In the modern digital transformation, only enterprises that harness the power of Artificial Intelligence (AI) can truly optimize their data management strategies. AI is revolutionizing Extract, Transform, Load (ETL) processes by introducing automation, intelligence, and adaptability, leading to enhanced efficiency, accuracy, and scalability. Independent researcher Gaurav Naresh Mittal explores the growing influence of AI in ETL workflows, demonstrating how AI-driven solutions are reshaping the way enterprises extract insights, improve data quality, and accelerate decision-making. As businesses deal with increasingly complex and voluminous datasets, AI-driven ETL is becoming a critical enabler of seamless, real-time data processing.
Intelligent Data Discovery and Unstructured Data Processing
Modern enterprises deal with an overwhelming volume of data, much of which is unstructured. AI-driven intelligent data discovery systems are revolutionizing data classification and retrieval by automating the identification and categorization of complex data formats. These systems significantly improve accuracy while reducing manual efforts. Additionally, Natural Language Processing (NLP) technologies have made it possible to convert unstructured data into actionable insights, improving data usability across industries.
Adaptive Web Scraping for Real-Time Data Collection
Data extraction is a critical part of ETL processes, and AI-driven adaptive web scraping solutions have emerged as a game-changer. These systems can automatically adjust to website structure changes, ensuring continuous and accurate data retrieval. By reducing manual intervention and enhancing extraction accuracy, businesses can maintain up-to-date and high-quality datasets.
AI-Powered Data Transformation: Cleansing and Smart Mapping
Data transformation has traditionally been a resource-intensive process. AI automates data cleansing by detecting and correcting anomalies, leading to significant improvements in data accuracy and consistency. Furthermore, AI-driven smart mapping tools streamline schema detection and field mapping, drastically reducing the time and effort required for data integration.
Real-Time Monitoring for Enhanced Data Quality
Ensuring data quality is a continuous challenge for enterprises. AI-powered real-time monitoring systems can identify and rectify inconsistencies within seconds, preventing data quality issues before they affect downstream processes. These systems not only improve data integrity but also optimize resource utilization by reducing computational overhead.
Optimized Data Loading with Intelligent Scheduling
AI is enhancing data loading through intelligent scheduling algorithms that optimize batch processing. These solutions dynamically allocate resources based on real-time conditions, reducing bottlenecks and improving processing speeds. As a result, enterprises benefit from lower operational costs and improved system performance.
Adaptive Performance Tuning for Big Data Workloads
Handling massive datasets efficiently requires adaptive strategies. AI-driven MapReduce optimization has significantly enhanced big data processing by dynamically adjusting resource allocation and predicting workload demands. These advancements allow enterprises to process vast amounts of data with reduced latency and improved cost efficiency.
Self-Healing Capabilities: Automated Error Recovery
AI-powered self-healing capabilities have transformed system reliability. These systems can detect and diagnose failures within seconds and initiate automated recovery procedures. By reducing manual intervention, enterprises can achieve near-continuous uptime and improved operational efficiency.
Predictive Maintenance for Infrastructure Optimization
Enterprises are leveraging AI-driven predictive maintenance to minimize system failures and optimize infrastructure utilization. By analyzing historical data and identifying patterns, AI can predict potential issues before they occur, reducing downtime and maintenance costs while extending the lifespan of critical systems.
AI-Driven Cloud Resource Optimization
With cloud computing playing a central role in enterprise operations, AI is ensuring optimal resource allocation. AI-powered solutions analyze workload patterns to adjust cloud resource provisioning dynamically, reducing costs and enhancing system efficiency. These technologies prevent resource wastage while ensuring peak performance across cloud environments.
The Future of AI-Enhanced ETL
The integration of AI in ETL processes is enabling enterprises to achieve unprecedented levels of efficiency and scalability. By automating critical data processing tasks, AI enhances data quality, accelerates processing times, and optimizes infrastructure utilization. As businesses continue to embrace digital transformation, AI-powered ETL solutions will remain a cornerstone of enterprise data management.
In conclusion, Gaurav Naresh Mittal’s research underscores the undeniable impact of AI on modern ETL systems. As AI continues to evolve, enterprises that embrace these innovations will gain a significant competitive advantage in managing their ever-growing data landscapes. By leveraging AI-driven automation, intelligent data processing, and real-time insights, organizations can enhance efficiency, improve decision-making, and future-proof their data strategies in an increasingly complex digital environment.
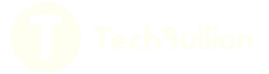