AI has transformed industries from finance to healthcare, yet trucking—one of the most vital links in the global supply chain—remains resistant to full-scale automation. If AI can predict financial markets and diagnose diseases, why is autonomous trucking still stuck in limited trials? The roadblocks are both technical and systemic: AI must navigate unpredictable conditions, integrate with human-driven fleets, comply with evolving regulations, and earn the trust of an industry built on precision. Until these challenges are conquered, autonomous trucking will remain a stalled revolution rather than an unstoppable force reshaping logistics.
Bridging the Gap Between AI and Real-World Logistics
AI has the potential to revolutionize freight movement, but its adoption hinges on bridging the gap between research and execution. The challenge isn’t just developing cutting-edge AI models—it’s ensuring they function seamlessly in high-stakes, real-world logistics.
To talk more on this, we turned to Yashovardhan Chaturvedi, a seasoned ML engineer with over a decade of experience. A Senior IEEE member, and ML Engineer II at Torc Robotics, he has played a key role in shaping AI-driven freight solutions. His expertise in developing scalable AI applications enhances efficiency and safety while tackling real-world uncertainties. By pushing AI beyond research labs into practical deployment, Yashovardhan is redefining autonomous freight management.
“AI in logistics goes beyond automation—it’s about building a system that continuously learns, adapts, and evolves with time,” Yashovardhan explains. “From route optimization to predictive maintenance, machine learning models must continuously evolve to handle the complexities of modern freight transport.”
His expertise lies in building scalable AI applications that bridge the gap between research and execution. By integrating machine learning with sensor-driven analytics, he is working toward enhancing vehicle perception, improving predictive analytics, and ensuring seamless AI-human collaboration in freight management. “The real challenge lies in building AI models that aren’t just technically advanced but resilient enough to perform reliably in unpredictable, high-stakes environments,” he adds.
AI in Action: Transforming Logistics with Data-Driven Intelligence
A study by the Singapore Land Transport Authority (LTA) found that AI-driven traffic systems led to a 15% reduction in congestion and a 20% improvement in road safety. Meanwhile, logistics firms leveraging AI-powered fleet management have reported a 30% increase in fuel efficiency and a 25% reduction in vehicle downtime, proving AI’s direct impact on optimizing freight operations.
Refining AI for real-world logistics requires more than just innovation—it demands the ability to handle unpredictability at scale. From traffic congestion to adverse weather, unforeseen obstacles continue to challenge full autonomy in trucking. “AI in logistics must be designed for the unexpected,” says Yashovardhan. “An algorithm that works perfectly in a controlled test environment is useless if it can’t adapt to real-world disruptions like sudden lane closures or erratic driver behavior.”
Adaptive learning is closing this gap, enabling AI systems to process live data, recognize patterns, and make real-time adjustments. As AI-powered logistics platforms grow more responsive, their ability to ensure efficiency and safety in unpredictable conditions becomes a defining factor in their adoption.
Smarter Logistics for a More Sustainable Future
AI is not only improving logistics efficiency but also driving sustainability in the process. Smarter route planning reduces fuel consumption, and predictive maintenance prevents costly breakdowns. In one notable case, AI-driven logistics solutions helped a major freight company cut carbon emissions by 18% and reduce idle time by 40%, showcasing AI’s ability to drive sustainability
Yashovardhan’s groundbreaking contributions earned him the ICMR-IITM award for developing adaptive routing systems that optimize fuel usage while maintaining delivery efficiency. Companies integrating AI-driven fleet management are reporting measurable reductions in carbon footprints, reinforcing AI’s critical role in creating an environmentally responsible transportation sector.
Addressing the Roadblocks to AI Adoption in Trucking
The skepticism around machine learning in logistics isn’t unfounded. Just as automated trading once faced resistance from traditional finance, autonomous trucking grapples with similar doubts. A 2023 MIT report highlighted that while 79% of logistics executives believe AI is critical to the industry’s future, only 32% have implemented AI-driven solutions at scale. The reluctance stems from regulatory uncertainty, integration challenges, and concerns over job displacement. Yet history shows that industries resisting technological evolution eventually fall behind.
“The industry is at an inflection point,” Yashovardhan notes. “Logistics firms must decide whether to lead AI adoption or scramble to catch up later.” As an ESP Journal Board Member, he emphasizes that AI isn’t a futuristic concept—it’s already optimizing deliveries, reducing emissions, and cutting operational costs. The real question is: will companies act now, or will they wait until AI-driven competitors force their hand?
The logistics industry is at a crossroads. Companies that hesitate risk falling behind, while those that embrace AI-driven innovation will lead the new times of transportation. AI in logistics is no longer an experiment—it’s an urgent necessity. The time for pilot programs and hesitant trials is over. The freight industry stands at a pivotal moment—companies that take bold steps toward AI-driven logistics today will shape the future of transportation for years to come.
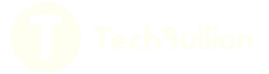