In today’s digital transformation era, financial institutions are rethinking how they safeguard transactions against increasingly sophisticated fraudulent activities. As the volume of digital transactions escalates, traditional security methods fall short, paving the way for advanced, AI-driven solutions that can operate at the speed and scale demanded by modern finance. Sudeep Annappa Shanubhog, a pioneering researcher in this field, has developed a framework that leverages machine learning and real-time analytics to redefine fraud prevention. His work illustrates how seamlessly integrating cutting-edge AI techniques with robust security protocols can elevate financial protection to unprecedented levels.
AI-Powered Innovation
At the core of this breakthrough is an innovative approach that harnesses the power of hybrid machine learning models. The system identifies known fraud patterns and emerging anomalies by combining supervised learning methods with unsupervised techniques. This multi-layered strategy enhances detection accuracy and minimizes false positives—a critical factor in maintaining smooth customer experiences. The fusion of diverse algorithmic approaches, including ensemble methods, has created a detection architecture that continuously evolves, adapting to the dynamic nature of financial transactions.
Real-Time Analytics Revolution
The system’s innovative design harnesses real-time processing capabilities to revolutionize fraud detection. By leveraging advanced stream processing techniques within a lambda architecture, it efficiently handles enormous data volumes with sub-100ms latency. This rapid processing allows for immediate transformation of raw transaction data into meaningful fraud indicators through dynamic feature engineering. Such agility ensures that any anomalous or suspicious activity is promptly identified and flagged, significantly reducing the window for fraudulent transactions to inflict damage. Unlike traditional batch-processing systems that suffer from delays, this real-time approach provides a proactive defense, enabling organizations to mitigate risks swiftly and maintain robust security protocols in a continuously evolving threat landscape. Financial institutions can safeguard assets in real-time with continuous monitoring and automated responses.
Securing Data with Advanced Protocols
Expanding on the multi-layered security and compliance framework, the system enforces strict zero-trust policies that continuously validate every access request and scrutinize data usage patterns. This rigorous approach detects anomalies and potential threats before they can escalate. Advanced encryption protocols safeguard sensitive financial data, while anonymization techniques protect user identities without compromising analytical depth. Continuous monitoring paired with AI-enhanced threat detection enables dynamic responses and real-time risk assessments, ensuring that security measures evolve alongside emerging cyber threats. This proactive, adaptive security posture reinforces system resilience and instills confidence in critical financial operations’ integrity, ensuring global uninterrupted operational excellence.
Scalable and Adaptable Systems
Another key innovation lies in the system’s scalable architecture, designed to handle high transaction volumes without compromising performance. The solution achieves both independent component scaling and optimal resource utilization by leveraging containerized microservices and distributed computing frameworks. The flexible API integration—built on a resilient service mesh architecture—ensures that various modules communicate seamlessly while maintaining stringent security controls. This modular design simplifies system maintenance and allows for rapid adaptation as transaction volumes and fraud tactics evolve.
Adaptive Learning and Future Prospects
Continuous learning is embedded at the heart of this innovative framework. The system employs online learning algorithms capable of updating models in real-time to accommodate emerging fraud patterns. Concept drift detection plays a crucial role here, automatically identifying shifts in transaction behavior and recalibrating the detection models accordingly. These adaptive capabilities are complemented by sophisticated risk-scoring methodologies that dynamically adjust model weights based on predictive performance. Looking ahead, emerging trends such as federated learning and graph-based analytics hold the potential to further enhance fraud detection, promising even more refined approaches to identifying complex fraud schemes.
In conclusion, Sudeep Annappa Shanubhog‘s innovative framework detailed above offers a glimpse into the future of fraud prevention, where real-time analytics and adaptive machine learning converge to create a secure financial ecosystem. This breakthrough approach addresses the immediate challenges posed by high-volume, digital transactions and lays the groundwork for continuous evolution in fraud detection strategies. By integrating advanced AI techniques with robust security measures, the system ensures financial institutions can stay one step ahead of increasingly sophisticated fraudulent activities. As digital transformation continues to reshape the financial landscape, innovations like these will safeguard the integrity of financial transactions and maintain trust in the digital economy.
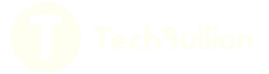