In the age of digital transformation, data is no longer just a business asset—it is the backbone of modern enterprises. As companies adopt more sophisticated technologies like artificial intelligence (AI) and machine learning (ML), the integration of robust data security and advanced analytics becomes critical to both protecting sensitive information and extracting actionable insights. Dilip Rachamalla, Senior IEEE Member and a Sr. Software Engineer at Intuit, has been at the forefront of leveraging data security protocols and analytics frameworks to drive operational efficiencies and innovation in the data-driven landscape. With over 16 years of experience in AI, data engineering, and cybersecurity, Rachamalla is regarded as a leader in shaping the future of data governance and analytics.
Rachamalla’s approach to data security and analytics centers around two primary objectives: securing the data infrastructure and ensuring it is optimized for high-performance analytics. “Data is the foundation upon which businesses are built, but without a robust security framework, this data becomes vulnerable to breaches that can undermine its value,” he explains.
The Convergence of Data Security and Advanced Analytics
Data analytics and security are intrinsically linked. Effective data analytics relies on data integrity and confidentiality, which can only be achieved by a comprehensive data security strategy. At Intuit, Rachamalla has played a pivotal role in designing scalable data security architectures that ensure sensitive data is not only secure but also ready for high-level analytics. “Data security is no longer just about perimeter defense—it’s about integrating security protocols within every aspect of the data lifecycle,” he asserts.
He explains that businesses leveraging AI-powered analytics platforms need to ensure the data feeding into these systems is secure and trustworthy. Without proper governance, any analysis derived from compromised data would be fundamentally flawed, leading to poor decision-making and potential regulatory issues. By employing data encryption, multi-factor authentication, and real-time anomaly detection, organizations can protect their data while enabling high-value analytics.
Rachamalla also highlights how AI and ML algorithms can bolster data security measures. For instance, machine learning models can be used to automatically detect security breaches by identifying anomalous patterns in data usage or access, thus preventing potential vulnerabilities from escalating into major issues. “AI-driven security systems are increasingly capable of autonomous monitoring, which reduces the time to identify and mitigate risks,” he notes.
As a judge at the Globee Awards for AI, Rachamalla leverages his deep understanding of both AI and data security to evaluate breakthrough innovations in these areas. His expertise in balancing security with performance makes him uniquely qualified to assess how emerging technologies can transform the security landscape while enabling organizations to leverage analytics at scale.
Data Security as a Foundation for Scalable Analytics
In a highly interconnected world, maintaining data integrity while ensuring accessibility for advanced analytics is crucial. “You can’t analyze data if it’s not secured properly. A compromised system not only risks the integrity of your data but also the efficacy of the insights drawn from it,” Rachamalla explains.
In his role at Intuit, Rachamalla has implemented data architectures that emphasize both security and analytics. By designing systems that compartmentalize and protect sensitive data, his teams ensure that advanced analytical tools can perform without hindrance. For example, by using encryption algorithms and securing access points, the organization’s analytics teams can query data at scale, all while maintaining stringent data privacy controls.
Rachamalla also advocates for the adoption of zero-trust security models within organizations. This framework is particularly effective when combined with AI-driven analytics, as it ensures that only authorized users and systems can access specific datasets. “In an AI-driven environment, trust is not given—it must be earned. Zero-trust models provide that necessary framework,” he asserts.
AI-Driven Data Security and Analytics
Looking to the future, Rachamalla predicts that AI and machine learning will play an increasingly prominent role in the automation of data security protocols. “We are heading toward a future where AI will not only optimize data workflows but also continuously monitor and fortify data security in real-time,” he states.
Rachamalla emphasizes the potential of AI to not only automate security but also make data security systems more adaptive and intelligent. He envisions AI algorithms identifying evolving security threats and adjusting security measures dynamically, preventing potential breaches before they happen.
Moreover, as organizations continue to rely on data-driven decision-making, the convergence of AI, data security, and analytics will enable businesses to gain deep, predictive insights while mitigating the risks of data misuse. Rachamalla believes this integration will create a new era of intelligent data governance, where businesses can make faster, more informed decisions while maintaining the highest levels of security.
In addition to his corporate impact, Rachamalla continues to shape global business strategies and contribute to the data community via research papers on scholarly publications where he has authored papers on data engineering synergies. His deep expertise in these domains enables him to contribute to the selection of technologies that can influence the direction of data-driven enterprises.
Rachamalla’s leadership in the realm of data security and analytics positions him as a key thought leader in shaping the future of AI-driven data governance.
Dilip Rachamalla’s work continues to inspire organizations globally, illustrating how integrating secure, AI-powered data architectures will be critical to unlocking the full potential of business analytics.
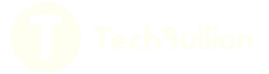