As AI reshapes industries, businesses are leveraging Large Language Models (LLMs) and structured documentation to enhance technical support and self-service systems. This fusion could bridge knowledge gaps, streamline customer interactions, and revolutionize user experience, ensuring faster, more accurate responses in an increasingly digital world.Rakesh Konda, a researcher in artificial intelligence and structured documentation, explores an innovative approach that integrates structured documentation systems with Large Language Models (LLMs). His insights reveal how this synergy enhances content management, improves customer experiences, and paves the way for intelligent self-service solutions.
The Evolution of Structured Documentation
Technical documentation has long relied on structured frameworks to ensure clarity, consistency, and reusability. A notable advancement in this domain is the Darwin Information Typing Architecture (DITA), which provides a modular approach to content creation. By segmenting information into self-contained topics, DITA enables organizations to maintain consistency while facilitating easier updates and localization.
The Rise of Large Language Models in Customer Support
LLMs have revolutionized artificial intelligence with their ability to process and generate human-like text. Trained on vast datasets, these models excel in natural language understanding, making them ideal for applications in customer support and self-service systems. Organizations have adopted LLM-powered chatbots, automated ticketing systems, and AI-driven knowledge bases to enhance user interactions.
While LLMs offer impressive capabilities, they also present challenges such as hallucination (generating incorrect information), contextual misinterpretation, and biases from training data. These limitations necessitate a structured approach to content integration to ensure reliability and accuracy in AI-generated responses.
Bridging the Gap: Integrating Structured Documentation with AI
The integration of structured documentation with LLMs creates a powerful synergy that enhances the accuracy and efficiency of AI-driven customer support. This approach involves several key steps:
- Content Extraction: Structured documentation is extracted and formatted to serve as a reliable knowledge base for AI models.
- Data Transformation: The content is converted into a format suitable for LLM training while preserving semantic relationships.
- AI Integration: A middleware layer facilitates seamless communication between the structured content repository and the AI model.
- Response Validation: AI-generated responses are cross-referenced with structured documentation to ensure accuracy and consistency.
- Continuous Feedback Loop: User interactions provide valuable feedback, allowing for iterative improvements in both documentation and AI performance.
This structured approach mitigates common AI challenges, providing more reliable, context-aware.
Advantages of an AI-Enhanced Documentation System
The convergence of structured documentation with AI presents several key benefits:
- Improved Accuracy: AI responses are more reliable when trained on structured, high-quality content.
- Scalability: Organizations can efficiently scale multilingual and multi-regional support using AI-powered documentation systems.
- Cost Reduction: Automated responses to common queries reduce the workload on human agents, leading to significant cost savings.
- Personalized Support: AI can tailor responses based on user history and preferences, delivering more relevant and engaging interactions.
Challenges and Considerations
Despite its advantages, the integration of structured documentation with AI presents several challenges:
- Data Privacy and Security: Organizations must adhere to regulations to ensure secure handling of customer data.
- Content Quality Control: AI-generated responses must align with structured documentation, requiring continuous monitoring and validation.
- Balancing Automation with Human Expertise: AI should complement, rather than replace, human support, ensuring seamless escalation for complex queries.
The Future of Intelligent Self-Service Systems
Looking ahead, AI-enhanced documentation and self-service systems will continue to evolve. Key trends shaping the future include:
- Predictive Support: AI will proactively anticipate user needs, offering solutions before issues arise.
- Multi-Modal Learning: AI will integrate text, images, and videos for more comprehensive support solutions.
- Emotional Intelligence: AI-driven support systems will incorporate sentiment analysis to provide empathetic and contextually appropriate responses.
As organizations embrace these advancements, they will redefine customer engagement ,ensuring more intelligent and responsive self-service ecosystems.
In conclusion, the integration of structured documentation with LLMs marks a transformative shift in technical communication and customer support. By leveraging modular content with AI-driven insights, organizations can enhance accuracy, scalability, and personalization while reducing operational costs. Though challenges such as data security and content governance persist, the potential benefits far outweigh the hurdles. As highlighted by Rakesh Konda, this innovative approach represents a promising future for AI-enhanced self-service systems, redefining the way businesses interact with their customers.
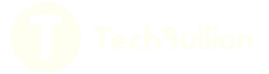