Artificial Intelligence (AI) has evolved to offer highly personalized experiences, Swapnil Hemant Thorat, in his latest research, explores groundbreaking frameworks that enable personalization in Large Language Models (LLMs) without compromising security. His study introduces innovative techniques that pave the way for a privacy-conscious digital future.
Federated Learning: Decentralized Personalization
Traditional AI personalization methods require central data storage, which poses privacy risks. Federated learning, an emerging technique, ensures model training occurs directly on user devices rather than on centralized servers. This decentralized approach not only enhances data security but also retains AI adaptability. By leveraging edge computing and secure execution environments, federated learning provides a scalable solution for privacy-preserving personalization.
Additionally, federated learning reduces bandwidth usage by transmitting only model updates rather than raw data. It enables real-time personalization while respecting increasingly stringent global privacy regulations. The approach addresses computational heterogeneity across devices through adaptive optimization techniques. As IoT expansion continues, federated learning represents a promising framework that balances personalization needs with fundamental privacy rights, potentially revolutionizing sectors like healthcare and finance where data sensitivity is paramount.
Voice Biometric Authentication: The Future of Secure Access
Passwords and PINs are increasingly vulnerable to security breaches, making biometric authentication a promising alternative. Voice biometric systems create unique voiceprints for users, allowing seamless and secure access to AI-powered services. These systems continuously refine user authentication without requiring frequent manual logins. However, strong encryption and liveness detection mechanisms are essential to prevent misuse and spoofing attempts.
Voice biometrics also offer accessibility advantages for users with physical limitations or visual impairments. The technology’s non-invasive nature increases user adoption compared to other biometric methods. Recent advances in deep learning have significantly reduced false acceptance and rejection rates, enhancing reliability. Multi-factor authentication approaches that combine voice with other biometrics create layered security that’s nearly impossible to breach. As voice-enabled devices proliferate in smart homes and vehicles, voice authentication provides a natural interface that balances convenience with robust security protocols while respecting user privacy preferences.
Differential Privacy: Protecting Sensitive Information
One of the core concerns in AI personalization is data exposure. Differential privacy introduces a mathematical model that adds noise to datasets, ensuring individual data points remain untraceable while still allowing AI to learn effectively. This technique strikes a balance between data utility and privacy, making it an essential tool in privacy-first AI development.
The integration of differential privacy into machine learning pipelines provides formal privacy guarantees with quantifiable privacy budgets. Organizations can now precisely control privacy-utility tradeoffs through epsilon parameters that regulate noise injection. Modern implementations incorporate adaptive mechanisms that automatically adjust privacy levels based on data sensitivity and context. Differential privacy also enables cross-organizational collaboration on sensitive datasets through privacy-preserving analytics. As regulatory frameworks like GDPR and CCPA intensify scrutiny of data practices, differential privacy offers a mathematically rigorous foundation for ethical AI systems that protect individual rights while enabling innovation.
Secure Processing Environments: Enhancing AI Trustworthiness
AI systems often process vast amounts of user data, making them susceptible to breaches. Secure enclaves and Trusted Execution Environments (TEEs) create isolated spaces for sensitive computations, preventing unauthorized access—even from system administrators. This hardware-based security model reinforces trust in AI applications, ensuring data remains confidential throughout processing.
Adaptive User Profiling: Dynamic and Privacy-Aware
User profiles drive AI personalization, but storing them centrally increases privacy risks. A hierarchical profile structure enables AI to customize responses while maintaining user anonymity. Additionally, dynamic profile switching allows systems to adapt based on contextual changes without permanently storing personal data. These advancements ensure AI remains responsive while safeguarding user identities. Local encryption further strengthens protection against unauthorized access to sensitive personalization data.
In conclusion,As AI technology progresses, maintaining a balance between customization and privacy remains crucial. Innovations such as federated learning, voice biometrics, and differential privacy provide a blueprint for a future where AI can deliver tailored experiences without infringing on user rights. Researchers, developers, and policymakers must collaborate to refine these technologies, ensuring AI remains both intelligent and ethical, Swapnil Hemant Thorat’s work serves as a significant contribution to this evolving field, highlighting practical solutions that can reshape AI personalization for a privacy-focused world.
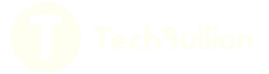