In the age of innovation, Artificial Intelligence (AI) has transcended its role as a mere automation tool to become an indispensable collaborator in industries where efficiency and adaptability are paramount. Rajarshi Tarafdar, a thought leader in AI-driven workflow optimization, presents a groundbreaking framework that redefines human-AI collaboration in decision-making processes. His research highlights how AI can enhance operational efficiency while preserving human oversight, paving the way for more intelligent, responsive, and resilient business ecosystems.
Beyond Automation: The Role of AI as a Collaborative Partner
Traditional automation has been instrumental in streamlining processes, yet fully automated systems often struggle with adaptability, ethical judgment, and contextual understanding. Human-AI collaboration bridges this gap, ensuring that AI assists rather than replaces human decision-makers. This approach optimizes workflows in industries such as manufacturing, logistics, and financial services, where complex decision-making and adaptability are crucial.
The Collaborative Workflow Intelligence Framework (CWIF)
The proposed Collaborative Workflow Intelligence Framework (CWIF) establishes structured information flows between AI and human operators. The framework consists of four key layers:
- Perception Layer – Gathers and processes operational data.
- Analysis Layer – Utilizes AI and human expertise to identify inefficiencies.
- Suggestion Layer – Converts insights into actionable recommendations.
- Implementation Layer – Ensures smooth execution of decisions with human oversight. This structured interaction between AI and humans results in an adaptive system capable of improving over time.
Enhancing Manufacturing with AI-Assisted Decision-Making
AI enhances manufacturing by optimizing production scheduling, quality control, and energy use through real-time data analysis. It suggests workflow improvements, while human expertise refines decisions. AI-driven vision systems detect defects, with human inspectors handling complex classifications and process adjustments.
Optimizing Logistics through AI-Driven Adaptability
Logistics operations rely on real-time decision-making, and AI can significantly enhance efficiency by optimizing supply chain management, route planning, and inventory allocation. AI-powered advisors analyze demand forecasts and transportation constraints, generating recommendations that human supply chain managers refine with their strategic insights. This symbiotic relationship ensures smooth operations even in unpredictable conditions.
Financial Services: AI for Risk Management and Compliance
The financial sector leverages AI-human collaboration in areas such as fraud detection, credit risk assessment, and transaction processing. AI models process vast datasets to identify potential risks, while human experts evaluate AI-generated insights for strategic decision-making. Ensuring transparency and regulatory compliance is crucial, making human oversight a necessary component in AI-assisted financial workflows.
Establishing Effective Decision Authority Boundaries
A crucial aspect of AI-human collaboration is defining clear decision authority boundaries. The CWIF proposes four models:
- Human Authority with AI Input – AI provides data-driven insights, but humans retain full decision-making power.
- Human Authority with Explained AI Recommendations – AI suggests options with transparent explanations, allowing humans to make informed choices.
- AI Authority with Human Veto – AI makes autonomous decisions, but human intervention is possible if needed.
- Delegated AI Authority – AI operates independently within predefined constraints, optimizing routine tasks. By structuring these decision-making roles, AI and humans work together effectively, maximizing efficiency while ensuring ethical considerations are upheld.
Challenges and Solutions in AI Integration
Despite the advantages of AI-human collaboration, challenges such as data integration, workforce adaptation, and regulatory compliance persist. Addressing these requires:
- Modular system architectures for seamless AI integration into existing workflows.
- Transparent communication to mitigate workforce concerns regarding job displacement.
- Training programs that equip employees with skills to interact with AI-driven systems effectively. A phased implementation approach, starting with advisory AI models before progressing to full automation, ensures smoother adoption.
The Future of AI-Driven Workflow Optimization
The future of AI collaboration extends beyond specific industries. With advancements in adaptive learning, AI systems will become more responsive to human expertise, refining recommendations based on continuous feedback. As organizations implement AI-assisted decision-making frameworks, the emphasis will remain on enhancing human capabilities rather than replacing them.
In conclusion, the research of Rajarshi Tarafdar paves the way for a transformative shift in workflow optimization, where AI serves as an intelligent collaborator rather than just a tool for automation. By fostering a balance between operational efficiency and human expertise, organizations can achieve sustainable improvements in productivity, innovation, and decision-making. This approach not only enhances immediate business outcomes but also ensures long-term adaptability in an increasingly dynamic technological landscape.
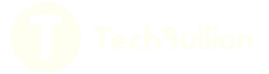