In an era when data fuels innovation and drives critical decision-making, maintaining the integrity of machine learning systems is paramount. Dr. Mohan Raja Pulicharla, a visionary researcher in the field of artificial intelligence, has unveiled a groundbreaking study entitled ML-Synergy for Data Drift Management: A Multi-Agent System for Proactive Monitoring, Alerting, and Auto-Correction of Data Pipelines. This pioneering work is poised to revolutionize how organizations handle the challenges posed by data drift, ensuring that their predictive models remain robust and reliable.
A New Paradigm in Data Integrity
As businesses increasingly rely on machine learning to power everything from customer service chatbots to financial forecasting models, the phenomenon known as “data drift” has emerged as a critical vulnerability. Data drift occurs when the statistical properties of incoming data begin to deviate from those of the training data, potentially undermining the performance of deployed models. Recognizing this challenge, Dr. Pulicharla’s research introduces a multi-agent system designed to not only detect these shifts but also to proactively manage and correct them.
“Traditional methods of addressing data drift have largely been reactive, addressing issues only after they manifest in performance degradation,” explains Dr. Pulicharla. “Our approach with ML-Synergy is to anticipate these shifts and mitigate their effects in real time, ensuring continuous operational excellence.” His innovative solution leverages the collective intelligence of multiple agents, each programmed to handle specific tasks—from monitoring data streams and detecting anomalies to initiating automatic corrective measures.
The Multi-Agent System: How It Works
At the core of ML-Synergy is a sophisticated framework that deploys an ensemble of intelligent agents across data pipelines. These agents work in unison to monitor vast and complex data environments, using state-of-the-art statistical methods to detect deviations from established patterns. When an anomaly is identified, the system immediately triggers a cascade of alerts, allowing for swift intervention. In many instances, the system is capable of initiating auto-correction protocols that recalibrate the data flow without human intervention.
The multi-agent architecture is highly modular, meaning it can be scaled to fit the needs of small start-ups as well as large, enterprise-level operations. “One of our main goals was to ensure that ML-Synergy is versatile enough to be implemented in a wide range of industries, from finance and healthcare to retail and logistics,” Dr. Pulicharla remarks. This scalability is achieved through a flexible design that can be customized to monitor and manage specific data streams unique to different operational contexts.
Industry Impact and Early Adoption
The implications of this research are far-reaching. In industries where data is not only vast but also constantly evolving, even minor disruptions can have significant operational and financial consequences. By preemptively identifying and addressing data drift, ML-Synergy minimizes the risk of erroneous outputs, ensuring that machine learning models continue to deliver accurate and actionable insights.
Early adopters have already reported promising results. Several multinational corporations are currently piloting ML-Synergy in their data centers, noting significant improvements in model performance and a reduction in downtime. “The introduction of a proactive monitoring and correction system is a game-changer,” says Anita Desai, Chief Data Officer at a leading financial institution. “It transforms data management from a reactive chore into a dynamic process of continuous improvement.” These early testimonials underscore the potential of Dr. Pulicharla’s work to reshape data governance practices across multiple sectors.
Bridging the Gap Between Research and Real-World Application
While the theoretical underpinnings of ML-Synergy are robust, its real-world application is what truly sets it apart. Dr. Pulicharla’s research not only delves into the complexities of multi-agent systems but also provides practical guidelines for implementing them in live environments. This dual focus on innovation and application has made the study a touchstone for both academic researchers and industry practitioners.
“By bridging the gap between theoretical research and practical deployment, Dr. Pulicharla has set a new standard for data management in the age of AI,” comments Professor Elena Martinez, a noted expert in data science at the Institute of Technology. “His work demonstrates that with the right tools and strategies, we can build systems that are not only intelligent but also resilient to the challenges posed by ever-changing data landscapes.”
The Future of Proactive Data Management
The introduction of ML-Synergy marks a significant milestone in the ongoing evolution of data management technologies. Its proactive approach is not merely a stopgap solution but a forward-thinking strategy designed to anticipate and adapt to future challenges. As machine learning models become even more integral to business operations, the ability to maintain data fidelity will be critical to sustaining competitive advantage.
Dr. Pulicharla envisions a future where data pipelines operate autonomously, with minimal human intervention required. “Imagine a world where our systems are self-regulating—constantly learning, adapting, and correcting themselves without the need for manual oversight,” he muses. Such a vision is not only inspiring but also achievable with innovations like ML-Synergy. By continuously refining its algorithms and expanding its agent network, the system is set to evolve alongside the very data it monitors.
Ethical Considerations and the Road Ahead
As with any significant technological advancement, the deployment of a highly automated system raises important ethical questions. One key concern is the balance between automation and human oversight. While ML-Synergy is designed to operate autonomously, Dr. Pulicharla emphasizes that it is meant to complement rather than replace human expertise. “The goal is to empower data professionals, not to render their skills obsolete,” he states. “Human judgment remains critical, particularly in scenarios where context and nuanced understanding are required.”
This commitment to ethical implementation is a cornerstone of Dr. Pulicharla’s research philosophy. He advocates for a collaborative model where machine intelligence and human insight work in tandem to achieve superior outcomes. By ensuring transparency and accountability in automated decision-making processes, ML-Synergy sets a new benchmark for ethical AI practices in the data management domain.
Looking Forward: Collaborations and Innovations
The excitement surrounding ML-Synergy extends beyond immediate practical benefits. Academic and industry collaborations are already underway to explore additional applications of multi-agent systems. Research teams are investigating how similar frameworks can be adapted for cybersecurity, network optimization, and even real-time decision support in critical infrastructure.
Dr. Pulicharla’s work is generating a ripple effect of innovation. “We are just scratching the surface of what multi-agent systems can achieve,” he notes. “The principles behind ML-Synergy have the potential to transform not only data management but many other aspects of technology and industry.” As the research community builds on these ideas, the next wave of innovations is expected to further enhance the resilience and efficiency of our digital ecosystems.
Conclusion
In a world where data is both a vital asset and a potential liability, Dr. Mohan Raja Pulicharla’s ML-Synergy stands out as a beacon of innovation. By proactively monitoring, alerting, and auto-correcting data pipelines, this multi-agent system addresses one of the most pressing challenges facing machine learning today—data drift. Its blend of cutting-edge research, practical application, and ethical foresight heralds a new era in data management, one where systems are not just reactive, but intelligently anticipatory.
As organizations across the globe strive to harness the full potential of their data, the impact of ML-Synergy will undoubtedly be profound. With early successes already sparking widespread interest, the future of proactive data management looks brighter than ever—thanks to the pioneering vision of Dr. Mohan Raja Pulicharla.
Full Research article is available at: https://ijmrset.com/upload/18_ML%20Synergy.pdf
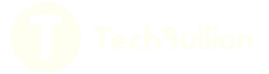