The rapid advancements in Artificial Intelligence (AI) and Machine Learning (ML) have ushered in a new era of language-driven innovations, redefining how businesses and individuals engage with technology. Lohitaksh Yogi, who holds a Bachelor’s in Computer Science, a Master’s in Data Science and Machine Learning, and Product Management Certificates from Stanford University, is a distinguished thought leader in this domain. His global recognition further underscores the impact of his work on AI-driven customer interactions and product innovation.
Mr. Lohitaksh Yogi’s expertise encompasses Product Management (strategic vision, customer centricity, critical decision-making, leadership, user-centric design) and AI (Machine Learning, Deep Learning, NLP, NLU, Large Language Models, Generative AI, RAG, multilingual and multimodal conversational AI.
A Defining Moment in Conversational AI at Adobe
One of the most significant advancements in AI has been the emergence of Large Language Models (LLMs) for conversational applications. Companies such as OpenAI, Google, Adobe, and numerous open-source contributors have demonstrated how transformer-based models (GPT, LLaMA, Bard, etc.) can encode and generate human-like text at scale. These breakthroughs revolutionize customer support, enabling virtual agents to address queries with greater speed and contextual accuracy.
Mr. Lohitaksh Yogi explains that instead of handling intents individually, they created a unified model to process various customer needs simultaneously for a more efficient support experience. Systems using LLMs can interpret user intent in real time, generate relevant suggestions, handle edge cases with minimal drift, and summarize knowledge from multiple documents.
In enterprise settings, deep learning and conversational AI have evolved from rigid FAQ bots to adaptive virtual agents. Typically, the process includes classification, named entity recognition, retrieval-augmented generation (RAG), and feedback loops. Mr. Lohitaksh Yogi emphasizes the importance of generative techniques: “We moved from static FAQ responses to dynamic solutions that integrate multiple knowledge sources.” This enhances user experience and operational efficiency, especially for large organizations managing diverse products and global users.
Challenges in Multimodal and Multilingual AI
While advanced LLMs have enhanced text-based conversational AI, integrating images, voice, and video is becoming crucial. Frameworks like CLIP, BLIP, SILMM, and new Large Multimodal Models combine language with visual or audio inputs for richer experiences.
However, this integration adds complexity, requiring bigger models, specialized training data, and optimized inference. “Expanding AI across languages and modalities posed challenges including model limitations, computational constraints, linguistic nuances, and responsible AI considerations,” Mr. Lohitaksh Yogi states, highlighting the balance between innovation and caution.
Multilingual AI is essential for global enterprises. Cultural norms and language structures challenge universal chatbots, requiring adaptation to each locale. Transformers trained on multilingual datasets help but struggle with rare dialects or less-documented languages. Mr. Lohitaksh Yogi highlights customizing solutions by region: “In Japan, politeness and honorifics are vital; in Western markets, a direct tone works better.” Adapting to cultural nuances enhances accuracy and user trust.
AI-Driven Interactions at Adobe
Industry events like NeurIPS, ICML, and ACL highlight LLM-powered transformations and best practices for AI deployment. Solutions such as Google’s Bard and OpenAI’s GPT-4 show how large-scale training and fine-tuning can enhance product ecosystems. At Adobe, generative AI is vital to creative and support platforms. “We integrated LLMs into Conversational AI and Search, resulting in higher resolution rates, more relevant responses, and a better user experience,” says Mr. Lohitaksh Yogi.
Advanced chatbots improve self-service by interpreting queries, summarizing documents, and providing immediate solutions without users needing to sift through multiple links. Document retrieval systems use RAG to fetch data from large repositories, while LLMs convert this into clear, contextually aligned answers. Mr. Yogi highlights that AI can summarize relevant information directly in search results instead of just linking help articles. These enhancements increase user satisfaction, reduce friction, and unify search experiences across products and support channels.
Retrieval-Augmented Generation (RAG) in Practice
Within the broader optimization strategies for LLMs, one technique that has gained substantial traction is Retrieval-Augmented Generation (RAG). By merging knowledge retrieval from indexed databases with a generative model, RAG systems ensure answers remain grounded in verified sources, minimizing hallucinations. “We used RAG to integrate retrieval-based search with generative AI models, ensuring that user queries were met with precise, knowledge-backed responses rather than purely generative text,” says Mr. Yogi, underscoring how RAG adds an extra layer of reliability to customer-facing applications.
Real-world deployments often use a semantic search backbone—such as BM25 combined with a dense vector model—to locate relevant documents, followed by an LLM that composes a final answer. This pipeline can be further refined with domain-specific datasets to improve retrieval and generation. Higher accuracy and trust are noted benefits as users receive precise, evidence-backed answers, leading to increased trust in AI responses. The combination of retrieval and generation enhances user experiences, allowing businesses to expand AI solutions across various areas (customer support, knowledge management, content creation) with minimal risk of misinformation.
Omnichannel AI Assistants
Looking ahead, experts anticipate significant advancements in omnichannel AI assistants, propelled by generative AI innovations, multimodal capabilities, and enhanced personalization. As large models become more efficient, they will be able to process text, images, or voice inputs in real time, facilitating seamless cross-platform interactions—whether through a messaging application, a virtual reality interface, or a smart speaker. “AI will comprehend and generate human-like responses across text, voice, video, and image inputs,” Mr. Yogi predicts, emphasizing the transition from static chatbots to more dynamic, immersive experiences.
These future assistants will increasingly leverage Retrieval-Augmented Generation (RAG) to utilize enterprise and user-level context, integrating historical conversation logs, database queries, and real-time analytics. Large or specialized organizations will be able to consolidate multiple data sources—such as Customer Relationship Management (CRM) systems, event streams, and product manuals—into a singular AI orchestrator. Mr. Yogi adds, “AI will recall user preferences with consent across various touchpoints (chat, email, mobile, IoT).” This continuity enables enterprises to deliver consistent, proactive engagements on any device, positioning AI as an integral component of daily workflows.
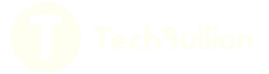