In early 2023, while enterprises raced to explore generative AI, seasoned AI Architect Pavan Emani was focused on something far less visible, the data foundation beneath it all.
With nearly two decades of experience in data and machine learning architecture, Mr. Emani has helped Fortune 500 companies and financial institutions modernize data platforms and scale AI systems. His central belief: without disciplined data infrastructure, generative AI cannot succeed at scale.
“The real differentiator isn’t the model,” says Mr. Emani. “It’s the integrity, governance, and structure of the data underneath.”
Generative AI Is Not Plug-and-Play
Mr. Emani cautions against the popular notion that generative AI tools can be easily layered onto existing systems. In practice, many pilots fail because of poor data quality, lack of lineage, and siloed infrastructure.
A 2022 Gartner report projected that 80% of AI projects would fail to scale, primarily due to inadequate data foundations. Mr. Emani has seen this firsthand: models built on fragmented or stale data often produce irrelevant or misleading outputs.
“You can’t automate intelligence when you can’t trust your inputs,” he notes.
Beyond Centralization: A Case for Data Mesh
Mr. Emani’s work at companies like Amazon Web Services (AWS) and Bank of America exposed him to complex, large-scale data environments. There, he became a strong proponent of the Data Mesh paradigm, which decentralizes data ownership and promotes domain-level stewardship.
In 2022, he published technical guidance for financial institutions adopting Data Mesh on the cloud, arguing that the model enables federated responsibility and scalable AI adoption. In one case, a firm reduced AI project delivery time by 40% after restructuring its data teams around this model.
“When data is owned by the teams closest to the business, it becomes more useful, more accurate, and better aligned to AI use cases,” Mr. Emani explains.
The Myth of “More Data”
Another misconception Mr. Emani pushes back on is that more data equals better models. In enterprise settings, this often leads to bloated pipelines filled with outdated or uncurated data.
“It’s not about volume, it’s about context,” he says. “Training a model on ten years of inconsistent records won’t help if only the last 18 months are relevant.”
He points to the 2023 Stanford Foundation Model Transparency Index, which found that curated, context-aware datasets outperformed larger, unfiltered datasets in enterprise scenarios. Mr. Emani’s own work confirms this: in multiple cases, focused fine-tuning outperformed brute-force scaling.
Aligning Data Strategy with AI Objectives
One structural issue Mr. Emani frequently sees is a disconnect between data and AI strategy. Often, data governance sits with operations, while AI innovation is driven by separate tech teams,leading to misalignment, duplication, and regulatory risk.
Mr. Emani recommends embedding shared accountability across the AI development lifecycle. That means linking SLAs, lineage, and governance directly into model design and deployment cycles.
His approach aligns with findings from the World Economic Forum’s 2023 AI Governance report, which emphasized that AI systems must include traceability and transparent data sourcing to maintain trust.
Start with the Plumbing, Not the Pilot
Despite the market’s focus on LLMs, co-pilots, and chatbots, Mr. Emani’s advice remains grounded: begin with your data architecture.
“Don’t chase Gen AI without fixing the basics,” he advises. “If your teams don’t trust your data warehouse, adding a model on top won’t help.”
In one engagement, Mr. Emani helped unify siloed customer data pipelines for a financial firm. Once lineage and access controls were standardized, the firm’s generative AI engine produced accurate, personalized recommendations,where previous versions had failed.
“The improvement didn’t come from a better model. It came from cleaner data,” he notes.
A Voice of Practical Innovation
Mr. Emani is known not only for his technical depth, but also for helping enterprises translate AI ambition into business impact. He has published technical blogs on cloud-native AI, mentored professionals across industries, and served as a judge for startup competitions and enterprise tech awards.
In every forum, he emphasizes the same point: AI must be grounded in systems that are measurable, governable, and aligned to outcomes.
“The hype will fade. What will remain are the platforms and patterns we build today,” Mr. Emani says. “If the data layer is fractured, the AI layer will be fragile.”
About the Profiled Leader:
Pavan Emani is a senior architect with close to 20 years of experience in data and machine learning systems. He has led large-scale transformation initiatives at major global firms, published technical guidance on AI engineering, and continues to advise organizations on building responsible, scalable AI systems.
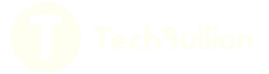