranging from social media sentiment to global economic reports—machine learning systems can help predict how various external factors might influence investment outcomes. This results in more informed decision-making and better risk management practices.
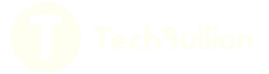